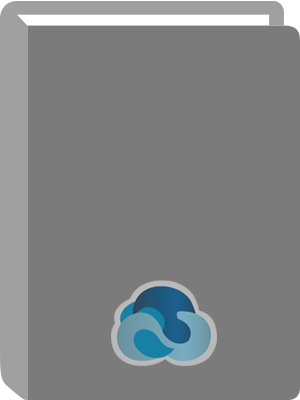
Blind Identification and Separation of Complex-valued Signals.
Title:
Blind Identification and Separation of Complex-valued Signals.
Author:
Moreau, Eric.
ISBN:
9781118579732
Personal Author:
Edition:
1st ed.
Physical Description:
1 online resource (108 pages)
Series:
FOCUS Series
Contents:
Cover -- Title Page -- Contents -- Preface -- Acknowledgments -- Chapter 1. Mathematical Preliminaries -- 1.1. Introduction -- 1.2. Linear mixing model -- 1.3. Problem definition -- 1.4. Statistics -- 1.4.1. Statistics of random variables and random vectors -- 1.4.2. Differential entropy of complex random vectors -- 1.4.3. Statistics of random processes -- 1.4.4. Complex matrix decompositions -- 1.5. Optimization: Wirtinger calculus -- 1.5.1. Scalar case -- 1.5.2. Vector case -- 1.5.3. Matrix case -- 1.5.4. Summary -- Chapter 2. Estimation by Joint Diagonalization -- 2.1. Introduction -- 2.2. Normalization, dimension reduction and whitening -- 2.2.1. Dimension reduction -- 2.2.2. Whitening -- 2.3. Exact joint diagonalization of two matrices -- 2.3.1. After the whitening stage -- 2.3.2. Without explicit whitening -- 2.4. Unitary approximate joint diagonalization -- 2.4.1. Considered problem -- 2.4.2. The 2 × 2 Hermitian case -- 2.4.3. The 2 × 2 complex symmetric case -- 2.5. General approximate joint diagonalization -- 2.5.1. Considered problem -- 2.5.2. A relative gradient algorithm -- 2.6. Summary -- Chapter 3. Maximum Likelihood Ica -- 3.1. Introduction -- 3.2. Cost function choice -- 3.2.1. Mutual information and mutual information rate minimization -- 3.2.2. Maximum likelihood -- 3.2.3. Identifiability of the complex ICA model -- 3.3. Algorithms -- 3.3.1. ML ICA: unconstrained W -- 3.3.2. Complex maximization of non-Gaussianity: ML ICA with unitary W -- 3.3.3. Density matching -- 3.3.4. A flexible complex ICA algorithm: Entropy bound minimization -- 3.4. Summary -- Bibliography -- Index.
Abstract:
Blind identification consists of estimating a multi-dimensional system only through the use of its output, and source separation, the blind estimation of the inverse of the system. Estimation is generally carried out using different statistics of the output. The authors of this book consider the blind identification and source separation problem in the complex-domain, where the available statistical properties are richer and include non-circularity of the sources - underlying components. They define identifiability conditions and present state-of-the-art algorithms that are based on algebraic methods as well as iterative algorithms based on maximum likelihood theory. Contents 1. Mathematical Preliminaries. 2. Estimation by Joint Diagonalization. 3. Maximum Likelihood ICA. About the Authors Eric Moreau is Professor of Electrical Engineering at the University of Toulon, France. His research interests concern statistical signal processing, high order statistics and matrix/tensor decompositions with applications to data analysis, telecommunications and radar. Tülay Adali is Professor of Electrical Engineering and Director of the Machine Learning for Signal Processing Laboratory at the University of Maryland, Baltimore County, USA. Her research interests concern statistical and adaptive signal processing, with an emphasis on nonlinear and complex-valued signal processing, and applications in biomedical data analysis and communications. Blind identification consists of estimating a multidimensional system through the use of only its output. Source separation is concerned with the blind estimation of the inverse of the system. The estimation is generally performed by using different statistics of the outputs. The authors consider the blind estimation of a multiple input/multiple output (MIMO) system that mixes a number of underlying signals of
interest called sources. They also consider the case of direct estimation of the inverse system for the purpose of source separation. They then describe the estimation theory associated with the identifiability conditions and dedicated algebraic algorithms. The algorithms depend critically on (statistical and/or time frequency) properties of complex sources that will be precisely described.
Local Note:
Electronic reproduction. Ann Arbor, Michigan : ProQuest Ebook Central, 2017. Available via World Wide Web. Access may be limited to ProQuest Ebook Central affiliated libraries.
Subject Term:
Genre:
Added Author:
Electronic Access:
Click to View