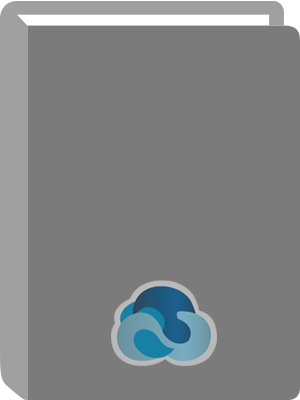
Lecture Notes in Data Mining.
Title:
Lecture Notes in Data Mining.
Author:
Berry, Michael W.
ISBN:
9789812773630
Personal Author:
Physical Description:
1 online resource (238 pages)
Contents:
CONTENTS -- Preface -- 1 Point Estimation Algorithms -- 1. Introduction -- 2. Motivation -- 3. Methods of Point Estimation -- 4. Measures of Performance -- 5. Summary -- 2 Applications of Bayes Theorem -- 1. Introduction -- 2. Motivation -- 3. The Bayes Approach for Classification -- 4. Examples -- 5. Summary -- 3 Similarity Measures -- 1. Introduction -- 2. Motivation -- 3. Classic Similarity Measures -- 4. Example -- 5. Current Applications -- 6. Summary -- 4 Decision Trees -- 1. Introduction -- 2. Motivation -- 3. Decision Tree Algorithms -- 4. Example: Classification of University Students -- 5. Applications of Decision Tree Algorithms -- 6. Summary -- 5 Genetic Algorithms -- 1. Introduction -- 2. Motivation -- 3. Fundamentals -- 4. Example: The Traveling-Salesman -- 5. Current and Future Applications -- 6. Summary -- 6 Classification: Distance-based Algorithms -- 1. Introduction -- 2. Motivation -- 3. Distance Functions -- 4. Classification Algorithms -- 5. Current Applications -- 6. Summary -- 7 Decision Tree-based Algorithms -- 1. Introduction -- 2. Motivation -- 3. ID3 -- 4. C4.5 -- 5. C5.0 -- 6. CART -- 7. Summary -- 8 Covering (Rule-based) Algorithms -- 1. Introduction -- 2. Motivation -- 3. Classification Rules -- 4. Covering (Rule-based) Algorithms -- 5. Applications of Covering Algorithms -- 6. Summary -- 9 Clustering: An Overview -- 1. Introduction -- 2. Motivation -- 3. The Clustering Process -- 4. Current Applications -- 5. Summary -- 10 Clustering: Hierarchical Algorithms -- 1. Introduction -- 2. Motivation -- 3. Agglomerative Hierarchical Algorithms -- 4. Divisive Hierarchical Algorithms -- 5. Summary -- 11 Clustering: Partitional Algorithms -- 1. Introduction -- 2. Motivation -- 3. Partitional Clustering Algorithms -- 4. Current Applications -- 5. Summary -- 12 Clustering: Large Databases.
1. Introduction -- 2. Motivation -- 3. Requirements for Scalable Clustering -- 4. Major Approaches to Scalable Clustering -- 5. BIRCH -- 6. DBSCAN -- 7. CURE -- 8. Summary -- 13 Clustering: Categorical Attributes -- 1. Introduction -- 2. Motivation -- 3. ROCK Clustering Algorithm -- 4. COOLCAT Clustering Algorithm -- 5. CACTUS Clustering Algorithm -- 6. Summary -- 14 Association Rules: An Overview -- 1. Introduction -- 2. Motivation -- 3. Association Rule Process -- 4. Large Itemset Discovery Algorithms -- 5. Summary -- 15 Association Rules: Parallel and Distributed Algorithms -- 1. Introduction -- 2. Motivation -- 3. Parallel and Distributed Algorithms -- 4. Discussion of Parallel Algorithms -- 5. Summary -- 16 Association Rules: Advanced Techniques and Measures -- 1. Introduction -- 2. Motivation -- 3. Incremental Rules -- 4. Generalized Association Rules -- 5. Quantitative Association Rules -- 6. Correlation Rules -- 7. Measuring the Quality of Association Rules -- 8. Summary -- 17 Spatial Mining: Techniques and Algorithms -- 1. Introduction and Motivation -- 2. Concept Hierarchies and Generalization -- 3. Spatial Rules -- 4. STING -- 5. Spatial Classification -- 6. Spatial Clustering -- 7. Summary -- References -- Index.
Abstract:
The continual explosion of information technology and the need for better data collection and management methods has made data mining an even more relevant topic of study. Books on data mining tend to be either broad and introductory or focus on some very specific technical aspect of the field. This book is a series of seventeen edited "student-authored lectures" which explore in depth the core of data mining (classification, clustering and association rules) by offering overviews that include both analysis and insight. The initial chapters lay a framework of data mining techniques by explaining some of the basics such as applications of Bayes Theorem, similarity measures, and decision trees. Before focusing on the pillars of classification, clustering and association rules, the book also considers alternative candidates such as point estimation and genetic algorithms. The book's discussion of classification includes an introduction to decision tree algorithms, rule-based algorithms (a popular alternative to decision trees) and distance-based algorithms. Five of the lecture-chapters are devoted to the concept of clustering or unsupervised classification. The functionality of hierarchical and partitional clustering algorithms is also covered as well as the efficient and scalable clustering algorithms used in large databases. The concept of association rules in terms of basic algorithms, parallel and distributive algorithms and advanced measures that help determine the value of association rules are discussed. The final chapter discusses algorithms for spatial data mining. Sample Chapter(s). Chapter 1: Point Estimation Algorithms (397 KB). Contents: Point Estimation Algorithms; Applications of Bayes Theorem; Similarity Measures; Decision Trees; Genetic Algorithms; Classification: Distance Based Algorithms; Decision Tree-Based Algorithms; Covering
(Rule-Based) Algorithms; Clustering: An Overview; Clustering Hierarchical Algorithms; Clustering Partitional Algorithms; Clustering: Large Databases; Clustering Categorical Attributes; Association Rules: An Overview; Association Rules: Parallel and Distributed Algorithms; Association Rules: Advanced Techniques and Measures; Spatial Mining: Techniques and Algorithms. Readership: An introductory data mining textbook or a technical data mining book for an upper level undergraduate or graduate level course.
Local Note:
Electronic reproduction. Ann Arbor, Michigan : ProQuest Ebook Central, 2017. Available via World Wide Web. Access may be limited to ProQuest Ebook Central affiliated libraries.
Genre:
Added Author:
Electronic Access:
Click to View