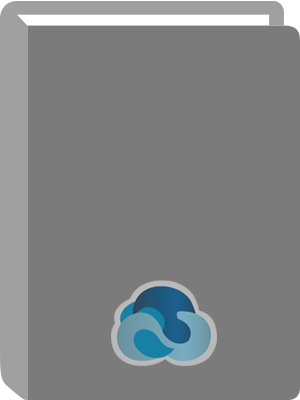
Handbook of Volatility Models and Their Applications.
Title:
Handbook of Volatility Models and Their Applications.
Author:
Bauwens, Luc.
ISBN:
9781118271995
Personal Author:
Edition:
1st ed.
Physical Description:
1 online resource (565 pages)
Series:
Wiley Handbooks in Financial Engineering and Econometrics ; v.3
Wiley Handbooks in Financial Engineering and Econometrics
Contents:
Handbook of Volatility Models and Their Applications -- Contents -- Preface -- Contributors -- 1 Volatility Models -- 1.1 Introduction -- 1.2 GARCH -- 1.2.1 Univariate GARCH -- 1.2.1.1 Structure of GARCH Models -- 1.2.1.2 Early GARCH Models -- 1.2.1.3 Probability Distributions for zt -- 1.2.1.4 New GARCH Models -- 1.2.1.5 Explanation of Volatility Clustering -- 1.2.1.6 Literature and Software -- 1.2.1.7 Applications of Univariate GARCH -- 1.2.2 Multivariate GARCH -- 1.2.2.1 Structure of MGARCH Models -- 1.2.2.2 Conditional Correlations -- 1.2.2.3 Factor Models -- 1.3 Stochastic Volatility -- 1.3.1 Leverage Effect -- 1.3.2 Estimation -- 1.3.3 Multivariate SV Models -- 1.3.4 Model Selection -- 1.3.5 Empirical Example: S&P 500 -- 1.3.6 Literature -- 1.4 Realized Volatility -- 1.4.1 Realized Variance -- 1.4.1.1 Empirical Application -- 1.4.2 Realized Covariance -- 1.4.2.1 Realized Quadratic Covariation -- 1.4.2.2 Realized Bipower Covariation -- Acknowledgments -- part one Autoregressive Conditional Heteroskedasticity and Stochastic Volatility -- 2 Nonlinear Models for Autoregressive Conditional Heteroskedasticity -- 2.1 Introduction -- 2.2 The Standard GARCH Model -- 2.3 Predecessors to Nonlinear GARCH Models -- 2.4 Nonlinear ARCH and GARCH Models -- 2.4.1 Engle's Nonlinear GARCH Model -- 2.4.2 Nonlinear ARCH Model -- 2.4.3 Asymmetric Power GARCH Model -- 2.4.4 Smooth Transition GARCH Model -- 2.4.5 Double Threshold ARCH Model -- 2.4.6 Neural Network ARCH and GARCH Models -- 2.4.7 Time-Varying GARCH -- 2.4.8 Families of GARCH Models and their Probabilistic Properties -- 2.5 Testing Standard GARCH Against Nonlinear GARCH -- 2.5.1 Size and Sign Bias Tests -- 2.5.2 Testing GARCH Against Smooth Transition GARCH -- 2.5.3 Testing GARCH Against Artificial Neural Network GARCH -- 2.6 Estimation of Parameters in Nonlinear GARCH Models.
2.6.1 Smooth Transition GARCH -- 2.6.2 Neural Network GARCH -- 2.7 Forecasting with Nonlinear GARCH Models -- 2.7.1 Smooth Transition GARCH -- 2.7.2 Asymmetric Power GARCH -- 2.8 Models Based on Multiplicative Decomposition of the Variance -- 2.9 Conclusion -- Acknowledgments -- 3 Mixture and Regime-Switching GARCH Models -- 3.1 Introduction -- 3.2 Regime-Switching GARCH Models for Asset Returns -- 3.2.1 The Regime-Switching Framework -- 3.2.2 Modeling the Mixing Weights -- 3.2.3 Regime-Switching GARCH Specifications -- 3.3 Stationarity and Moment Structure -- 3.3.1 Stationarity -- 3.3.2 Moment Structure -- 3.4 Regime Inference, Likelihood Function, and Volatility Forecasting -- 3.4.1 Determining the Number of Regimes -- 3.4.2 Volatility Forecasts -- 3.4.3 Application of MS-GARCH Models to Stock Return Indices -- 3.5 Application of Mixture GARCH Models to Density Prediction and Value-at-Risk Estimation -- 3.5.1 Value-at-Risk -- 3.5.2 Data and Models -- 3.5.3 Empirical Results -- 3.6 Conclusion -- Acknowledgments -- 4 Forecasting High Dimensional Covariance Matrices -- 4.1 Introduction -- 4.2 Notation -- 4.3 Rolling Window Forecasts -- 4.3.1 Sample Covariance -- 4.3.2 Observable Factor Covariance -- 4.3.3 Statistical Factor Covariance -- 4.3.4 Equicorrelation -- 4.3.5 Shrinkage Estimators -- 4.4 Dynamic Models -- 4.4.1 Covariance Targeting Scalar VEC -- 4.4.2 Flexible Multivariate GARCH -- 4.4.3 Conditional Correlation GARCH Models -- 4.4.4 Orthogonal GARCH -- 4.4.5 RiskMetrics -- 4.4.6 Alternative Estimators for Multivariate GARCH Models -- 4.5 High Frequency Based Forecasts -- 4.5.1 Realized Covariance -- 4.5.2 Mixed-Frequency Factor Model Covariance -- 4.5.3 Regularization and Blocking Covariance -- 4.6 Forecast Evaluation -- 4.6.1 Portfolio Constraints -- 4.7 Conclusion -- Acknowledgments.
