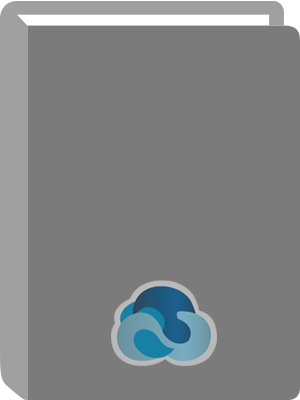
Modern Industrial Statistics : with applications in R, MINITAB and JMP.
Title:
Modern Industrial Statistics : with applications in R, MINITAB and JMP.
Author:
Kenett, Ron.
ISBN:
9781118763681
Personal Author:
Edition:
2nd ed.
Physical Description:
1 online resource (587 pages)
Series:
Statistics in Practice
Contents:
Cover -- Title Page -- Copyright -- Contents -- Preface to Second Edition -- Preface to First Edition -- Abbreviations -- Part I Principles of Statistical Thinking and Analysis -- Chapter 1 The Role of Statistical Methods in Modern Industry and Services -- 1.1 The different functional areas in industry and services -- 1.2 The quality-productivity dilemma -- 1.3 Fire-fighting -- 1.4 Inspection of products -- 1.5 Process control -- 1.6 Quality by design -- 1.7 Information quality and practical statistical efficiency -- 1.8 Chapter highlights -- 1.9 Exercises -- Chapter 2 Analyzing Variability: Descriptive Statistics -- 2.1 Random phenomena and the structure of observations -- 2.2 Accuracy and precision of measurements -- 2.3 The population and the sample -- 2.4 Descriptive analysis of sample values -- 2.4.1 Frequency distributions of discrete random variables -- 2.4.2 Frequency distributions of continuous random variables -- 2.4.3 Statistics of the ordered sample -- 2.4.4 Statistics of location and dispersion -- 2.5 Prediction intervals -- 2.6 Additional techniques of exploratory data analysis -- 2.6.1 Box and whiskers plot -- 2.6.2 Quantile plots -- 2.6.3 Stem-and-leaf diagrams -- 2.6.4 Robust statistics for location and dispersion -- 2.7 Chapter highlights -- 2.8 Exercises -- Chapter 3 Probability Models and Distribution Functions -- 3.1 Basic probability -- 3.1.1 Events and sample spaces: Formal presentation of random measurements -- 3.1.2 Basic rules of operations with events: Unions, intersections -- 3.1.3 Probabilities of events -- 3.1.4 Probability functions for random sampling -- 3.1.5 Conditional probabilities and independence of events -- 3.1.6 Bayes formula and its application -- 3.2 Random variables and their distributions -- 3.2.1 Discrete and continuous distributions.
3.2.2 Expected values and moments of distributions -- 3.2.3 The standard deviation, quantiles, measures of skewness and kurtosis -- 3.2.4 Moment generating functions -- 3.3 Families of discrete distribution -- 3.3.1 The binomial distribution -- 3.3.2 The hypergeometric distribution -- 3.3.3 The Poisson distribution -- 3.3.4 The geometric and negative binomial distributions -- 3.4 Continuous distributions -- 3.4.1 The uniform distribution on the interval (a, b), a < b -- 3.4.2 The normal and log-normal distributions -- 3.4.3 The exponential distribution -- 3.4.4 The gamma and Weibull distributions -- 3.4.5 The Beta distributions -- 3.5 Joint, marginal and conditional distributions -- 3.5.1 Joint and marginal distributions -- 3.5.2 Covariance and correlation -- 3.5.3 Conditional distributions -- 3.6 Some multivariate distributions -- 3.6.1 The multinomial distribution -- 3.6.2 The multi-hypergeometric distribution -- 3.6.3 The bivariate normal distribution -- 3.7 Distribution of order statistics -- 3.8 Linear combinations of random variables -- 3.9 Large sample approximations -- 3.9.1 The law of large numbers -- 3.9.2 The Central Limit Theorem -- 3.9.3 Some normal approximations -- 3.10 Additional distributions of statistics of normal samples -- 3.10.1 Distribution of the sample variance -- 3.10.2 The "Student'' t-statistic -- 3.10.3 Distribution of the variance ratio -- 3.11 Chapter highlights -- 3.12 Exercises -- Chapter 4 Statistical Inference and Bootstrapping -- 4.1 Sampling characteristics of estimators -- 4.2 Some methods of point estimation -- 4.2.1 Moment equation estimators -- 4.2.2 The method of least squares -- 4.2.3 Maximum likelihood estimators -- 4.3 Comparison of sample estimates -- 4.3.1 Basic concepts -- 4.3.2 Some common one-sample tests of hypotheses -- 4.4 Confidence intervals -- 4.4.1 Confidence intervals for m -- s known.
