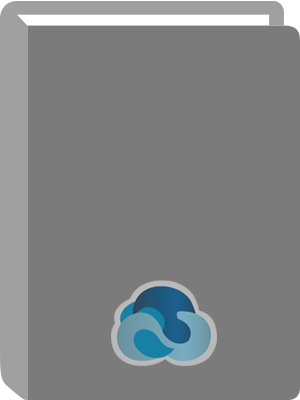
Biomedical Informatics in Translational Research.
Title:
Biomedical Informatics in Translational Research.
Author:
Hu, Hai.
ISBN:
9781596930391
Personal Author:
Physical Description:
1 online resource (278 pages)
Contents:
Biomedical Informatics in Translational Research -- Contents -- Preface -- Chapter 1 Biomedical Informatics in TranslationalResearch -- 1.1 Evolution of Terminology -- 1.1.1 Translational Research -- 1.1.2 Systems Biology -- 1.1.3 Personalized Medicine -- References -- Chapter 2 The Clinical Perspective -- 2.1 Introduction -- 2.2 Ethics in Clinical Research -- 2.3 Regulatory Policies for Protecting a Research Subject's Privacy -- 2.4 Informed Consent -- 2.5 Collecting Clinical Data: Developing and Administering Survey Instruments -- 2.6 Issues Important to Biomedical Informatics -- 2.6.1 Data Tracking and Centralization -- 2.6.2 Deidentifying Data -- 2.6.3 Quality Assurance -- 2.6.4 Data Transfer from the Health Care Clinic to the Research Setting -- 2.7 Standard Operating Procedures -- 2.8 Developing and Implementing a Research Protocol -- 2.8.1 Developing a Research Protocol -- 2.8.2 Implementing the Research Protocol -- 2.9 Summary -- References -- Chapter 3 Tissue Banking: Collection, Processing, and Pathologic Characterization of Biospecimens for Research -- 3.1 Introduction -- 3.1.1 A Biorepository's Mandate -- 3.1.2 Overview of Current Tissue Banking Practices -- 3.2 Consenting and Clinical Data Acquisition -- 3.3 Blood Collection, Processing, and Storage -- 3.4 Tissue Collection, Processing, Archiving, and Annotation -- 3.4.1 Tissue Collection -- 3.4.2 Tissue Processing -- 3.4.3 Tissue Archiving and Storage -- 3.4.4 Pathologic Characterization of Tissue Samples -- 3.5 Conclusion -- References -- Chapter 4 Biological Perspective -- 4.1 Background for "Omics" Technologies -- 4.2 Basic Biology and Definitions -- 4.2.1 A Historical Perspective -- 4.2.2 Biological Processes -- 4.2.3 Some Definitions -- 4.3 Very Basic Biochemistry -- 4.3.1 DNA -- 4.3.2 RNA -- 4.3.3 Proteins -- 4.4 Summary -- References -- Chapter 5 Genomics Studies.
5.1 Introduction -- 5.2 Genomic Technologies Used for DNA Analysis -- 5.2.1 DNA Sequencing -- 5.2.1.2 Biomedical Informatics Requirements -- 5.2.1.3 Future Directions -- 5.2.2 Genotyping -- 5.2.2.1 Array Technologies -- 5.2.2.2 Technological Assessment of Genotyping -- 5.2.2.3 Affymetrix Genotyping SNP Assay Workflow -- 5.2.2.4 QA/SOP Issues -- 5.2.2.5 Biomedical Informatics Requirements -- 5.2.2.6 Future Directions -- 5.2.3 Array-Based Comparative Genomic Hybridization -- 5.2.3.1 Technological Assessment of Chromosomal Rearrangements -- 5.2.3.2 Example Platform -- 5.2.3.3 QA/SOP Issues -- 5.2.3.4 Biomedical Informatics Requirements -- 5.2.3.5 Oligo-Based aCGH Platform -- 5.3 Genomic Technology Used for RNA Analysis -- 5.3.1 Real-Time PCR -- 5.3.1.1 Data Analysis Methods -- 5.3.1.2 Biomedical Informatics Requirements -- 5.3.1.3 Future Directions -- 5.3.2 Microarrays -- 5.3.2.1 Array Technologies -- 5.3.2.2 Example Platform -- 5.3.2.3 QA/SOP Issues -- 5.3.2.4 MIAME Checklist and Platform Comparison -- 5.3.2.5 Data Analysis Issues -- 5.3.2.6 Biomedical Informatics Requirements -- 5.3.2.7 Future Directions -- 5.3.3 Chips for Alternative Splicing Analysis (GeneChip Exon) -- 5.3.3.1 Array Technology -- 5.3.3.2 Biomedical Informatics Requirements -- 5.3.3.3 Future Directions -- 5.4 Translational Research Case Studies -- 5.4.1 Case 1 -- 5.4.2 Case 2 -- 5.5 Summary -- References -- Chapter 6 Proteomics -- 6.1 Introduction -- 6.2 Clinical Specimens -- 6.2.1 Body Fluids -- 6.2.2 Tissue -- 6.2.1.1 Blood -- 6.2.1.2 Urine -- 6.2.1.3 Cerebrospinal Fluid -- 6.3 Proteomics Technologies -- 6.3.1 Two-Dimensional Gel Electrophoresis -- 6.3.2 MALDI-TOF -- 6.3.3 Liquid Chromatography Mass Spectrometry -- 6.3.3.1 Shotgun Proteomics -- 6.3.3.2 Characterization of Posttranslational Modification of Proteins Using the Shotgun Approach -- 6.3.4 Protein Arrays.
