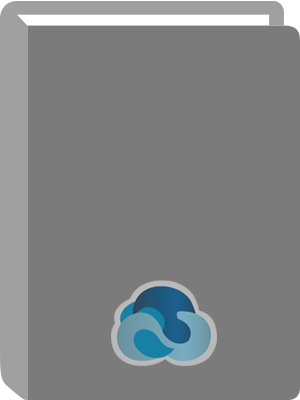
Statistical Modelling of Molecular Descriptors in QSAR/QSPR.
Title:
Statistical Modelling of Molecular Descriptors in QSAR/QSPR.
Author:
Emmert-Streib, Frank.
ISBN:
9783527645022
Personal Author:
Edition:
2nd ed.
Physical Description:
1 online resource (458 pages)
Series:
Quantitative and Network Biology (VCH) Ser.
Contents:
Statistical Modelling of Molecular Descriptors in QSAR/QSPR -- Contents -- Preface -- List of Contributors -- 1 Current Modeling Methods Used in QSAR/QSPR -- 1.1 Introduction -- 1.2 Modeling Methods -- 1.2.1 Methods for Regression Problems -- 1.2.1.1 Multiple Linear Regression -- 1.2.1.2 Partial Least Squares -- 1.2.1.3 Feedforward Backpropagation Neural Network -- 1.2.1.4 General Regression Neural Network -- 1.2.1.5 Gaussian Processes -- 1.2.2 Methods for Classification Problems -- 1.2.2.1 Logistic Regression -- 1.2.2.2 Linear Discriminant Analysis -- 1.2.2.3 Decision Tree and Random Forest -- 1.2.2.4 k-Nearest Neighbor -- 1.2.2.5 Probabilistic Neural Network -- 1.2.2.6 Support Vector Machine -- 1.3 Software for QSAR Development -- 1.3.1 Structure Drawing or File Conversion -- 1.3.2 3D Structure Generation -- 1.3.3 Descriptor Calculation -- 1.3.4 Modeling -- 1.3.5 General purpose -- 1.4 Conclusion -- References -- 2 Developing Best Practices for Descriptor-Based Property Prediction: Appropriate Matching of Datasets, Descriptors, Methods, and Expectations -- 2.1 Introduction -- 2.1.1 Posing the Question -- 2.1.2 Validating the Models -- 2.1.3 Interpreting the Models -- 2.2 Leveraging Experimental Data and Understanding their Limitations -- 2.3 Descriptors: The Lexicon of QSARs -- 2.3.1 Classical QSAR Descriptors and Uses -- 2.3.2 Experimentally Derived Descriptors -- 2.3.2.1 Biodescriptors -- 2.3.2.2 Descriptors from Spectroscopy/Spectrometry and Microscopy -- 2.3.3 0D, 1D and 2D Computational Descriptors -- 2.3.4 3D Descriptors and Beyond -- 2.3.5 Local Molecular Surface Property Descriptors -- 2.3.6 Quantum Chemical Descriptors -- 2.4 Machine Learning Methods: The Grammar of QSARs -- 2.4.1 Principal Component Analysis -- 2.4.2 Factor Analysis.
2.4.3 Multidimensional Scaling, Stochastic Proximity Embedding, and Other Nonlinear Dimensionality Reduction Methods -- 2.4.4 Clustering -- 2.4.5 Partial Least Squares (PLS) -- 2.4.6 k-Nearest Neighbors (kNN) -- 2.4.7 Neural Networks -- 2.4.8 Ensemble Models -- 2.4.9 Decision Trees and Random Forests -- 2.4.10 Kernel Methods -- 2.4.11 Ranking Methods -- 2.5 Defining Modeling Strategies: Putting It All Together -- 2.6 Conclusions -- References -- 3 Mold2 Molecular Descriptors for QSAR -- 3.1 Background -- 3.1.1 History of QSAR -- 3.1.2 Introduction to QSAR -- 3.1.3 Molecular Descriptors: Bridge for QSAR -- 3.1.3.1 Molecular Descriptors -- 3.1.3.2 Role of Molecular Descriptors -- 3.1.3.3 Types of Molecular Descriptors -- 3.1.3.4 Calculation of Molecular Descriptors (Software Packages) -- 3.2 Mold2 Molecular Descriptors -- 3.2.1 Description of Mold2 Descriptors -- 3.2.1.1 Topological Descriptors -- 3.2.1.2 Constitutional Descriptors -- 3.2.1.3 Information Content-based Descriptors -- 3.2.2 Calculation of Mold2 Descriptors -- 3.2.3 Evaluation of Mold2 Descriptors -- 3.2.3.1 Information Content by Shannon Entropy Analysis -- 3.2.3.2 Correlations between Descriptors -- 3.3 QSAR Using Mold2 Descriptors -- 3.3.1 Classification Models based on Mold2 Descriptors -- 3.3.2 Regression Models based on Mold2 Descriptors -- 3.4 Conclusion Remarks -- References -- 4 Multivariate Analysis of Molecular Descriptors -- 4.1 Introduction -- 4.2 2D Matrix-Based Descriptors -- 4.3 Graph-Theoretical Matrices -- 4.3.1 Vertex Weighting Schemes -- 4.4 Multivariate Similarity Analysis of Chemical Spaces -- 4.5 Analysis of Chemical Information of Descriptors from Graph-Theoretical Matrices -- 4.5.1 Data Sets -- 4.5.2 Comparison of Graph-Theoretical Matrices -- 4.5.2.1 Comparison of Weighted Graph-Theoretical Matrices -- 4.5.3 Comparison of Matrix Operators.
