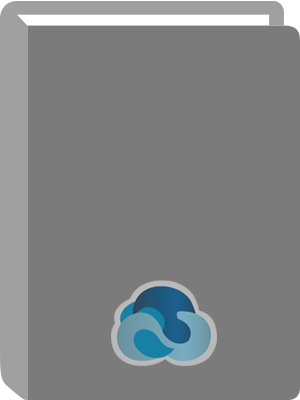
Compositional Data Analysis : Theory and Applications.
Title:
Compositional Data Analysis : Theory and Applications.
Author:
Pawlowsky-Glahn, Vera.
ISBN:
9781119976479
Personal Author:
Edition:
1st ed.
Physical Description:
1 online resource (402 pages)
Contents:
Compositional Data Analysis -- Contents -- Preface -- List of Contributors -- Part I Introduction -- 1 A Short History of Compositional Data Analysis -- 1.1 Introduction -- 1.2 Spurious Correlation -- 1.3 Log and Log-Ratio Transforms -- 1.4 Subcompositional Dependence -- 1.5 alr, clr, ilr: Which Transformation to Choose? -- 1.6 Principles, Perturbations and Back to the Simplex -- 1.7 Biplots and Singular Value Decompositions -- 1.8 Mixtures -- 1.9 Discrete Compositions -- 1.10 Compositional Processes -- 1.11 Structural, Counting and Rounded Zeros -- 1.12 Conclusion -- Acknowledgement -- References -- 2 Basic Concepts and Procedures -- 2.1 Introduction -- 2.2 Election Data and Raw Analysis -- 2.3 The Compositional Alternative -- 2.3.1 Scale Invariance: Vectors with Proportional Positive Components Represent the Same Composition -- 2.3.2 Subcompositional Coherence: Analyses Concerning a Subset of Parts Must Not Depend on Other Non-Involved Parts -- 2.3.3 Permutation Invariance: The Conclusions of a Compositional Analysis Should Not Depend on the Order of the Parts -- 2.4 Geometric Settings -- 2.5 Centre and Variability -- 2.6 Conclusion -- Acknowledgements -- References -- Part II Theory - Statistical Modelling -- 3 The Principle of Working on Coordinates -- 3.1 Introduction -- 3.2 The Role of Coordinates in Statistics -- 3.3 The Simplex -- 3.3.1 Basis of the Simplex -- 3.3.2 Working on Orthonormal Coordinates -- 3.4 Move or Stay in the Simplex -- 3.5 Conclusions -- Acknowledgements -- References -- 4 Dealing with Zeros -- 4.1 Introduction -- 4.2 Rounded Zeros -- 4.2.1 Non-Parametric Replacement of Rounded Zeros -- 4.2.2 Parametric Modified EM Algorithm for Rounded Zeros -- 4.3 Count Zeros -- 4.4 Essential Zeros -- 4.5 Difficulties, Troubles and Challenges -- Acknowledgements -- References -- 5 Robust Statistical Analysis -- 5.1 Introduction.
5.2 Elements of Robust Statistics from a Compositional Point of View -- 5.3 Robust Methods for Compositional Data -- 5.3.1 Multivariate Outlier Detection -- 5.3.2 Principal Component Analysis -- 5.3.3 Discriminant Analysis -- 5.4 Case Studies -- 5.4.1 Multivariate Outlier Detection -- 5.4.2 Principal Component Analysis -- 5.4.3 Discriminant Analysis -- 5.5 Summary -- Acknowledgement -- References -- 6 Geostatistics for Compositions -- 6.1 Introduction -- 6.2 A Brief Summary of Geostatistics -- 6.3 Cokriging of Regionalised Compositions -- 6.4 Structural Analysis of Regionalised Composition -- 6.5 Dealing with Zeros: Replacement Strategies and Simplicial Indicator Cokriging -- 6.6 Application -- 6.6.1 Delimiting the Body: Simplicial Indicator Kriging -- 6.6.2 Interpolating the Oil-Brine-Solid Content -- 6.7 Conclusions -- Acknowledgements -- References -- 7 Compositional VARIMA Time Series -- 7.1 Introduction -- 7.2 The Simplex SD as a Compositional Space -- 7.2.1 Basic Concepts and Notation -- 7.2.2 The Covariance Structure on the Simplex -- 7.3 Compositional Time Series Models -- 7.3.1 C-Stationary Processes -- 7.3.2 C-VARIMA Processes -- 7.4 CTS Modelling: An Example -- 7.4.1 Expenditure Shares in the UK -- 7.4.2 Model Selection -- 7.4.3 Estimation of Parameters -- 7.4.4 Interpretation and Comparison -- 7.5 Discussion -- Acknowledgements -- References -- Appendix -- 8 Compositional Data and Correspondence Analysis -- 8.1 Introduction -- 8.2 Comparative Technical Definitions -- 8.3 Properties and Interpretation of LRA and CA -- 8.4 Application to Fatty Acid Compositional Data -- 8.5 Discussion and Conclusions -- Acknowledgements -- References -- 9 Use of Survey Weights for the Analysis of Compositional Data -- 9.1 Introduction -- 9.2 Elements of Survey Design -- 9.2.1 Randomization -- 9.2.2 Design-Based Estimation.