6.4 Analysis of Proteomics Data -- 6.4.1 2D DIGE Data Analysis -- 6.4.2 SELDI-TOF/MALDI-TOF Data Analysis -- 6.4.3 Shotgun Proteomics Data Analysis -- 6.5 Summary -- References -- Chapter 7 Data Tracking Systems -- 7.1 Introduction -- 7.1.1 Definition of a Data Tracking System -- 7.1.2 Why Use a Data Tracking System? -- 7.2 Overview of Data Tracking Systems -- 7.2.1 Historical Review -- 7.2.2 Available Resources -- 7.2.3 Data Tracking Systems in the Life Sciences -- 7.2.3.1 Clinical Data Collection -- 7.2.3.2 Tissue Processing and Banking -- 7.2.3.3 Data Tracking for Genomics and Proteomics Studies -- 7.3 Major Requirements of a Data Tracking System for Biomedical Informatics Research -- 7.3.1 General Requirements -- 7.3.2 Front-End Requirements -- 7.3.3 Back-End Requirements -- 7.3.4 Field-Specific Requirements -- 7.3.4.1 Requirements for Clinical Data Tracking -- 7.3.4.2 Requirements for Tissue Banking and Specimen Preparation -- 7.3.4.3 Requirements for Genomics Experiments -- 7.3.4.4 Requirements for Proteomics Experiments -- 7.3.5 Additional Points -- 7.4 Ways to Establish a Data Tracking System -- 7.4.1 Buy a System Off the Shelf -- 7.4.2 Develop a System -- 7.4.3 Pursue a Hybrid Approach -- 7.5 Deployment Challenges and Other Notes -- 7.5.1 Resistance from End Users -- 7.5.2 Training -- 7.5.3 Mismatches Between System Features and Real Needs -- 7.5.4 Protocol Changes and Other Evolutions -- 7.5.5 Data Tracking System as a Data Source -- 7.6 Summary -- References -- Chapter 8 Data Centralization -- 8.1 An Overview of Data Centralization -- 8.2 Types of Data in Question -- 8.2.1 in-house Patient-Centric Clinical, Genomic, and Proteomic Data -- 8.2.2 Publicly Available Annotation and Experimental Data -- 8.2.2.1 Popular Public Databases -- 8.2.2.2 Public Databases of Special Values.
8.2.2.3 Considerations and Concerns About Using or Integrating Public Data -- 8.2.3 Data Format Standards -- 8.3 DW Development for Integrative Biomedical Informatics Research -- 8.3.1 Selection of the Developing Partner-Experiences in the Field -- 8.3.2 DW Requirements -- 8.3.3 Data Source Selection -- 8.3.4 Hardware and the Database Management Systems Selection -- 8.3.5 DW Structural Models-Integrated, Federated, or Hybrid -- 8.3.6 Data Models: Dimensional Models, Data Marts, and Normalization Levels -- 8.3.7 Data Models: EAV, Entity-Relationship, and Object-Oriented Modules -- 8.3.8 Data Models: Handling of the Temporal Information -- 8.3.9 Data Extraction, Cleansing, Transformation, and Loading -- 8.3.10 Tuning and QA -- 8.3.11 Changes-The Dynamic Nature -- 8.4 Use of the DW -- 8.5 Example Case -- 8.6 Summary -- References -- Chapter 9 Data Analysis -- 9.1 The Nature and Diversity of Research Data in Translational Medicine -- 9.1.1 Where Data Reside -- 9.1.2 Operational Versus Analytical Data Systems -- 9.1.3 Data Warehouses -- 9.1.4 Data Preprocessing -- 9.1.4.1 Missing Value Handling -- 9.1.4.2 Outlier Removal (Clipping) -- 9.1.4.3 Binning -- 9.1.4.4 Normalization -- 9.2 Data Analysis Methods and Techniques -- 9.2.3 Predictive Modeling -- 9.2.3.1 Feature Selection -- 9.2.3.2 Regression -- 9.2.3.3 Classification -- 9.2.4 Clustering -- 9.2.4.1 Self-Organizing Maps -- 9.2.4.2 K-Means -- 9.2.4.3 Hierarchical -- 9.2.5 Evaluation and Validation Methodologies -- 9.2.5.1 Type I and Type II Errors -- 9.2.5.2 Sensitivity Versus Specificity -- 9.2.5.3 ROC Graph -- 9.2.5.4 Cross Validation -- 9.2.1 Generalized Forms of Analysis -- 9.2.1.1 Significance Testing -- 9.2.1.2 Predictive Modeling -- 9.2.1.3 Clustering -- 9.2.2 Significance Testing -- 9.2.2.1 Pearson's Chi-Square Test -- 9.2.2.2 Student's t-Test -- 9.2.2.3 ANOVA.
