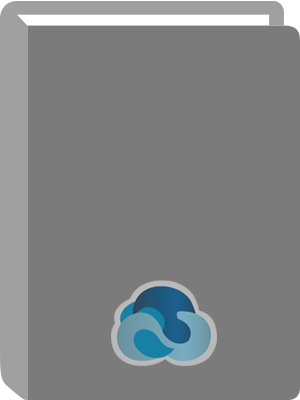
Graph-Related Optimization and Decision Theory.
Title:
Graph-Related Optimization and Decision Theory.
Author:
Krichen, Saoussen.
ISBN:
9781118984253
Personal Author:
Edition:
1st ed.
Physical Description:
1 online resource (186 pages)
Contents:
Cover page -- Half-Title page -- Title page -- Copyright page -- Contents -- List of Tables -- List of Figures -- List of Algorithms -- Introduction -- 1: Basic Concepts in Optimization and Graph Theory -- 1.1. Introduction -- 1.2. Notation -- 1.3. Problem structure and variants -- 1.4. Features of an optimization problem -- 1.5. A didactic example -- 1.6. Basic concepts in graph theory -- 1.6.1. Degree of a graph -- 1.6.2. Matrix representation of a graph -- 1.6.3. Connected graphs -- 1.6.4. Itineraries in a graph -- 1.6.5. Tree graphs -- 1.6.6. The bipartite graphs -- 1.7. Conclusion -- 2: Knapsack Problems -- 2.1. Introduction -- 2.2. Graph modeling of the knapsack problem -- 2.3. Notation -- 2.4. 0-1 knapsack problem -- 2.5. An example -- 2.6. Multiple knapsack problem -- 2.6.1. Mathematical model -- 2.6.2. An example -- 2.7. Multidimensional knapsack problem -- 2.7.1. Mathematical model -- 2.7.2. An example -- 2.8. Quadratic knapsack problem -- 2.8.1. Mathematical model -- 2.8.2. An example -- 2.9. Quadratic multidimensional knapsack problem -- 2.9.1. Mathematical model -- 2.9.2. An example -- 2.10. Solution approaches for knapsack problems -- 2.10.1. The greedy algorithm -- 2.10.2. A genetic algorithm for the KP -- 2.10.2.1. Solution encoding -- 2.10.2.2. Crossover -- 2.10.2.3. Mutation -- 2.11. Conclusion -- 3: Packing Problems -- 3.1. Introduction -- 3.2. Graph modeling of the bin packing problem -- 3.3. Notation -- 3.4. Basic bin packing problem -- 3.4.1. Mathematical modeling of the BPP -- 3.4.2. An example -- 3.5. Variable cost and size BPP -- 3.5.1. Mathematical model -- 3.5.2. An example -- 3.6. Vector BPP -- 3.6.1. Mathematical model -- 3.6.2. An example -- 3.7. BPP with conflicts -- 3.7.1. Mathematical model -- 3.7.2. An example -- 3.8. Solution approaches for the BPP -- 3.8.1. The next-fit strategy -- 3.8.2. The first-fit strategy.
3.8.3. The best-fit strategy -- 3.8.4. The minimum bin slack -- 3.8.5. The minimum bin slack' -- 3.8.6. The least loaded heuristic -- 3.8.7. A genetic algorithm for the bin packing problem -- 3.8.7.1. Solution encoding -- 3.8.7.2. Crossover -- 3.8.7.3. Mutation -- 3.9. Conclusion -- 4: Assignment Problem -- 4.1. Introduction -- 4.2. Graph modeling of the assignment problem -- 4.3. Notation -- 4.4. Mathematical formulation of the basic AP -- 4.4.1. An example -- 4.5. Generalized assignment problem -- 4.5.1. An example -- 4.6. The generalized multiassignment problem -- 4.6.1. An example -- 4.7. Weighted assignment problem -- 4.8. Generalized quadratic assignment problem -- 4.9. The bottleneck GAP -- 4.10. The multilevel GAP -- 4.11. The elastic GAP -- 4.12. The multiresource GAP -- 4.13. Solution approaches for solving the AP -- 4.13.1. A greedy algorithm for the AP -- 4.13.2. A genetic algorithm for the AP -- 4.13.2.1. Solution encoding -- 4.13.2.2. Crossover -- 4.13.2.3. Mutation -- 4.14. Conclusion -- 5: The Resource Constrained Project Scheduling Problem -- 5.1. Introduction -- 5.2. Graph modeling of the RCPSP -- 5.3. Notation -- 5.4. Single-mode RCPSP -- 5.4.1. Mathematical modeling of the SM-RCPSP -- 5.4.2. An example of an SM-RCPSP -- 5.5. Multimode RCPSP -- 5.6. RCPSP with time windows -- 5.7. Solution approaches for solving the RCPSP -- 5.7.1. A greedy algorithm for the RCPSP -- 5.7.2. A genetic algorithm for the RCPSP -- 5.7.2.1. Chromosome encoding -- 5.7.2.2. Selection -- 5.7.2.3. Crossover -- 5.7.2.4. Mutation -- 5.8. Conclusion -- 6: Spanning Tree Problems -- 6.1. Introduction -- 6.2. Minimum spanning tree problem -- 6.2.1. Notation -- 6.2.2. Mathematical formulation -- 6.2.3. Algorithms for the MST problem -- 6.2.3.1. The Kruskal algorithm -- 6.2.3.2. The Prim algorithm -- 6.3. Generalized minimum spanning tree problem -- 6.3.1. Notation.
