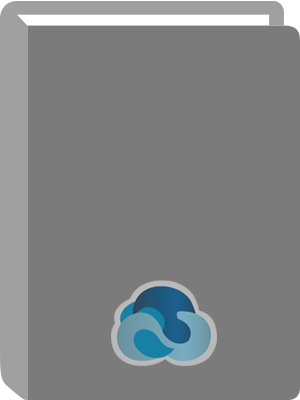
Panel Data Analysis using EViews.
Title:
Panel Data Analysis using EViews.
Author:
Agung, I. Gusti Ngurah.
ISBN:
9781118715574
Personal Author:
Edition:
1st ed.
Physical Description:
1 online resource (541 pages)
Contents:
Panel Data Analysis Using EViews -- Contents -- Preface -- About the Author -- Part One: Panel Data as a Multivariate Time Series by States -- 1 Data Analysis Based on a Single Time Series by States -- 1.1 Introduction -- 1.2 Multivariate Growth Models -- 1.2.1 Continuous Growth Models -- 1.2.2 Discontinuous Growth Models -- 1.3 Alternative Multivariate Growth Models -- 1.3.1 A Generalization of MAR(p)_GM -- 1.3.2 Multivariate Lagged Variables Growth Models -- 1.3.3 Multivariate Lagged-Variable Autoregressive Growth Models -- 1.3.4 Bounded MLVAR(p -- q)_GM -- 1.3.5 Special Notes -- 1.4 Various Models Based on Correlated States -- 1.4.1 Seemingly Causal Models with Trend -- 1.4.2 The Application of the Object "VAR" -- 1.4.3 The Application of the Instrumental Variables Models -- 1.5 Seemingly Causal Models with Time-Related Effects -- 1.5.1 SCM Based on the Path Diagram in Figure 1.10(a) -- 1.5.2 SCM Based on the Path Diagram in Figure 1.10(b) -- 1.6 The Application of the Object POOL -- 1.6.1 What is a Fixed-Effect Model? -- 1.6.2 What is a Random Effect Model? -- 1.6.3 Special Notes -- 1.7 Growth Models of Sample Statistics -- 1.8 Special Notes on Time-State Observations -- 1.9 Growth Models with an Environmental Variable -- 1.9.1 The Simplest Possible Model -- 1.9.2 The Application of VAR and VEC Models -- 1.9.3 Application of ARCH Model -- 1.9.4 The Application of Instrumental Variables Models -- 1.10 Models with an Environmental Multivariate -- 1.10.1 Bivariate Correlation and Simple Linear Regressions -- 1.10.2 Simple Models with an Environmental Multivariate -- 1.10.3 The VAR Models -- 1.11 Special Piece-Wise Models -- 1.11.1 The Application of Growth Models -- 1.11.2 Equality Tests by Classifications -- 2 Data Analysis Based on Bivariate Time Series by States -- 2.1 Introduction -- 2.2 Models Based on Independent States.
2.2.1 MAR(p) Growth Model with an Exogenous Variable -- 2.2.2 A General MAR(p) Model with an Exogenous Variable -- 2.3 Time-Series Models Based on Two Correlated States -- 2.3.1 Analysis using the Object System -- 2.3.2 Two-SLS Instrumental Variables Models -- 2.3.3 Three-SLS Instrumental Variables Models -- 2.3.4 Analysis using the Object "VAR" -- 2.4 Time-Series Models Based on Multiple Correlated States -- 2.4.1 Extension of the Path Diagram in Figure 2.6 -- 2.4.2 SCMs as VAR Models -- 2.5 Time-Series Models with an Environmental Variable Zt, Based on Independent States -- 2.5.1 The Simplest Possible Model -- 2.5.2 Interaction Models Based on Two Independent States -- 2.6 Models Based on Correlated States -- 2.6.1 MLV(1) Interaction Model with Trend -- 2.6.2 Simultaneous SCMs with Trend -- 2.7 Piece-Wise Time-Series Models -- 3 Data Analysis Based on Multivariate Time Series by States -- 3.1 Introduction -- 3.2 Models Based on (X_i,Y_i,Z_i) for Independent States -- 3.2.1 MLVAR(p -- q) Model with Trend Based on (X_i,Y_i,Z_i) -- 3.3 Models Based on (X_i, Y_i,Z_i) for Correlated States -- 3.3.1 MLV(1) Interaction Model with Trend -- 3.3.2 MLV(1) Interaction Model with Time-Related Effects -- 3.4 Simultaneous SCMs with Trend -- 3.4.1 The Basic Simultaneous SCMs with Trend -- 3.4.2 Alternative Time Series Models -- 3.5 Models Based on (X1_i,X2_i,X3_i, Y1_i,Y2_i) for Independent States -- 3.5.1 Lagged Endogenous Variables: First Autoregressive Model with Exogenous Variables and Trend -- 3.5.2 A Mixed Lagged Variables First Autoregressive Model with Trend -- 3.5.3 Lagged Endogenous Variables: First Autoregressive Model with Exogenous Variables and Time-Related Effects -- 3.5.4 Various Interaction Models -- 3.6 Models Based on (X_i,Y_i) for Correlated States -- 3.6.1 Additive Models -- 3.6.2 Interaction Models.
