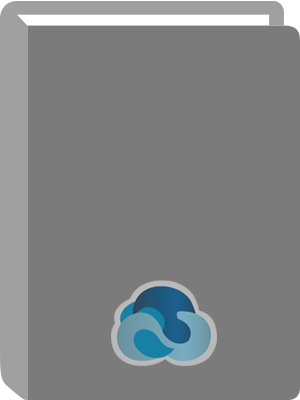
Supervision and Safety of Complex Systems.
Title:
Supervision and Safety of Complex Systems.
Author:
Matta, Nada.
ISBN:
9781118588017
Personal Author:
Edition:
1st ed.
Physical Description:
1 online resource (313 pages)
Series:
Iste
Contents:
Cover -- Supervision and Safety of Complex Systems -- Title Page -- Copyright Page -- Table of Contents -- Foreword -- Foreword -- Introduction -- PART 1. INDUSTRIAL ISSUES -- Chapter 1. Safety and Performance of Electricity Production Facilities -- Chapter 2. Monitoring of Radioactive Waste Disposal Cells in Deep Geological Formation -- 2.1. Context -- 2.2. Monitoring of the environment -- 2.3. Monitoring of geological repository structures -- 2.4. Conclusion and perspectives -- Chapter 3. Towards Fourth-generation Nuclear Reactors -- 3.1. Context -- 3.2. Surveillance and acoustic detection -- 3.3. Inspection during operation -- 3.3.1. The case of acoustic measurements -- 3.4. Conclusion -- PART 2. SUPERVISION AND MODELING OF COMPLEX SYSTEMS -- Chapter 4. Fault-tolerant Data-fusion Method: Application on Platoon Vehicle Localization -- 4.1. Introduction -- 4.2. Review -- 4.3. Bayesian network for data fusion -- 4.3.1. Bayesian network and Kalman filter -- 4.4. Localization of a single vehicle: multisensor data fusion with a dynamic Bayesian network -- 4.4.1. Presentation of the approach developed -- 4.4.2. Inference in switching Kalman filter -- 4.4.3. Detailed synopsis of the method based on Bayesian networks -- 4.4.4. Example of management of multi-hypotheses by a Bayesian network -- 4.4.5. Illustration of the map localization method using SKF -- 4.5. Multi-vehicle localization -- 4.5.1. The problem studied -- 4.5.2. Communication within the convoy -- 4.5.3. Sensors used on each vehicle in the convoy -- 4.5.4. Bayesian network for the localization of a chain of vehicles -- 4.5.5. Extension of the approach: modeling and localization of a chain of vehicles -- 4.5.6. The issue with this model -- 4.5.7. New model for the localization of a chain of vehicles -- 4.5.8. Proportional commands -- 4.5.9. Functional analysis of models of the convoy.
4.6. Conclusions and perspectives -- 4.7. Bibliography -- Chapter 5 Damage and Forecast Modeling -- 5.1. Introduction -- 5.1.1. Operational level -- 5.1.2. Strategic level -- 5.2. Preliminary study of data -- 5.2.1. Structure of the database -- 5.2.2. Performance criterion for the prognostic -- 5.2.3. Definition of a deterioration indicator -- 5.3. Construction of the deterioration indicator -- 5.3.1. Study of the failure space with PCA -- 5.3.2. Damage indicator defined as a distance -- 5.4. Estimation of the residual life span (RUL) -- 5.4.1. Simple approach based on the life span -- 5.4.2. Stochastic deterioration model -- 5.5. Conclusion -- 5.6. Bibliography -- Chapter 6. Diagnosis of Systems with Multiple Operating Modes -- 6.1. Introduction -- 6.2. Detection of faults for a class of switching systems -- 6.2.1. Introduction -- 6.2.2. Structure of the residual generator and observer design -- 6.2.3. Simulation and results -- 6.2.4. Conclusions -- 6.3. Analytical method to obtain a multiple model -- 6.3.1. Introduction -- 6.3.2. Setting the problem -- 6.3.3. Transformation in multiple-model form -- 6.3.4. Conclusion -- 6.4. Detection of switching and operating mode recognition without the explicituse of model parameters -- 6.4.1. Introduction -- 6.4.2. Diagnosis of SSs with linear modes -- 6.4.3. Diagnosis of a switching system with uncertain nonlinear modes -- 6.4.4. Conclusions -- 6.5. Modeling, observation and monitoring of switching systems: application toa multicellular converter -- 6.5.1. Introduction -- 6.5.2. Multicellular converter with two arms or four quadrants -- 6.5.3. Diagnosing faults in the four quadrant converter -- 6.5.4. Experimental benchmark for validation -- 6.6. Bibliography -- Chapter 7. Multitask Learning for the Diagnosis of Machine Fleet -- 7.1. Introduction -- 7.2. Single-task learning of one-class SVM classifier.