6.3.2. Mathematical formulation -- 6.3.3. Greedy approaches for the GMST problem -- 6.3.4. Genetic algorithm for the GMST problem -- 6.3.4.1. Encoding -- 6.3.4.2. Fitness -- 6.3.4.3. Crossover -- 6.3.4.4. Mutation -- 6.3.4.5. Parameters -- 6.4. k-cardinality tree problem KCT -- 6.4.1. Problem definition -- 6.4.2. An example -- 6.4.3. Notation -- 6.4.4. Mathematical formulation -- 6.4.5. Greedy approaches for the k-cardinality tree problem -- 6.4.5.1. The k-Prim approach and the dual-greedy algorithm -- 6.4.6. Minimum path approach -- 6.4.7. A genetic approach for the k-cardinality problem -- 6.5. The capacitated minimum spanning tree problem -- 6.5.1. Problem definition -- 6.5.2. Notation -- 6.5.3. An example -- 6.5.4. Solution approaches for the CMST problem -- 6.5.4.1. A greedy approach -- 6.5.4.2. Genetic algorithm for the CMST problem -- 6.5.4.2.1. Encoding -- 6.5.4.2.2. Fitness -- 6.5.4.2.3. Mutation -- 6.5.4.2.4. Parameters -- 6.6. Conclusion -- 7: Steiner Problems -- 7.1. Introduction -- 7.2. The Steiner tree problem -- 7.2.1. Problem definition -- 7.2.2. Problem formulation -- 7.2.3. Constructive heuristics for the Steiner tree problem -- 7.2.3.1. Shortest paths heuristic -- 7.2.3.2. Shortest distance heuristic -- 7.2.3.3. Arbitrary node insertion -- 7.2.3.4. Cheapest node insertion -- 7.2.3.5. Cheapest node insertion for all roots -- 7.3. The price collecting Steiner tree problem -- 7.3.1. Problem definition -- 7.3.2. Example -- 7.3.3. Mathematical formulation -- 7.3.4. A greedy approach to solve the PCSTP -- 7.3.5. A genetic algorithm for the PCSTP -- 7.3.5.1. Encoding -- 7.3.5.2. Fitness -- 7.3.5.3. Crossover -- 7.4. Conclusion -- 8: A DSS Design for Optimization Problems -- 8.1. Introduction -- 8.2. Definition of a DSS -- 8.3. Taxonomy of a DSS -- 8.4. Architecture and design of a DSS -- 8.4.1. Architecture of a DSS -- 8.4.2. DSS design.
8.5. A DSS for the knapsack problem -- 8.6. A DSS for the DCVRP -- 8.6.1. Statement and modeling of the CVRP -- 8.6.2. Notation -- 8.6.3. Mathematical formulation of the DCVRP -- 8.6.4. DCVRP-DSS interfaces -- 8.6.5. A real application: the case of Tunisia -- 8.7. Conclusion -- Conclusion -- Glossary -- Bibliography -- Index.
Abstract:
Constrained optimization is a challenging branch of operations research that aims to create a model which has a wide range of applications in the supply chain, telecommunications and medical fields. As the problem structure is split into two main components, the objective is to accomplish the feasible set framed by the system constraints. The aim of this book is expose optimization problems that can be expressed as graphs, by detailing, for each studied problem, the set of nodes and the set of edges. This graph modeling is an incentive for designing a platform that integrates all optimization components in order to output the best solution regarding the parameters' tuning. The authors propose in their analysis, for optimization problems, to provide their graphical modeling and mathematical formulation and expose some of their variants. As a solution approaches, an optimizer can be the most promising direction for limited-size instances. For large problem instances, approximate algorithms are the most appropriate way for generating high quality solutions. The authors thus propose, for each studied problem, a greedy algorithm as a problem-specific heuristic and a genetic algorithm as a metaheuristic.
Local Note:
Electronic reproduction. Ann Arbor, Michigan : ProQuest Ebook Central, 2017. Available via World Wide Web. Access may be limited to ProQuest Ebook Central affiliated libraries.
Genre:
Added Author:
Electronic Access:
Click to View