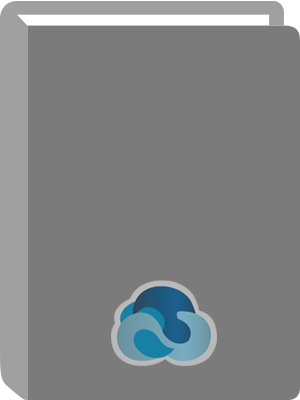
Case Studies in Bayesian Statistical Modelling and Analysis.
Title:
Case Studies in Bayesian Statistical Modelling and Analysis.
Author:
Alston, Clair L.
ISBN:
9781118394496
Personal Author:
Edition:
1st ed.
Physical Description:
1 online resource (499 pages)
Series:
Wiley Series in Probability and Statistics
Contents:
Case Studies in Bayesian Statistical Modelling and Analysis -- Contents -- Preface -- List of contributors -- 1 Introduction -- 1.1 Introduction -- 1.2 Overview -- 1.3 Further reading -- 1.3.1 Bayesian theory and methodology -- 1.3.2 Bayesian methodology -- 1.3.3 Bayesian computation -- 1.3.4 Bayesian software -- 1.3.5 Applications -- References -- 2 Introduction to MCMC -- 2.1 Introduction -- 2.2 Gibbs sampling -- 2.2.1 Example: Bivariate normal -- 2.2.2 Example: Change-point model -- 2.3 Metropolis-Hastings algorithms -- 2.3.1 Example: Component-wise MH or MH within Gibbs -- 2.3.2 Extensions to basic MCMC -- 2.3.3 Adaptive MCMC -- 2.3.4 Doubly intractable problems -- 2.4 Approximate Bayesian computation -- 2.5 Reversible jump MCMC -- 2.6 MCMC for some further applications -- References -- 3 Priors: Silent or active partners of Bayesian inference? -- 3.1 Priors in the very beginning -- 3.1.1 Priors as a basis for learning -- 3.1.2 Priors and philosophy -- 3.1.3 Prior chronology -- 3.1.4 Pooling prior information -- 3.2 Methodology I: Priors defined by mathematical criteria -- 3.2.1 Conjugate priors -- 3.2.2 Impropriety and hierarchical priors -- 3.2.3 Zellner's g-prior for regression models -- 3.2.4 Objective priors -- 3.3 Methodology II: Modelling informative priors -- 3.3.1 Informative modelling approaches -- 3.3.2 Elicitation of distributions -- 3.4 Case studies -- 3.4.1 Normal likelihood: Time to submit research dissertations -- 3.4.2 Binomial likelihood: Surveillance for exotic plant pests -- 3.4.3 Mixture model likelihood: Bioregionalization -- 3.4.4 Logistic regression likelihood: Mapping species distribution via habitat models -- 3.5 Discussion -- 3.5.1 Limitations -- 3.5.2 Finding out about the problem -- 3.5.3 Prior formulation -- 3.5.4 Communication -- 3.5.5 Conclusion -- Acknowledgements -- References.
4 Bayesian analysis of the normal linear regression model -- 4.1 Introduction -- 4.2 Case studies -- 4.2.1 Case study 1: Boston housing data set -- 4.2.2 Case study 2: Production of cars and station wagons -- 4.3 Matrix notation and the likelihood -- 4.4 Posterior inference -- 4.4.1 Natural conjugate prior -- 4.4.2 Alternative prior specifications -- 4.4.3 Generalizations of the normal linear model -- 4.4.4 Variable selection -- 4.5 Analysis -- 4.5.1 Case study 1: Boston housing data set -- 4.5.2 Case study 2: Car production data set -- References -- 5 Adapting ICU mortality models for local data: A Bayesian approach -- 5.1 Introduction -- 5.2 Case study: Updating a known risk-adjustment model for local use -- 5.3 Models and methods -- 5.4 Data analysis and results -- 5.4.1 Updating using the training data -- 5.4.2 Updating the model yearly -- 5.5 Discussion -- References -- 6 A Bayesian regression model with variable selection for genome-wide association studies -- 6.1 Introduction -- 6.2 Case study: Case-control of Type 1 diabetes -- 6.3 Case study: GENICA -- 6.4 Models and methods -- 6.4.1 Main effect models -- 6.4.2 Main effects and interactions -- 6.5 Data analysis and results -- 6.5.1 WTCCC TID -- 6.5.2 GENICA -- 6.6 Discussion -- Acknowledgements -- References -- 6.A Appendix: SNP IDs -- 7 Bayesian meta-analysis -- 7.1 Introduction -- 7.2 Case study 1: Association between red meat consumption and breast cancer -- 7.2.1 Background -- 7.2.2 Meta-analysis models -- 7.2.3 Computation -- 7.2.4 Results -- 7.2.5 Discussion -- 7.3 Case study 2: Trends in fish growth rate and size -- 7.3.1 Background -- 7.3.2 Meta-analysis models -- 7.3.3 Computation -- 7.3.4 Results -- 7.3.5 Discussion -- Acknowledgements -- References -- 8 Bayesian mixed effects models -- 8.1 Introduction -- 8.2 Case studies -- 8.2.1 Case study 1: Hot carcase weight of sheep carcases.