7.3. Multitask learning of 1-SVM classifiers -- 7.3.1. Formulation of the problem -- 7.3.2. Dual problem -- 7.4. Experimental results -- 7.4.1. Academic nonlinear example -- 7.4.2. Analysis of textured images -- 7.5. Conclusion -- 7.6. Acknowledgments -- 7.7. Bibliography -- Chapter 8. The APPRODYN Project: Dynamic Reliability Approaches to Modeling Critical Systems -- 8.1.Context and aims -- 8.1.1.Context -- 8.1.2. Objectives -- 8.2. Brief overview of the test case -- 8.2.1. General remarks -- 8.2.2. Functional description -- 8.2.3. Modeling the process -- 8.2.4. Modeling command logic -- 8.2.5. Reliability data and state graphs -- 8.2.6. Ageing -- 8.2.7. Sensors -- 8.3. Modeling using a stochastic hybrid automaton approach -- 8.3.1. Main concepts and references -- 8.3.2. What is a stochastic hybrid automaton? -- 8.3.3. Structuring and synchronization approach -- 8.3.4. Modeling the case study -- 8.3.5. Qualitative and quantitative results -- 8.3.6. Conclusion and perspectives for the stochastic hybrid automaton approach -- 8.4. Modeling using piecewise deterministic Markov processes -- 8.4.1. Principles and references -- 8.4.2. What is a piecewise deterministic Markov process? -- 8.4.3. Modeling the test case -- 8.4.4. Modeling the VVP -- 8.4.5. Modeling CEX -- 8.4.6. Qualitative and quantitative results -- 8.4.7. Conclusion and perspectives for the piecewise deterministic Markov processes and simulation approach -- 8.5. Modeling using stochastic Petri nets -- 8.5.1. Principles and references -- 8.5.2. What is a stochastic Petri net? -- 8.5.3. Modeling framework -- 8.5.4. Qualitative and quantitative results -- 8.5.5. SPN approach: conclusions and perspectives -- 8.6. Preliminary conclusion and perspectives -- 8.7. Bibliography.
