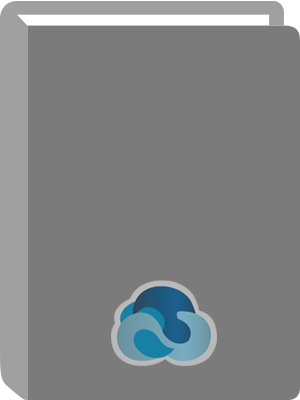
Regression Analysis of Count Data.
Title:
Regression Analysis of Count Data.
Author:
Cameron, A. Colin.
ISBN:
9781139145886
Personal Author:
Physical Description:
1 online resource (434 pages)
Series:
Econometric Society Monographs ; v.30
Econometric Society Monographs
Contents:
Cover -- Half-title -- Series-title -- Title -- Copyright -- Dedication -- Contents -- List of Figures -- List of Tables -- Preface -- CHAPTER 1 Introduction -- 1.1 Poisson Distribution -- 1.1.1 Poisson as the "Law of Rare Events" -- 1.1.2 Poisson Process -- 1.1.3 Waiting Time Distributions -- 1.1.4 Binomial Stopped by the Poisson -- 1.2 Poisson Regression -- 1.2.1 Counts Derived from a Point Process -- 1.2.2 Counts Derived from Continuous Data -- 1.2.3 Regression Specification -- 1.3 Examples -- 1.3.1 Health Services -- 1.3.2 Patents -- 1.3.3 Recreational Demand -- 1.3.4 Takeover Bids -- 1.3.5 Bank Failures -- 1.3.6 Accident Insurance -- 1.3.7 Credit Rating -- 1.3.8 Presidential Appointments -- 1.3.9 Criminal Careers -- 1.3.10 Doctoral Publications -- 1.3.11 Manufacturing Defects -- 1.4 Overview of Major Issues -- 1.5 Bibliographic Notes -- CHAPTER 2 Model Specification and Estimation -- 2.1 Introduction -- 2.2 Example and Definitions -- 2.2.1 Example -- 2.2.2 Definitions -- 2.3 Likelihood-Based Models -- 2.3.1 Regularity Conditions -- 2.3.2 Maximum Likelihood -- 2.3.3 Profile Likelihood -- 2.3.4 Misspecified Density -- 2.4 Generalized Linear Models -- 2.4.1 Weighted Linear Least Squares -- 2.4.2 Linear Exponential Family Models -- 2.4.3 LEF with Nuisance Parameter -- 2.4.4 Generalized Linear Models -- 2.4.5 Extensions -- 2.5 Moment-Based Models -- 2.5.1 Estimating Equations -- 2.5.2 Generalized Methods of Moments -- 2.5.3 Optimal GMM -- 2.5.4 Sequential Two-Step Estimators -- 2.6 Testing -- 2.6.1 Likelihood-Based Models -- 2.6.2 General Models -- 2.6.3 Conditional Moment Tests -- 2.7 Derivations -- 2.7.1 General Framework -- 2.7.2 Likelihood-Based Models -- 2.7.3 Generalized Linear Models -- 2.7.4 Moment-Based Models -- 2.7.5 Conditional Moment Tests -- 2.8 Bibliographic Notes -- 2.9 Exercises -- CHAPTER 3 Basic Count Regression.
3.1 Introduction -- 3.2 Poisson MLE, PMLE, and GLM -- 3.2.1 Poisson MLE -- 3.2.2 NB1 and NB2 Variance Functions -- 3.2.3 Poisson PMLE -- Poisson PMLE with Poisson Variance Function -- Poisson PMLE with NB1 Variance Function -- Poisson PMLE with NB2 Variance Function -- Poisson PMLE with Unspecified Variance Function -- 3.2.4 Poisson GLM -- 3.2.5 Poisson EE -- 3.2.6 Example: Doctor Visits -- 3.3 Negative Binomial MLE and QGPMLE -- 3.3.1 NB2 Model and MLE -- 3.3.2 NB2 Model and QGPMLE -- 3.3.3 NB1 Model and MLE -- 3.3.4 Discussion -- 3.4 Overdispersion Tests -- 3.5 Use of Regression Results -- 3.5.1 Interpretation of Coefficients -- 3.5.2 Prediction -- 3.6 Ordered and Other Discrete-Choice Models -- 3.6.1 Binary-Choice Models -- 3.6.2 Ordered Probit -- 3.7 Other Models -- 3.7.1 OLS without Transformation -- 3.7.2 OLS with Transformation -- 3.7.3 Nonlinear Least Squares -- 3.7.4 Exponential Duration Model -- 3.8 Iteratively Reweighted Least Squares -- 3.9 Bibliographic Notes -- 3.10 Exercises -- CHAPTER 4 Generalized Count Regression -- 4.1 Introduction -- 4.2 Mixture Models for Unobserved Heterogeneity -- 4.2.1 Unobserved Heterogeneity and Overdispersion -- 4.2.2 Negative Binomial Model -- 4.2.3 Other Characterizations of NB -- 4.2.4 General Mixture Results -- 4.2.5 Identification -- 4.2.6 Consequences of Misspecified Heterogeneity -- 4.3 Models Based on Waiting-Time Distributions -- 4.3.1 True and Apparent Contagion -- 4.3.2 Renewal Process -- 4.3.3 Waiting-Time Distribution -- 4.3.4 Gamma Waiting Times -- 4.3.5 Dependence and Dispersion -- 4.4 Katz, Double Poisson, and Generalized Poisson -- 4.4.1 The Katz System -- 4.4.2 Example: Doctor Visits -- 4.4.3 Double Poisson Model -- 4.4.4 Neyman's Contagious Distributions -- 4.4.5 Consul's Generalized Poisson -- 4.5 Truncated Counts -- 4.5.1 Standard Truncated Models -- 4.5.2 Maximum Likelihood Estimation.