9.3 Application to Compositional Data -- 9.3.1 Weighted Arithmetic and Geometric Means -- 9.3.2 Closed Arithmetic Mean of Amounts -- 9.3.3 Centred Log-Ratio of the Geometric Mean Composition -- 9.3.4 Closed Geometric Mean Composition -- 9.3.5 Example: Swiss Earnings Structure Survey (SESS) -- 9.4 Discussion -- References -- 10 Notes on the Scaled Dirichlet Distribution -- 10.1 Introduction -- 10.2 Genesis of the Scaled Dirichlet Distribution -- 10.3 Properties of the Scaled Dirichlet Distribution -- 10.3.1 Graphical Comparison -- 10.3.2 Membership in the Exponential Family -- 10.3.3 Measures of Location and Variability -- 10.4 Conclusions -- Acknowledgements -- References -- Part III Theory - Algebra and Calculus -- 11 Elements of Simplicial Linear Algebra and Geometry -- 11.1 Introduction -- 11.2 Elements of Simplicial Geometry -- 11.2.1 n-Part Simplex -- 11.2.2 Vector Space -- 11.2.3 Centred Log-Ratio Representation -- 11.2.4 Metrics -- 11.2.5 Orthonormal Basis and Coordinates -- 11.3 Linear Functions -- 11.3.1 Linear Functions Defined on the Simplex -- 11.3.2 Simplicial Linear Function Defined on a Real Space -- 11.3.3 Simplicial Linear Function Defined on the Simplex -- 11.4 Conclusions -- Acknowledgements -- References -- 12 Calculus of Simplex-Valued Functions -- 12.1 Introduction -- 12.2 Limits, Continuity and Differentiability -- 12.2.1 Limits and Continuity -- 12.2.2 Differentiability -- 12.2.3 Higher Order Derivatives -- 12.3 Integration -- 12.3.1 Antiderivatives. Indefinite Integral -- 12.3.2 Integration of Continuous SV Functions -- 12.4 Conclusions -- Acknowledgements -- References -- 13 Compositional Differential Calculus on the Simplex -- 13.1 Introduction -- 13.2 Vector-Valued Functions on the Simplex -- 13.2.1 Scale-Invariant Vector-Valued Functions on Rn -- 13.2.2 Vector-Valued Functions on Sn -- 13.3 C-Derivatives on the Simplex.
13.3.1 Derivative of a Scale-Invariant Vector-Valued Function on Rn -- 13.3.2 Directional C-Derivatives -- 13.3.3 C-Derivative -- 13.3.4 C-Gradient -- 13.3.5 Critical Points of a C-Differentiable Real-Valued Function on Sn -- 13.4 Example: Experiments with Mixtures -- 13.4.1 Polynomial of Degree One -- 13.4.2 Polynomial of Degree Two -- 13.4.3 Polynomial of Degree One in Logarithms -- 13.4.4 A numerical Example -- 13.5 Discussion -- Acknowledgements -- References -- Part IV Applications -- 14 Proportions, Percentages, PPM: Do the Molecular Biosciences Treat Compositional Data Right? -- 14.1 Introduction -- 14.2 The Omics Imp and Two Bioscience Experiment Paradigms -- 14.3 The Impact of Compositional Constraints in the Omics -- 14.3.1 Univariate Impact of Compositional Constraints -- 14.3.2 Impact of Compositional Constraints on Multivariate Distance Metrics -- 14.4 Impact of Compositional Constraints on Correlation and Covariance -- 14.4.1 Compositional Constraints, Covariance, Correlation and Log-Transformed Data -- 14.4.2 A Simulation Approach to Understanding the Impact Closure -- 14.5 Implications -- 14.5.1 Gathering Information to Infer Absolute Abundance -- 14.5.2 Analysing Compositional Omics Data Appropriately -- Acknowledgements -- References -- 15 Hardy-Weinberg Equilibrium: A Nonparametric Compositional Approach -- 15.1 Introduction -- 15.2 Genetic Data Sets -- 15.3 Classical Tests for HWE -- 15.4 A Compositional Approach -- 15.5 Example -- 15.6 Conclusion and Discussion -- Acknowledgements -- References -- 16 Compositional Analysis in Behavioural and Evolutionary Ecology -- 16.1 Introduction -- 16.2 CODA in Population Genetics -- 16.3 CODA in Habitat Choice -- 16.4 Multiple Choice and Individual Variation in Preferences -- 16.5 Ecological Specialization -- 16.6 Time Budgets: More on Specialization -- 16.7 Conclusions -- Acknowledgements.