PART 3. CHARACTERIZING BACKGROUND NOISE, IDENTIFYING CHARACTERISTIC SIGNATURES IN TEST CASES AND DETECTING NOISE REACTORS -- Chapter 9. Aims, Context and Type of Signals Studied -- Chapter 10. Detection/Classification of Argon and Water Injections into Sodium into an SG of a Fast Neutron Reactor -- 10.1. Context and aims -- 10.2. Data -- 10.3. Online (sequential) detection-isolation -- 10.3.1. Formulating the practical problem -- 10.3.2. Formulating the statistical problem -- 10.3.3. Non-recursive approach -- 10.3.4. Recursive approach -- 10.3.5. Practical algorithm -- 10.3.6. Experimental results -- 10.4. Offline classification (non-sequential) -- 10.4.1. Characterization and approach used -- 10.4.2. Initial characterization -- 10.4.3. Effective features -- 10.4.4. Classification -- 10.4.5. Performance evaluation -- 10.4.6. Experimental results -- 10.5. Results and comments -- 10.6. Conclusion -- 10.7. Bibliography -- Chapter 11. A Dynamic Learning-based Approach to the Surveillance and Monitoring of Steam Generators in Prototype Fast Reactors -- 11.1. Introduction -- 11.2. Proposed method for the surveillance and monitoring of a steam generator -- 11.2.1. Learning and classification -- 11.2.2. Detecting the evolution of a class -- 11.2.3. Adapting a class after validating its evolution and creating a new class -- 11.2.4. Validating classes -- 11.2.5. Defining the parameters of the SS-DFKNN method -- 11.3. Results -- 11.3.1. Data analysis -- 11.3.2. Classification results -- 11.3.3. Designing an automaton to improve classification rates -- 11.4. Conclusion and perspectives -- 11.5. Bibliography -- Chapter 12. SVM Time-Frequency Classification for the Detection of Injection States -- 12.1. Introduction -- 12.2. Preliminary examination of the data -- 12.2.1. Approach -- 12.2.2. Spectral analysis of the data -- 12.2.3. Class visualization.
12.3. Detection algorithm -- 12.3.1. SVM implementation -- 12.3.2. Algorithm calibration -- 12.4. Role of sensors -- 12.5. Experimental results -- 12.6. Bibliography -- Chapter 13. Time and Frequency Domain Approaches for the Characterization of Injection States -- 13.1. Introduction -- 13.1.1. Framework of the study -- 13.1.2. Processing recordings -- 13.1.3. Identifying the injection zones -- 13.1.4. Extraction of "non-injection" zones -- 13.2. Analyzing the statistical properties of spectral power densities -- 13.2.1. Methodology -- 13.2.2. Results -- 13.2.3. Exploring implementation in a new installation -- 13.3. Analysis of the filtering characteristics -- 13.3.1. Estimating filtering characteristics using an AR model -- 13.3.2. Comparing filtering characteristics -- 13.3.3. A leak detection algorithm -- 13.3.4. Conclusions on the autoregressive signal modeling-based approach -- 13.4. Conclusion on frequential and temporal approaches -- 13.5. Bibliography -- PART 4. HUMAN, ORGANIZATIONAL AND ENVIRONMENTAL FACTORS IN RISK ANALYSIS -- Chapter 14. Risk Analysis and Management in Systems Integrating Technical, Human, Organizational and Environmental Aspects -- 14.1. Aims of the project -- 14.2. State of the art -- 14.2.1. Context of the study -- 14.2.2. Towards an "integrated" approach to risk: combining several specialist disciplines -- 14.3. Integrated risk analysis -- 14.3.1. Concepts -- 14.3.2. A description of the approach -- 14.4. Accounting for uncertainty in risk analysis -- 14.4.1. Different kinds and sources of uncertainty -- 14.4.2. Frameworks for modeling uncertainty -- 14.5. Modeling risk for a quantitative assessment of risk -- 14.5.1. Bayesian networks -- 14.5.2. Evaluating risk beyond a probabilistic framework -- 14.6. Conclusions and future perspectives -- 14.7. Bibliography.
Chapter 15. Integrating Human and Organizational Factors into the BCD Risk Analysis Model: An Influence Diagram-based Approach.
Abstract:
This book presents results of projects carried out by both scientific and industry researchers into the techniques to help in maintenance, control, supervision and security of systems, taking into account the technical environmental and human factors. This work is supported by the Scientific Group GIS 3SGS. It is a collaborative work from 13 partners (academic and industrial) who have come together to deal with security problems. The problems and techniques discussed mainly focus on stochastic and dynamic modeling, maintenance, forecasting, diagnosis, reliability, performance, organizational, human and environmental factors, uncertainty and experience feedback.
Local Note:
Electronic reproduction. Ann Arbor, Michigan : ProQuest Ebook Central, 2017. Available via World Wide Web. Access may be limited to ProQuest Ebook Central affiliated libraries.
Subject Term:
Genre:
Electronic Access:
Click to View