4.4.2 Confidence intervals for m -- s unknown -- 4.4.3 Confidence intervals for Q2 -- 4.4.4 Confidence intervals for p -- 4.5 Tolerance intervals -- 4.5.1 Tolerance intervals for the normal distributions -- 4.6 Testing for normality with probability plots -- 4.7 Tests of goodness of fit -- 4.7.1 The chi-square test (large samples) -- 4.7.2 The Kolmogorov-Smirnov test -- 4.8 Bayesian decision procedures -- 4.8.1 Prior and posterior distributions -- 4.8.2 Bayesian testing and estimation -- 4.8.3 Credibility intervals for real parameters -- 4.9 Random sampling from reference distributions -- 4.10 Bootstrap sampling -- 4.10.1 The bootstrap method -- 4.10.2 Examining the bootstrap method -- 4.10.3 Harnessing the bootstrap method -- 4.11 Bootstrap testing of hypotheses -- 4.11.1 Bootstrap testing and confidence intervals for the mean -- 4.11.2 Studentized test for the mean -- 4.11.3 Studentized test for the difference of two means -- 4.11.4 Bootstrap tests and confidence intervals for the variance -- 4.11.5 Comparing statistics of several samples -- 4.12 Bootstrap tolerance intervals -- 4.12.1 Bootstrap tolerance intervals for Bernoulli samples -- 4.12.2 Tolerance interval for continuous variables -- 4.12.3 Distribution-free tolerance intervals -- 4.13 Non-parametric tests -- 4.13.1 The sign test -- 4.13.2 The randomization test -- 4.13.3 The Wilcoxon Signed Rank test -- 4.14 Description of MINITAB macros (available for download from Appendix VI of the book website) -- 4.15 Chapter highlights -- 4.16 Exercises -- Chapter 5 Variability in Several Dimensions and Regression Models -- 5.1 Graphical display and analysis -- 5.1.1 Scatterplots -- 5.1.2 Multiple boxplots -- 5.2 Frequency distributions in several dimensions -- 5.2.1 Bivariate joint frequency distributions -- 5.2.2 Conditional distributions.
5.3 Correlation and regression analysis -- 5.3.1 Covariances and correlations -- 5.3.2 Fitting simple regression lines to data -- 5.4 Multiple regression -- 5.4.1 Regression on two variables -- 5.5 Partial regression and correlation -- 5.6 Multiple linear regression -- 5.7 Partial F-tests and the sequential SS -- 5.8 Model construction: Step-wise regression -- 5.9 Regression diagnostics -- 5.10 Quantal response analysis: Logistic regression -- 5.11 The analysis of variance: The comparison of means -- 5.11.1 The statistical model -- 5.11.2 The one-way analysis of variance (ANOVA) -- 5.12 Simultaneous confidence intervals: Multiple comparisons -- 5.13 Contingency tables -- 5.13.1 The structure of contingency tables -- 5.13.2 Indices of association for contingency tables -- 5.14 Categorical data analysis -- 5.14.1 Comparison of binomial experiments -- 5.15 Chapter highlights -- 5.16 Exercises -- Part II Acceptance Sampling -- Chapter 6 Sampling for Estimation of Finite Population Quantities -- 6.1 Sampling and the estimation problem -- 6.1.1 Basic definitions -- 6.1.2 Drawing a random sample from a finite population -- 6.1.3 Sample estimates of population quantities and their sampling distribution -- 6.2 Estimation with simple random samples -- 6.2.1 Properties of Xn and Sn2 under RSWR -- 6.2.2 Properties of Xn and Sn2 under RSWOR -- 6.3 Estimating the mean with stratified RSWOR -- 6.4 Proportional and optimal allocation -- 6.5 Prediction models with known covariates -- 6.6 Chapter highlights -- 6.7 Exercises -- Chapter 7 Sampling Plans for Product Inspection -- 7.1 General discussion -- 7.2 Single-stage sampling plans for attributes -- 7.3 Approximate determination of the sampling plan -- 7.4 Double-sampling plans for attributes -- 7.5 Sequential sampling -- 7.6 Acceptance sampling plans for variables.