8.2.2 Case study 2: Growth of primary school girls -- 8.3 Models and methods -- 8.3.1 Model for Case study 1 -- 8.3.2 Model for Case study 2 -- 8.3.3 MCMC estimation -- 8.4 Data analysis and results -- 8.5 Discussion -- References -- 9 Ordering of hierarchies in hierarchical models: Bone mineral density estimation -- 9.1 Introduction -- 9.2 Case study -- 9.2.1 Measurement of bone mineral density -- 9.3 Models -- 9.3.1 Hierarchical model -- 9.3.2 Model H1 -- 9.3.3 Model H2 -- 9.4 Data analysis and results -- 9.4.1 Model H1 -- 9.4.2 Model H2 -- 9.4.3 Implication of ordering -- 9.4.4 Simulation study -- 9.4.5 Study design -- 9.4.6 Simulation study results -- 9.5 Discussion -- References -- 9.A Appendix: Likelihoods -- 10 Bayesian Weibull survival model for gene expression data -- 10.1 Introduction -- 10.2 Survival analysis -- 10.3 Bayesian inference for the Weibull survival model -- 10.3.1 Weibull model without covariates -- 10.3.2 Weibull model with covariates -- 10.3.3 Model evaluation and comparison -- 10.4 Case study -- 10.4.1 Weibull model without covariates -- 10.4.2 Weibull survival model with covariates -- 10.4.3 Model evaluation and comparison -- 10.5 Discussion -- References -- 11 Bayesian change point detection in monitoring clinical outcomes -- 11.1 Introduction -- 11.2 Case study: Monitoring intensive care unit outcomes -- 11.3 Risk-adjusted control charts -- 11.4 Change point model -- 11.5 Evaluation -- 11.6 Performance analysis -- 11.7 Comparison of Bayesian estimator with other methods -- 11.8 Conclusion -- References -- 12 Bayesian splines -- 12.1 Introduction -- 12.2 Models and methods -- 12.2.1 Splines and linear models -- 12.2.2 Link functions -- 12.2.3 Bayesian splines -- 12.2.4 Markov chain Monte Carlo -- 12.2.5 Model choice -- 12.2.6 Posterior diagnostics -- 12.3 Case studies -- 12.3.1 Data -- 12.3.2 Analysis -- 12.4 Conclusion.
12.4.1 Discussion -- 12.4.2 Extensions -- 12.4.3 Summary -- References -- 13 Disease mapping using Bayesian hierarchical models -- 13.1 Introduction -- 13.2 Case studies -- 13.2.1 Case study 1: Spatio-temporal model examining the incidence of birth defects -- 13.2.2 Case study 2: Relative survival model examining survival from breast cancer -- 13.3 Models and methods -- 13.3.1 Case study 1 -- 13.3.2 Case study 2 -- 13.4 Data analysis and results -- 13.4.1 Case study 1 -- 13.4.2 Case study 2 -- 13.5 Discussion -- References -- 14 Moisture, crops and salination: An analysis of a three-dimensional agricultural data set -- 14.1 Introduction -- 14.2 Case study -- 14.2.1 Data -- 14.2.2 Aim of the analysis -- 14.3 Review -- 14.3.1 General methodology -- 14.3.2 Computations -- 14.4 Case study modelling -- 14.4.1 Modelling framework -- 14.5 Model implementation: Coding considerations -- 14.5.1 Neighbourhood matrices and CAR models -- 14.5.2 Design matrices vs indexing -- 14.6 Case study results -- 14.7 Conclusions -- References -- 15 A Bayesian approach to multivariate state space modelling: A study of a Fama-French asset-pricing model with time-varying regressors -- 15.1 Introduction -- 15.2 Case study: Asset pricing in financial markets -- 15.2.1 Data -- 15.3 Time-varying Fama-French model -- 15.3.1 Specific models under consideration -- 15.4 Bayesian estimation -- 15.4.1 Gibbs sampler -- 15.4.2 Sampling Σε -- 15.4.3 Sampling β1:n -- 15.4.4 Sampling Σα -- 15.4.5 Likelihood calculation -- 15.5 Analysis -- 15.5.1 Prior elicitation -- 15.5.2 Estimation output -- 15.6 Conclusion -- References -- 16 Bayesian mixture models: When the thing you need to know is the thing you cannot measure -- 16.1 Introduction -- 16.2 Case study: CT scan images of sheep -- 16.3 Models and methods -- 16.3.1 Bayesian mixture models.