4.6 Censored Counts -- 4.7 Hurdle and Zero-Inflated Models -- 4.7.1 With Zeros and Hurdle Models -- 4.7.2 Zero-Inflated Counts -- 4.7.3 Example: Hurdles and Two-Part Decisionmaking -- 4.8 Finite Mixtures and Latent Class Analysis -- 4.8.1 Finite Mixtures -- 4.8.2 Nonparametric Maximum Likelihood -- 4.8.3 Latent Class Analysis -- 4.8.4 Estimation -- 4.8.5 Inference on C -- 4.9 Estimation by Simulation -- 4.10 Derivations -- 4.11 Bibliographic Notes -- 4.12 Exercises -- CHAPTER 5 Model Evaluation and Testing -- 5.1 Introduction -- 5.2 Residual Analysis -- 5.2.1 Pearson, Deviance, and Anscombe Residuals -- 5.2.2 Generalized Residuals -- 5.2.3 Using Residuals -- 5.2.4 Small Sample Corrections and Influential Observations -- 5.2.5 Example: Takeover Bids -- 5.3 Goodness of Fit -- 5.3.1 Pearson Statistic -- 5.3.2 Deviance Statistic -- 5.3.3 Pseudo R-Squared Measures -- 5.3.4 Chi-Square Goodness of Fit Test -- 5.4 Hypothesis Tests -- 5.4.1 LM Test for Overdispersion against Katz System -- 5.4.2 Auxiliary Regressions for LM Test -- 5.4.3 LM Test against Local Alternatives -- 5.5 Inference with Finite Sample Corrections -- 5.5.1 Bootstrap -- 5.5.2 Other Corrections -- 5.6 Conditional Moment Specification Tests -- 5.6.1 Introduction -- 5.6.2 Regression-Based Tests for Overdispersion -- 5.6.3 Regression-Based CM Tests -- 5.6.4 Orthogonal Polynomial Tests -- 5.6.5 Information Matrix Tests -- 5.6.6 Hausman Tests -- 5.7 Discriminating among Nonnested Models -- 5.7.1 Information Criteria -- 5.7.2 Tests of Nonnested Models -- 5.8 Derivations -- 5.8.1 Test of Poisson Against Katz System -- 5.8.2 LM Test Against Local Alternatives -- 5.9 Bibliographic Notes -- 5.10 Exercises -- CHAPTER 6 Empirical Illustrations -- 6.1 Introduction -- 6.2 Background -- 6.2.1 Fully Parametric Estimation -- 6.2.2 Repeated Events -- 6.3 Analysis of Demand for Health Services.