7.7 Rectifying inspection of lots -- 7.8 National and international standards -- 7.9 Skip-lot sampling plans for attributes -- 7.9.1 The ISO 2859 skip-lot sampling procedures -- 7.10 The Deming inspection criterion -- 7.11 Published tables for acceptance sampling -- 7.12 Chapter highlights -- 7.13 Exercises -- Part III Statistical Process Control -- Chapter 8 Basic Tools and Principles of Process Control -- 8.1 Basic concepts of statistical process control -- 8.2 Driving a process with control charts -- 8.3 Setting up a control chart: Process capability studies -- 8.4 Process capability indices -- 8.5 Seven tools for process control and process improvement -- 8.6 Statistical analysis of Pareto charts -- 8.7 The Shewhart control charts -- 8.7.1 Control charts for attributes -- 8.7.2 Control charts for variables -- 8.8 Chapter highlights -- 8.9 Exercises -- Chapter 9 Advanced Methods of Statistical Process Control -- 9.1 Tests of randomness -- 9.1.1 Testing the number of runs -- 9.1.2 Runs above and below a specified level -- 9.1.3 Runs up and down -- 9.1.4 Testing the length of runs up and down -- 9.2 Modified Shewhart control charts for X -- 9.3 The size and frequency of sampling for Shewhart control charts -- 9.3.1 The economic design for X-charts -- 9.3.2 Increasing the sensitivity of p-charts -- 9.4 Cumulative sum control charts -- 9.4.1 Upper Page's scheme -- 9.4.2 Some theoretical background -- 9.4.3 Lower and two-sided Page's scheme -- 9.4.4 Average run length, probability of false alarm and conditional expected delay -- 9.5 Bayesian detection -- 9.6 Process tracking -- 9.6.1 The EWMA procedure -- 9.6.2 The BECM procedure -- 9.6.3 The Kalman filter -- 9.6.4 Hoadley's QMP -- 9.7 Automatic process control -- 9.8 Chapter highlights -- 9.9 Exercises.
Chapter 10 Multivariate Statistical Process Control.
Abstract:
Fully revised and updated, this book combines a theoretical background with examples and references to R, MINITAB and JMP, enabling practitioners to find state-of-the-art material on both foundation and implementation tools to support their work. Topics addressed include computer-intensive data analysis, acceptance sampling, univariate and multivariate statistical process control, design of experiments, quality by design, and reliability using classical and Bayesian methods. The book can be used for workshops or courses on acceptance sampling, statistical process control, design of experiments, and reliability. Graduate and post-graduate students in the areas of statistical quality and engineering, as well as industrial statisticians, researchers and practitioners in these fields will all benefit from the comprehensive combination of theoretical and practical information provided in this single volume. Modern Industrial Statistics: With applications in R, MINITAB and JMP: Combines a practical approach with theoretical foundations and computational support. Provides examples in R using a dedicated package called MISTAT, and also refers to MINITAB and JMP. Includes exercises at the end of each chapter to aid learning and test knowledge. Provides over 40 data sets representing real-life case studies. Is complemented by a comprehensive website providing an introduction to R, and installations of JMP scripts and MINITAB macros, including effective tutorials with introductory material: www.wiley.com/go/modern_industrial_statistics.
Local Note:
Electronic reproduction. Ann Arbor, Michigan : ProQuest Ebook Central, 2017. Available via World Wide Web. Access may be limited to ProQuest Ebook Central affiliated libraries.
Genre:
Electronic Access:
Click to View