3.6.3 Alternative Models for Two Correlated States -- 3.7 Discontinuous Time-Series Models -- 3.8 Additional Examples for Correlated States -- 3.9 Special Notes and Comments -- 3.9.1 Extended Models -- 3.9.2 Not-Recommended Models -- 3.9.3 Problems with Data Analysis -- 4 Applications of Seemingly Causal Models -- 4.1 Introduction -- 4.1.1 Deleting Time t from Models -- 4.1.2 Replacing Time t with an Environmental Variable -- 4.2 SCMs Based on a Single Time Series Y_it -- 4.3 SCMs Based on Bivariate Time Series (X_it,Y_it) -- 4.3.1 Additive SCM -- 4.3.2 ATwo-Way Interaction Standard SCM -- 4.3.3 AThree-Way Interaction Standard SCM -- 4.4 SCMs Based on a Trivariate (X1_i,X2_i,Y1_i) -- 4.4.1 Simple SCMs for Two Correlated States -- 4.4.2 Various Time-Series Models -- 4.5 SCMs Based on a Trivariate (X_it,Y1_it,Y2_it) -- 4.6 SCMs Based on Multivariate Endogenous and Exogenous Variables -- 4.6.1 Simple Additive SCM -- 4.6.2 A Two-Way Interaction SCM -- 4.6.3 A Three-Way Interaction SCM -- 4.6.4 Special Notes and Comments -- 4.6.5 Various Alternative SCMs -- 4.7 Fixed- and Random Effects Models -- 4.7.1 A Fixed-Effect Model and a MANCOVA Model -- 4.7.2 A Random Effect Model and a Single Regression -- 4.8 Models with Cross-Section Specific Coefficients -- 4.8.1 MAR(p) Model with Cross-Section Specific Coefficients -- 4.8.2 Advanced PLS Estimation Methods -- 4.8.3 Instrumental Variables Model -- 4.8.4 Special Notes and Comments -- 4.9 Cases in Industry -- 4.9.1 Dummy Variables Models -- Part Two: Pool Panel Data Analysis -- 5 Evaluation Analysis -- 5.1 Introduction -- 5.2 Preliminary Evaluation Analysis -- 5.3 The Application of the Object "Descriptive Statistics and Tests" -- 5.3.1 Analysis Using the Option "Stats by Classification. . ." -- 5.3.2 Analysis Using the Option "Equality Tests by Classification. . .".
5.3.3 Analysis Using the Option "N-Way Tabulation. . ." -- 5.4 Analysis Based on Ordinal Problem Indicators -- 5.5 Multiple Association between Categorical Variables -- 5.5.1 Applications of N-Way Tabulation -- 5.5.2 Application of Kendall's Tau -- 6 General Choice Models -- 6.1 Introduction -- 6.2 Multi-Factorial Binary Choice Models -- 6.2.1 One-Way Binary Choice Model -- 6.2.2 Two-Way Binary Choice Model -- 6.2.3 Multi-Factorial Binary Choice Model -- 6.3 Binary Logit Model of Yit on a Numerical Variable Xit -- 6.3.1 A Comparative Study -- 6.3.2 General Binary Logit Model with a Single Numerical Variable X -- 6.3.3 Special Notes and Comments -- 6.4 Binary Logit Model of a Zero-One Indicator Yit on (X1it,X2it) -- 6.4.1 The Simplest Possible Function -- 6.4.2 Functions for Binary Logit Translog Models -- 6.5 Binary Choice Model of a Zero-One Indicator Yit on (X1it,X2it,X3it) -- 6.5.1 The Linear Effect of X3 on Y Depends on X1 and X2 -- 6.5.2 Alternative Effects of X3 on Y Depends on X1 and X2 -- 6.6 Binary Choice Model of a Zero-One Indicator Yit on (X1it,. . ., Xhit,. . .) -- 6.7 Special Notes and Comments -- 7 Advanced General Choice Models -- 7.1 Introduction -- 7.2 Categorical Data Analyses -- 7.2.1 Multi-Factorial Binary Choice Models -- 7.2.2 Ordered Choice Models -- 7.2.3 Not-Recommended Models -- 7.2.4 Application of N-Way Tabulation -- 7.2.5 Applications of Ordinal-Ordinal Association -- 7.3 Multi-Factorial Choice Models with a Numerical Independent Variable -- 7.3.1 Nonparametric Estimation Methods -- 7.3.2 Parametric Estimation Methods -- 7.3.3 Polynomial-Effect BCM with Three Numerical Exogenous Variables -- 7.3.4 A Study of Li, et al. (2010) -- 7.3.5 A Study of Hameed, et al. (2010) -- 7.3.6 A Study of Francis and Martin (2010) -- 8 Univariate General Linear Models -- 8.1 Introduction -- 8.2 ANOVA and Quantile Models.