References -- 17 Flying in Compositional Morphospaces: Evolution of Limb Proportions in Flying Vertebrates -- 17.1 Introduction -- 17.2 Flying Vertebrates - General Anatomical and Functional Characteristics -- 17.3 Materials -- 17.4 Methods -- 17.5 Aitchison Distance Disparity Metrics -- 17.5.1 Intragroup Aitchison Distance -- 17.5.2 Intergroup Aitchison Distance -- 17.6 Statistical Tests -- 17.7 Biplots -- 17.7.1 Chiroptera -- 17.7.2 Pterosauria -- 17.8 Balances -- 17.9 Size Effect -- 17.10 Final Remarks -- 17.10.1 All Groups -- 17.10.2 Aves -- 17.10.3 Pterosauria -- 17.10.4 Chiroptera -- Acknowledgements -- References -- 18 Natural Laws Governing the Distribution of the Elements in Geochemistry: The Role of the Log-Ratio Approach -- 18.1 Introduction -- 18.2 Geochemical Processes and Log-Ratio Approach -- 18.3 Log-Ratio Approach and Water Chemistry -- 18.4 Log-Ratio Approach and Volcanic Gas Chemistry -- 18.5 Log-Ratio Approach and Subducting Sediment Composition -- 18.6 Conclusions -- Acknowledgements -- References -- 19 Compositional Data Analysis in Planetology: The Surfaces of Mars and Mercury -- 19.1 Introduction -- 19.1.1 Mars -- 19.1.2 Mercury -- 19.1.3 Analysis of Surface Composition -- 19.2 Compositional Analysis of Mars' Surface -- 19.3 Compositional Analysis of Mercury's Surface -- 19.4 Conclusion -- Acknowledgement -- References -- 20 Spectral Analysis of Compositional Data in Cyclostratigraphy -- 20.1 Introduction -- 20.2 The Method -- 20.3 Case Study -- 20.4 Discussion -- 20.5 Conclusions -- Acknowledgement -- References -- 21 Multivariate Geochemical Data Analysis in Physical Geography -- 21.1 Introduction -- 21.2 Context -- 21.3 Data -- 21.4 Analysis -- 21.5 Discussion -- 21.6 Conclusion -- Acknowledgement -- References -- 22 Combining Isotopic and Compositional Data: A Discrimination of Regions Prone to Nitrate Pollution.
22.1 Introduction.
Abstract:
It is difficult to imagine that the statistical analysis of compositional data has been a major issue of concern for more than 100 years. It is even more difficult to realize that so many statisticians and users of statistics are unaware of the particular problems affecting compositional data, as well as their solutions. The issue of ``spurious correlation'', as the situation was phrased by Karl Pearson back in 1897, affects all data that measures parts of some whole, such as percentages, proportions, ppm and ppb. Such measurements are present in all fields of science, ranging from geology, biology, environmental sciences, forensic sciences, medicine and hydrology. This book presents the history and development of compositional data analysis along with Aitchison's log-ratio approach. Compositional Data Analysis describes the state of the art both in theoretical fields as well as applications in the different fields of science. Key Features: Reflects the state-of-the-art in compositional data analysis. Gives an overview of the historical development of compositional data analysis, as well as basic concepts and procedures. Looks at advances in algebra and calculus on the simplex. Presents applications in different fields of science, including, genomics, ecology, biology, geochemistry, planetology, chemistry and economics. Explores connections to correspondence analysis and the Dirichlet distribution. Presents a summary of three available software packages for compositional data analysis. Supported by an accompanying website featuring R code. Applied scientists working on compositional data analysis in any field of science, both in academia and professionals will benefit from this book, along with graduate students in any field of science working with compositional data.
Local Note:
Electronic reproduction. Ann Arbor, Michigan : ProQuest Ebook Central, 2017. Available via World Wide Web. Access may be limited to ProQuest Ebook Central affiliated libraries.
Genre:
Added Author:
Electronic Access:
Click to View