4.5.4 Comparison of Single Operators from Different Graph-Theoretical Matrices -- 4.6 Conclusions -- References -- 5 Partial-Order Ranking and Linear Modeling: Their Use in Predictive QSAR/QSPR Studies -- 5.1 Introduction -- 5.2 Linear QSAR Methodology, ERM, RM and GA -- 5.2.1 Replacement Method -- 5.2.2 Enhanced Replacement Method -- 5.2.3 Genetic Algorithm -- 5.2.4 Main Differences between MRM and RM -- 5.3 Principles of Ranking Methods -- 5.4 Selection of the Molecular Descriptors for Ranking -- 5.5 QSAR Based on Hasse Diagrams -- 5.6 Discussion -- 5.7 Conclusions -- References -- 6 Graph-Theoretical Descriptors for Branched Polymers -- 6.1 Introduction -- 6.2 Algebraic Graph Theory -- 6.3 Ideal Chain Models -- 6.4 Graph-Theoretical Approach to Chain Dynamics and Statistics -- 6.4.1 Radius of Gyration -- 6.4.2 Rouse Dynamics -- 6.4.3 Intrinsic Viscosity -- 6.4.4 Scattering Function -- 6.4.5 High Moments of Relaxation Time and Radius of Gyration -- 6.5 Applications -- 6.6 Final Remarks -- References -- 7 Structural-Similarity-Based Approaches for the Development of Clustering and QSPR/QSAR Models in Chemical Databases -- 7.1 Chemical Structural Similarity -- 7.1.1 Molecular Graph and Structural Similarity -- 7.1.2 Descriptor-Based Structural Similarity -- 7.1.3 Combining Structural Similarity Approaches -- 7.1.4 Approximate Structural Similarity -- 7.2 Clustering Models Based on Structural Similarity -- 7.2.1 Clustering of Chemical Databases -- 7.2.1.1 Pattern Representation of Chemicals Structures -- 7.2.1.2 Clustering of Chemical Databases -- 7.3 QSPR/QSAR Models Based on Structural Similarity -- 7.3.1 Dataset Selection -- 7.3.2 Dataset Representation -- 7.3.3 Fitting of the Dataset Representation -- 7.3.4 Building and Validation of the QSAR Model -- References.