6.3.1 Health Service Data -- 6.3.2 Demand for Medical Care -- 6.3.3 Competing Models -- 6.3.4 Is There a Mixture? -- 6.3.5 Model Comparison and Selection -- 6.3.6 Evaluation of Fitted Models -- 6.3.7 Assessing the Preferred Model -- 6.3.8 Interpreting the Coefficients -- 6.3.9 Economic Significance -- 6.4 Analysis of Recreational Trips -- 6.4.1 Recreational Trips Data -- 6.4.2 Initial Specifications -- 6.4.3 Modified Poisson Models -- 6.4.4 Economic Implications -- 6.5 LR Test: A Digression -- 6.5.1 Simulation Analysis of Model Selection Criteria -- Simulation Design -- Simulation Outcomes -- 6.5.2 Bootstrapping the LR Test -- 6.6 Concluding Remarks -- 6.7 Bibliographic Notes -- 6.8 Exercises -- CHAPTER 7 Time Series Data -- 7.1 Introduction -- 7.2 Models for Time Series Data -- 7.2.1 Linear Models -- 7.2.2 Count Models -- 7.3 Static Regression -- 7.3.1 Estimation -- 7.3.2 Tests of Serial Correlation -- 7.3.3 Trends and Seasonality -- 7.3.4 Example: Strikes -- 7.4 Integer-Valued ARMA Models -- 7.4.1 Pure Time Series Models -- 7.4.2 Regression Models -- 7.5 Autoregressive Models -- 7.6 Serially Correlated Error Models -- 7.7 State-Space Models -- 7.7.1 Conjugate Distributed Mean -- 7.7.2 Normally Distributed Parameters -- 7.8 Hidden Markov Models -- 7.9 Discrete ARMA Models -- 7.10 Application -- 7.11 Derivations -- 7.12 Bibliographic Notes -- 7.13 Exercises -- CHAPTER 8 Multivariate Data -- 8.1 Introduction -- 8.2 Characterizing Dependence -- 8.2.1 Some Approaches -- 8.2.2 Example -- 8.3 Parametric Models -- 8.3.1 Bivariate Poisson -- 8.3.2 Other Fully Parametric Models -- 8.4 Moment-Based Estimation -- 8.4.1 Bivariate Poisson with Heterogeneity -- 8.4.2 Seemingly Unrelated Regressions -- 8.5 Orthogonal Polynomial Series Expansions -- 8.5.1 Definitions and Conditions -- 8.5.2 Univariate Expansion -- 8.5.3 Multivariate Expansions.
8.5.4 Tests of Independence -- 8.5.5 Example: Medical Services -- 8.6 Mixed Multivariate Models -- 8.6.1 Discrete Choice and Counts -- 8.6.2 Counts and Continuous Variables -- 8.7 Derivations -- 8.8 Bibliographic Notes -- CHAPTER 9 Longitudinal Data -- 9.1 Introduction -- 9.2 Models for Longitudinal Data -- 9.2.1 Linear Models -- 9.2.2 Count Models -- 9.3 Fixed Effects Models -- 9.3.1 Maximum Likelihood -- 9.3.2 Conditional Maximum Likelihood -- 9.3.3 Moment-Based Methods -- 9.3.4 Example: Patents -- 9.4 Random Effects Models -- 9.4.1 Conjugate-Distributed Random Effects -- 9.4.2 Gaussian Random Effects -- 9.4.3 Moment-Based Methods -- 9.5 Discussion -- 9.6 Specification Tests -- 9.6.1 Fixed Versus Random Effects -- 9.6.2 Tests for Serial Correlation -- 9.7 Dynamic and Transition Models -- 9.7.1 Some Approaches -- 9.7.2 Fixed Effects Models -- 9.8 Derivations -- 9.8.1 Conditional Density for Poisson Fixed Effects -- 9.8.2 Density for Poisson with Gamma Random Effects -- 9.9 Bibliographic Notes -- 9.10 Exercises -- CHAPTER 10 Measurement Errors -- 10.1 Introduction -- 10.2 Measurement Errors in Exposure -- 10.2.1 Correctly Observed Exposure -- 10.2.2 Multiplicative Error in Exposure -- 10.2.3 Proxy Variables for Exposure -- 10.3 Measurement Errors in Regressors -- 10.3.1 Additive Measurement Errors -- 10.3.2 Multiplicative Error in Regressors -- 10.4 Measurement Errors in Counts -- 10.4.1 Additive Measurement Errors in Counts -- 10.4.2 Misclassified Counts -- 10.4.3 Outlying Counts -- 10.5 Underreported Counts -- 10.5.1 Mechanism and Examples -- 10.5.2 Dependence Between Events and Recording -- 10.5.3 Distribution of Recorded Events -- Poisson Distribution -- Negative Binomial Distribution -- 10.5.4 Underrecorded-Count Regressions under Independence -- Poisson Case -- Example: Safety Violations -- Negative Binomial Case.
10.5.5 Underreported-Count Regressions under Dependence.
Abstract:
This analysis provides a comprehensive account of models and methods to interpret frequency data.
Local Note:
Electronic reproduction. Ann Arbor, Michigan : ProQuest Ebook Central, 2017. Available via World Wide Web. Access may be limited to ProQuest Ebook Central affiliated libraries.
Genre:
Added Author:
Electronic Access:
Click to View