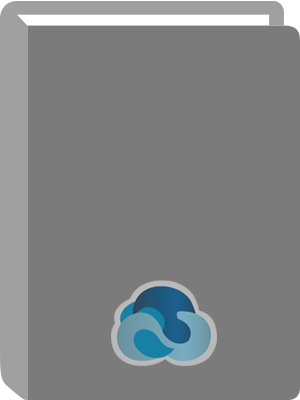
Multi-frame super-resolution without priors
Title:
Multi-frame super-resolution without priors
Author:
Gülmez, Veli, author.
Personal Author:
Physical Description:
ix, 62 leaves: color illustrations, charts;+ 1 computer laser optical disc.
Abstract:
There are mainly two types of super-resolution methods: traditional methods and deep learning methods. While traditional methods define closed-form expressions with assumptions, deep learning methods rely on priors learned from data sets. However, both of them have disadvantages such as being too simple and having strong trust in priors. We focus on how to generate a high-resolution image using low-resolution images without priors by utilizing spatial hash encoding. We propose a grid-based super-resolution model using spatial hash encoding to map coordinate information into higher dimensional space. Our aim is to eliminate long training times and not rely on priors from data sets that are not able to cover all real-world scenarios. Therefore, our proposed model is able to do task-specific super-resolution without priors and eliminate potential hallucination effects caused by wrong priors.
Added Author:
Added Corporate Author:
Added Uniform Title:
Thesis (Master)--İzmir Institute of Technology:Computer Engineering.
İzmir Institute of Technology: Computer Engineering--Thesis (Master).
Electronic Access:
Access to Electronic Versiyon.