8 Statistical Methods for Predicting Compound Recovery Rates for Ligand- Based Virtual Screening and Assessing the Probability of Activity -- 8.1 Introduction -- 8.2 Theory -- 8.2.1 Bayesian Approach to Virtual Screening -- 8.2.2 Predicting the Performance of Bayesian Screening -- 8.2.3 Practical Prediction of Compound Recall -- 8.2.4 Exemplary Results -- 8.3 Alternative Approaches to the Prediction of Compound Recall -- 8.4 Conclusions -- References -- 9 Molecular Descriptors and the Electronic Structure -- 9.1 Introduction -- 9.2 The Structure of Molecules -- 9.2.1 General Remarks -- 9.2.2 Structure Coding -- 9.2.3 Structural Features -- 9.2.4 Structure and Energy -- 9.3 The Electronic Structure -- 9.4 Dividing Molecules in Atoms and Bonds -- 9.4.1 Bonding in Molecules -- 9.4.2 Energy Partitioning -- 9.4.3 Energy and the Hückel Approach -- 9.4.4 Energy Components of Atoms and Bonds -- 9.4.5 Perturbation Treatment of the Electronic Structure -- 9.4.6 Thermodynamic Equilibrium -- 9.4.7 Model of ''Atom in Molecules'' -- 9.5 Structure and Dynamics -- 9.5.1 Molecular Flexibility -- 9.5.2 Molecular Dynamics Simulation -- 9.5.3 Conformational Space -- 9.6 Structure and Properties -- 9.6.1 Structure Property Relationships -- 9.6.2 Type of Molecular Properties -- 9.6.3 Molecular Commonality and Similarity -- 9.6.4 Multilinear Regression -- 9.6.5 Selection of Molecular Descriptors -- 9.7 Modeling of Physicochemical Properties of the Isomers of Hexane -- 9.8 Modeling of the Proton Affinity -- 9.8.1 Proton Affinity of Pyridines -- 9.8.1.1 Data and Mechanism -- 9.8.1.2 Model I -- 9.8.1.3 Model II -- 9.8.1.4 Model III -- 9.8.1.5 Model IV -- 9.8.1.6 Model V -- 9.8.1.7 Model VI -- 9.8.2 Basicity of N-Heterocyclic Aromatics -- 9.9 Molecular Surface Properties -- 9.10 Conclusions -- References -- 10 New Types of Descriptors and Models in QSAR/QSPR.
10.1 Introduction -- 10.2 Local Properties -- 10.2.1 Molecular Electrostatic Potential -- 10.2.2 Electron Density -- 10.2.3 Local Polarizability -- 10.2.4 Local Ionization Energy and Local Electron Affinity -- 10.3 Descriptors Derived from Local Properties -- 10.3.1 PEST Methodology -- 10.4 MEP as Descriptor for Hydrogen-Bonding Strengths -- 10.5 ParaSurf (Politzer-Murray) Descriptors -- 10.6 4D: Conformational-Ensemble-based Descriptors -- 10.7 Proper Validation/Generation of QSA(P)R Models -- 10.8 Conclusions -- References -- 11 Consensus Models of Activity Landscapes -- 11.1 Introduction -- 11.2 Characterization of the Activity Landscape -- 11.3 Consensus Models of Activity Landscape -- 11.3.1 Chemical Space and Molecular Representation -- 11.3.2 Activity Landscape with Multiple Representations -- 11.4 Conclusions and Future Perspectives -- References -- 12 Reverse Engineering Chemical Reaction Networks from Time Series Data -- 12.1 Introduction -- 12.2 Problem Definition -- 12.3 Reconstruction of Elementary Reaction Networks from Data by Network Search -- 12.3.1 Network Search as a Nonlinear Integer Programming Problem -- 12.3.2 Estimation of the Rate Coefficients for Trial Reaction Networks -- 12.4 Formulation of the Objective Function for Network Search -- 12.4.1 Physical/Chemical Information Available 336 -- 12.4.2 No physical/Chemical Information Available -- 12.5 Differential Evolution for Searching the Space of Reaction Networks -- 12.5.1 Basic DE Optimization Method -- 12.5.2 Self-Adaptive DE with Integer Variables -- 12.6 Network Identification Case Studies -- 12.6.1 Estimation of Time Derivatives -- 12.6.2 DE Settings -- 12.6.3 Model Selection Methodology -- 12.6.4 Results -- 12.7 Conclusions -- References.
13 Reduction of Dimensionality, Order, and Classification in Spaces of Theoretical Descriptions of Molecules: An Approach Based on Metrics, Pattern Recognition Techniques, and Graph Theoretic Considerations.
Abstract:
This handbook and ready reference presents a combination of statistical, information-theoretic, and data analysis methods to meet the challenge of designing empirical models involving molecular descriptors within bioinformatics. The topics range from investigating information processing in chemical and biological networks to studying statistical and information-theoretic techniques for analyzing chemical structures to employing data analysis and machine learning techniques for QSAR/QSPR. The high-profile international author and editor team ensures excellent coverage of the topic, making this a must-have for everyone working in chemoinformatics and structure-oriented drug design.
Local Note:
Electronic reproduction. Ann Arbor, Michigan : ProQuest Ebook Central, 2017. Available via World Wide Web. Access may be limited to ProQuest Ebook Central affiliated libraries.
Genre:
Electronic Access:
Click to View