5 Mean, Volatility, and Skewness Spillovers in Equity Markets -- 5.1 Introduction -- 5.2 Data and Summary Statistics -- 5.2.1 Data -- 5.2.2 Time-Varying Skewness (Univariate Analysis) -- 5.2.3 Spillover Models -- 5.3 Empirical Results -- 5.3.1 Parameter Estimates -- 5.3.2 Spillover Effects in Variance and Skewness -- 5.3.2.1 Variance Ratios -- 5.3.2.2 Pattern and Size of Skewness Spillovers -- 5.4 Conclusion -- Acknowledgments -- 6 Relating Stochastic Volatility Estimation Methods -- 6.1 Introduction -- 6.2 Theory and Methodology -- 6.2.1 Quasi-Maximum Likelihood Estimation -- 6.2.2 Gaussian Mixture Sampling -- 6.2.3 Simulated Method of Moments -- 6.2.4 Methods Based on Importance Sampling -- 6.2.4.1 Approximating in the Basic IS Approach -- 6.2.4.2 Improving on IS with IIS -- 6.2.4.3 Alternative Efficiency Gains with EIS -- 6.2.5 Alternative Sampling Methods: SSS and MMS -- 6.3 Comparison of Methods -- 6.3.1 Setup of Data-Generating Process and Estimation Procedures -- 6.3.2 Parameter Estimates for the Simulation -- 6.3.3 Precision of IS -- 6.3.4 Precision of Bayesian Methods -- 6.4 Estimating Volatility Models in Practice -- 6.4.1 Describing Return Data of Goldman Sachs and IBM Stock -- 6.4.2 Estimating SV Models -- 6.4.3 Extracting Underlying Volatility -- 6.4.4 Relating the Returns in a Bivariate Model -- 6.5 Conclusion -- 7 Multivariate Stochastic Volatility Models -- 7.1 Introduction -- 7.2 MSV Model -- 7.2.1 Model -- 7.2.1.1 Likelihood Function -- 7.2.1.2 Prior Distribution -- 7.2.1.3 Posterior Distribution -- 7.2.2 Bayesian Estimation -- 7.2.2.1 Generation of a -- 7.2.2.2 Generation of o -- 7.2.2.3 Generation of s -- 7.2.3 Multivariate-t Errors -- 7.2.3.1 Generation of v -- 7.2.3.2 Generation of l -- 7.3 Factor MSV Model -- 7.3.1 Model -- 7.3.1.1 Likelihood Function -- 7.3.1.2 Prior and Posterior Distributions -- 7.3.2 Bayesian Estimation.
7.3.2.1 Generation of a, o, and s -- 7.3.2.2 Generation of f -- 7.3.2.3 Generation of l -- 7.3.2.4 Generation of b -- 7.3.2.5 Generation of v -- 7.4 Applications to Stock Indices Returns -- 7.4.1 S&P 500 Sector Indices -- 7.4.2 MSV Model with Multivariate t Errors -- 7.4.2.1 Prior Distributions -- 7.4.2.2 Estimation Results -- 7.4.3 Factor MSV Model -- 7.4.3.1 Prior Distributions -- 7.4.3.2 Estimation Results -- 7.5 Conclusion -- 7.6 Appendix: Sampling a in the MSV Model -- 7.6.1 Single-Move Sampler -- 7.6.2 Multi-move Sampler -- 8 Model Selection and Testing of Conditional and Stochastic Volatility Models -- 8.1 Introduction -- 8.1.1 Model Specifications -- 8.2 Model Selection and Testing -- 8.2.1 In-Sample Comparisons -- 8.2.2 Out-of-Sample Comparisons -- 8.2.2.1 Direct Model Evaluation -- 8.2.2.2 Indirect Model Evaluation -- 8.3 Empirical Example -- 8.4 Conclusion -- part two Other Models and Methods -- 9 Multiplicative Error Models -- 9.1 Introduction -- 9.2 Theory and Methodology -- 9.2.1 Model Formulation -- 9.2.1.1 Specifications for u -- 9.2.1.2 Specifications for et -- 9.2.2 Inference -- 9.2.2.1 Maximum Likelihood Inference -- 9.2.2.2 Generalized Method of Moments Inference -- 9.3 MEMs for Realized Volatility -- 9.4 MEM Extensions -- 9.4.1 Component Multiplicative Error Model -- 9.4.2 Vector Multiplicative Error Model -- 9.5 Conclusion -- 10 Locally Stationary Volatility Modeling -- 10.1 Introduction -- 10.2 Empirical Evidences -- 10.2.1 Structural Breaks, Nonstationarity, and Persistence -- 10.2.2 Testing Stationarity -- 10.3 Locally Stationary Processes and their Time-Varying Autocovariance Function -- 10.4 Locally Stationary Volatility Models -- 10.4.1 Multiplicative Models -- 10.4.2 Time-Varying ARCH Processes -- 10.4.3 Adaptive Approaches -- 10.5 Multivariate Models for Locally Stationary Volatility -- 10.5.1 Multiplicative Models.