9.2.2.4 Wilcoxon Signed-Rank Test -- 9.3 Analysis of High-Throughput Genomic and Proteomic Data -- 9.3.1 Genomic Data -- 9.3.1.1 Different Platforms Available -- 9.3.1.2 Quality Control -- 9.3.1.3 Image Analysis -- 9.3.1.4 Data Normalization and Transformation -- 9.3.1.5 Statistical Analysis -- 9.3.1.6 High-Level Analysis: Profiling or Pattern Recognition -- 9.3.2 Proteomic Data -- 9.3.3 Functional Determination -- 9.3.3.1 Gene Ontology -- 9.3.3.2 Pathway Analysis -- 9.4 Analysis of Clinical Data -- 9.5 Analysis of Textual Data -- 9.5.1 Data Sources -- 9.5.2 Biological Entity -- 9.5.3 Mining Relations Between Named Entities -- 9.6 Integrative Analysis and Application Examples -- 9.7 Data Analysis Tools and Resources -- 9.8 Summary -- References -- Chapter 10 Research and Application: Examples -- 10.1 Introduction -- 10.2 deCODE Genetics -- 10.2.1 Data Repository Development and Data Centralization -- 10.2.1.1 Clinical Data Collection: Patient Consent and Deidentification -- 10.2.2 Genomic Studies -- 10.2.2.1 Linkage Analysis -- 10.2.2.2 Association Studies -- 10.2.2.3 Limitations -- 10.2.2.4 Analysis Tool -- 10.2.3 Application -- 10.2.3.1 Drug Development Based on Identification of Genotype for Myocardial Infarction Risk -- 10.2.3.2 Development of a Diagnostic to Detect a Diabetes Risk Genotype -- 10.3 Windber Research Institute -- 10.3.1 Clinical Data Collection and Storage -- 10.3.1.1 The Clinical Breast Care Project -- 10.3.1.2 Integrative Cardiac and Metabolic Health Program -- 10.3.2 Data Tracking -- 10.3.3 Data Centralization -- 10.3.4 Genomic and Proteomic Studies -- 10.3.4.1 Allelic Imbalance to Characterize Genomic Instability -- 10.3.4.2 Proteomic Analysis of Cardiovascular Risk Factor Modification -- 10.3.5 Data Analysis, Data Mining, and Data Visualization -- 10.3.5.1 Knowledge Development Environment.
10.3.5.2 Pathology Co-Occurrence Analysis.
Abstract:
This groundbreaking resource on biomedical informatics gives you step-by-step insight into innovative techniques for integrating and federating data from clinical and high-throughput molecular study platforms as well as from the public domain. It details how to apply computational and statistical technologies to clinical, genomic, and proteomic studies to enhance data collection, tracking, storage, visualization, analysis, and knowledge discovery processes, and to translate knowledge from "bench to bedside" and "bedside to bench" with never-before efficiency.Filling the need for informatics applications that bridge the clinical-basic domains and facilitate the bi-directional flow of research, this definitive volume offers a systems-oriented approach to the subject that complements the traditional bottom-up approach of systems biology. You get clear insight into how to conduct biomedical informatics research at both the clinical and molecular levels, with detailed guidelines on study design, IRB protocol development, questionnaire design, specimen collection, and other procedures and applications. The book explains the latest data integration and federation approaches, and points the way to potential new data analysis and mining methodologies for tackling problems that cannot be readily resolved using current technologies. Complete with in-depth case examples demonstrating how to develop tools for specific biomedical informatics tasks, this pioneering work will prove invaluable to your efforts in managing clinical and high-throughput data and making the most of targeted basic research.
Local Note:
Electronic reproduction. Ann Arbor, Michigan : ProQuest Ebook Central, 2017. Available via World Wide Web. Access may be limited to ProQuest Ebook Central affiliated libraries.
Genre:
Added Author:
Electronic Access:
Click to View