16.3.2 Parameter estimation using the Gibbs sampler -- 16.3.3 Extending the model to incorporate spatial information -- 16.4 Data analysis and results -- 16.4.1 Normal Bayesian mixture model -- 16.4.2 Spatial mixture model -- 16.4.3 Carcase volume calculation -- 16.5 Discussion -- References -- 17 Latent class models in medicine -- 17.1 Introduction -- 17.2 Case studies -- 17.2.1 Case study 1: Parkinson's disease -- 17.2.2 Case study 2: Cognition in breast cancer -- 17.3 Models and methods -- 17.3.1 Finite mixture models -- 17.3.2 Trajectory mixture models -- 17.3.3 Goodness of fit -- 17.3.4 Label switching -- 17.3.5 Model computation -- 17.4 Data analysis and results -- 17.4.1 Case study 1: Phenotype identification in PD -- 17.4.2 Case study 2: Trajectory groups for verbal memory -- 17.5 Discussion -- References -- 18 Hidden Markov models for complex stochastic processes: A case study in electrophysiology -- 18.1 Introduction -- 18.2 Case study: Spike identification and sorting of extracellular recordings -- 18.3 Models and methods -- 18.3.1 What is an HMM? -- 18.3.2 Modelling a single AP: Application of a simple HMM -- 18.3.3 Multiple neurons: An application of a factorial HMM -- 18.3.4 Model estimation and inference -- 18.4 Data analysis and results -- 18.4.1 Simulation study -- 18.4.2 Case study: Extracellular recordings collected during deep brain stimulation -- 18.5 Discussion -- References -- 19 Bayesian classification and regression trees -- 19.1 Introduction -- 19.2 Case studies -- 19.2.1 Case study 1: Kyphosis -- 19.2.2 Case study 2: Cryptosporidium -- 19.3 Models and methods -- 19.3.1 CARTs -- 19.3.2 Bayesian CARTs -- 19.4 Computation -- 19.4.1 Building the BCART model - stochastic search -- 19.4.2 Model diagnostics and identifying good trees -- 19.5 Case studies - results -- 19.5.1 Case study 1: Kyphosis.
19.5.2 Case study 2: Cryptosporidium.
Abstract:
Provides an accessible foundation to Bayesian analysis using real world models This book aims to present an introduction to Bayesian modelling and computation, by considering real case studies drawn from diverse fields spanning ecology, health, genetics and finance. Each chapter comprises a description of the problem, the corresponding model, the computational method, results and inferences as well as the issues that arise in the implementation of these approaches. Case Studies in Bayesian Statistical Modelling and Analysis: Illustrates how to do Bayesian analysis in a clear and concise manner using real-world problems. Each chapter focuses on a real-world problem and describes the way in which the problem may be analysed using Bayesian methods. Features approaches that can be used in a wide area of application, such as, health, the environment, genetics, information science, medicine, biology, industry and remote sensing. Case Studies in Bayesian Statistical Modelling and Analysis is aimed at statisticians, researchers and practitioners who have some expertise in statistical modelling and analysis, and some understanding of the basics of Bayesian statistics, but little experience in its application. Graduate students of statistics and biostatistics will also find this book beneficial.
Local Note:
Electronic reproduction. Ann Arbor, Michigan : ProQuest Ebook Central, 2017. Available via World Wide Web. Access may be limited to ProQuest Ebook Central affiliated libraries.
Genre:
Electronic Access:
Click to View