10.5.2 Adaptive Approaches -- 10.6 Conclusions -- Acknowledgments -- 11 Nonparametric and Semiparametric Volatility Models: Specification, Estimation, and Testing -- 11.1 Introduction -- 11.2 Nonparametric and Semiparametric Univariate Volatility Models -- 11.2.1 Stationary Volatility Models -- 11.2.1.1 The Simplest Nonparametric Volatility Model -- 11.2.1.2 Additive Nonparametric Volatility Model -- 11.2.1.3 Functional-Coefficient Volatility Model -- 11.2.1.4 Single-Index Volatility Model -- 11.2.1.5 Stationary Semiparametric ARCH (D) Models -- 11.2.1.6 Semiparametric Combined Estimator of Volatility -- 11.2.1.7 Semiparametric Inference in GARCH-in-Mean Models -- 11.2.2 Nonstationary Univariate Volatility Models -- 11.2.3 Specification of the Error Density -- 11.2.4 Nonparametric Volatility Density Estimation -- 11.3 Nonparametric and Semiparametric Multivariate Volatility Models -- 11.3.1 Modeling the Conditional Covariance Matrix under Stationarity -- 11.3.1.1 Hafner, van Dijk, and Franses' Semiparametric Estimator -- 11.3.1.2 Long, Su, and Ullah's Semiparametric Estimator -- 11.3.1.3 Test for the Correct Specification of Parametric Conditional Covariance Models -- 11.3.2 Specification of the Error Density -- 11.4 Empirical Analysis -- 11.5 Conclusion -- Acknowledgments -- 12 Copula-Based Volatility Models -- 12.1 Introduction -- 12.2 Definition and Properties of Copulas -- 12.2.1 Sklar's Theorem -- 12.2.2 Conditional Copula -- 12.2.3 Some Commonly Used Bivariate Copulas -- 12.2.4 Copula-Based Dependence Measures -- 12.3 Estimation -- 12.3.1 Exact Maximum Likelihood -- 12.3.2 IFM -- 12.3.3 Bivariate Static Copula Models -- 12.4 Dynamic Copulas -- 12.4.1 Early Approaches -- 12.4.2 Dynamics Based on the DCC Model -- 12.4.3 Alternative Methods -- 12.5 Value-at-Risk -- 12.6 Multivariate Static Copulas -- 12.6.1 Multivariate Archimedean Copulas.
12.6.2 Vines.
Abstract:
A complete guide to the theory and practice of volatility models in financial engineering Volatility has become a hot topic in this era of instant communications, spawning a great deal of research in empirical finance and time series econometrics. Providing an overview of the most recent advances, Handbook of Volatility Models and Their Applications explores key concepts and topics essential for modeling the volatility of financial time series, both univariate and multivariate, parametric and non-parametric, high-frequency and low-frequency. Featuring contributions from international experts in the field, the book features numerous examples and applications from real-world projects and cutting-edge research, showing step by step how to use various methods accurately and efficiently when assessing volatility rates. Following a comprehensive introduction to the topic, readers are provided with three distinct sections that unify the statistical and practical aspects of volatility: Autoregressive Conditional Heteroskedasticity and Stochastic Volatility presents ARCH and stochastic volatility models, with a focus on recent research topics including mean, volatility, and skewness spillovers in equity markets Other Models and Methods presents alternative approaches, such as multiplicative error models, nonparametric and semi-parametric models, and copula-based models of (co)volatilities Realized Volatility explores issues of the measurement of volatility by realized variances and covariances, guiding readers on how to successfully model and forecast these measures Handbook of Volatility Models and Their Applications is an essential reference for academics and practitioners in finance, business, and econometrics who work with volatility models in their everyday work. The book also serves as a supplement for courses on risk management
and volatility at the upper-undergraduate and graduate levels.
Local Note:
Electronic reproduction. Ann Arbor, Michigan : ProQuest Ebook Central, 2017. Available via World Wide Web. Access may be limited to ProQuest Ebook Central affiliated libraries.
Genre:
Electronic Access:
Click to View