8.3 Continuous Linear-Effect Models -- 8.3.1 Bivariate Correlation Analysis -- 8.3.2 STEPLS Regressions -- 8.4 Piece-Wise Autoregressive Linear Models by Time Points -- 8.4.1 The Simplest Linear-Effect Models Based on (Xit,Yit) by Time Points -- 8.4.2 General Linear-Effect Model Based on (Xit,Yit) by Time Points -- 8.4.3 Linear-Effect Model Based on (X1it, X2it,Yit) by Time Points -- 8.4.4 Linear Models Based on (X1it, X2it, X3it,Yit) by Time Points -- 8.5 ANCOVA Models -- 9 Fixed-Effects Models and Alternatives -- 9.1 Introduction -- 9.2 Cross-Section Fixed-Effects Models -- 9.2.1 Individual Fixed-Effects Models -- 9.2.2 Group Fixed-Effects Models -- 9.3 Time-Fixed-Effects Models -- 9.4 Two-Way Fixed-Effects Models -- 9.4.1 Two-Way Fixed-Effects Models -- 9.4.2 Interaction FEMs -- 9.5 Extended Fixed-Effects Models -- 9.5.1 Least Square FEMs -- 9.5.2 Alternative FEMs -- 9.6 Selected Fixed-Effects Models from the Journal of Finance, 2011 -- 9.6.1 Additive FEM Applied by Hendershott, et al. (2011) -- 9.6.2 FEM Applied by Engelberg and Parsons (2011) -- 9.6.3 Special FEMs Applied by Benmelech and Bergman (2011) -- 9.7 Heterogeneous Regression Models -- 9.7.1 Heterogeneous Regressions by Individuals -- 9.7.2 Heterogeneous Classical Growth Models by Individuals or Groups -- 9.7.3 Piece-Wise Heterogeneous Regressions -- 9.7.4 Heterogeneous Regressions with Trend by Individuals or Groups -- 9.7.5 Heterogeneous Regressions with Time-Related Effects by Individuals or Groups -- 10 Special Notes on Selected Problems -- 10.1 Introduction -- 10.2 Problems with Dummy Variables -- 10.2.1 A Dummy of the Return Rate Rit -- 10.2.2 Other Types of Dummy Variables -- 10.3 Problems with the Numerical Variable Rit -- 10.3.1 Problem with Outliers -- 10.3.2 Problem with the Models of Rit with its Dummy DRit -- 10.4 Problems with the First Difference Variable.
10.5 Problems with Ratio Variables.
Abstract:
A comprehensive and accessible guide to panel data analysis using EViews software This book explores the use of EViews software in creating panel data analysis using appropriate empirical models and real datasets. Guidance is given on developing alternative descriptive statistical summaries for evaluation and providing policy analysis based on pool panel data. Various alternative models based on panel data are explored, including univariate general linear models, fixed effect models and causal models, and guidance on the advantages and disadvantages of each one is given. Panel Data Analysis using EViews: Provides step-by-step guidance on how to apply EViews software to panel data analysis using appropriate empirical models and real datasets. Examines a variety of panel data models along with the author's own empirical findings, demonstrating the advantages and limitations of each model. Presents growth models, time-related effects models, and polynomial models, in addition to the models which are commonly applied for panel data. Includes more than 250 examples divided into three groups of models (stacked, unstacked, and structured panel data), together with notes and comments. Provides guidance on which models not to use in a given scenario, along with advice on viable alternatives. Explores recent new developments in panel data analysis An essential tool for advanced undergraduate or graduate students and applied researchers in finance, econometrics and population studies. Statisticians and data analysts involved with data collected over long time periods will also find this book a useful resource.
Local Note:
Electronic reproduction. Ann Arbor, Michigan : ProQuest Ebook Central, 2017. Available via World Wide Web. Access may be limited to ProQuest Ebook Central affiliated libraries.
Genre:
Electronic Access:
Click to View