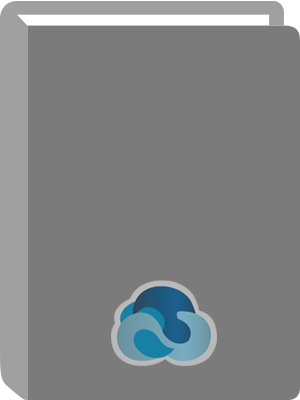
Meta-Analysis : A Structural Equation Modeling Approach.
Title:
Meta-Analysis : A Structural Equation Modeling Approach.
Author:
Cheung, Mike W.-L.
ISBN:
9781118957837
Personal Author:
Edition:
1st ed.
Physical Description:
1 online resource (403 pages)
Contents:
Cover -- Title Page -- Copyright -- Contents -- Preface -- Acknowledgments -- List of abbreviations -- List of figures -- List of tables -- Chapter 1 Introduction -- 1.1 What is meta-analysis? -- 1.2 What is structural equation modeling? -- 1.3 Reasons for writing a book on meta-analysis and structural equation modeling -- 1.3.1 Benefits to users of structural equation modeling and meta-analysis -- 1.4 Outline of the following chapters -- 1.4.1 Computer examples and data sets used in this book -- 1.5 Concluding remarks and further readings -- References -- Chapter 2 Brief review of structural equation modeling -- 2.1 Introduction -- 2.2 Model specification -- 2.2.1 Equations -- 2.2.2 Path diagram -- 2.2.3 Matrix representation -- 2.3 Common structural equation models -- 2.3.1 Path analysis -- 2.3.2 Confirmatory factor analysis -- 2.3.3 Structural equation model -- 2.3.4 Latent growth model -- 2.3.5 Multiple-group analysis -- 2.4 Estimation methods, test statistics, and goodness-of-fit indices -- 2.4.1 Maximum likelihood estimation -- 2.4.2 Weighted least squares -- 2.4.3 Multiple-group analysis -- 2.4.4 Likelihood ratio test and Wald test -- 2.4.5 Confidence intervals on parameter estimates -- 2.4.6 Test statistics versus goodness-of-fit indices -- 2.5 Extensions on structural equation modeling -- 2.5.1 Phantom variables -- 2.5.2 Definition variables -- 2.5.3 Full information maximum likelihood estimation -- 2.6 Concluding remarks and further readings -- References -- Chapter 3 Computing effect sizes for meta-analysis -- 3.1 Introduction -- 3.2 Effect sizes for univariate meta-analysis -- 3.2.1 Mean differences -- 3.2.2 Correlation coefficient and its Fisher's z transformation -- 3.2.3 Binary variables -- 3.3 Effect sizes for multivariate meta-analysis -- 3.3.1 Mean differences.
3.3.2 Correlation matrix and its Fisher's z transformation -- 3.3.3 Odds ratio -- 3.4 General approach to estimating the sampling variances and covariances -- 3.4.1 Delta method -- 3.4.2 Computation with structural equation modeling -- 3.5 Illustrations Using R -- 3.5.1 Repeated measures -- 3.5.2 Multiple treatment studies -- 3.5.3 Multiple-endpoint studies -- 3.5.4 Multiple treatment with multiple-endpoint studies -- 3.5.5 Correlation matrix -- 3.6 Concluding remarks and further readings -- References -- Chapter 4 Univariate meta-analysis -- 4.1 Introduction -- 4.2 Fixed-effects model -- 4.2.1 Estimation and hypotheses testing -- 4.2.2 Testing the homogeneity of effect sizes -- 4.2.3 Treating the sampling variance as known versus as estimated -- 4.3 Random-effects model -- 4.3.1 Estimation and hypothesis testing -- 4.3.2 Testing the variance component -- 4.3.3 Quantifying the degree of the heterogeneity of effect sizes -- 4.4 Comparisons between the fixed- and the random-effects models -- 4.4.1 Conceptual differences -- 4.4.2 Statistical differences -- 4.5 Mixed-effects model -- 4.5.1 Estimation and hypotheses testing -- 4.5.2 Explained variance -- 4.5.3 A cautionary note -- 4.6 Structural equation modeling approach -- 4.6.1 Fixed-effects model -- 4.6.2 Random-effects model -- 4.6.3 Mixed-effects model -- 4.7 Illustrations using R -- 4.7.1 Odds ratio of atrial fibrillation between bisphosphonate and non-bisphosphonate users -- 4.7.2 Correlation between organizational commitment and salesperson job performance -- 4.8 Concluding remarks and further readings -- References -- Chapter 5 Multivariate meta-analysis -- 5.1 Introduction -- 5.1.1 Types of dependence -- 5.1.2 Univariate meta-analysis versus multivariate meta-analysis -- 5.2 Fixed-effects model -- 5.2.1 Testing the homogeneity of effect sizes.
5.2.2 Estimation and hypotheses testing -- 5.3 Random-effects model -- 5.3.1 Structure of the variance component of random effects -- 5.3.2 Nonnegative definite of the variance component of random effects -- 5.3.3 Estimation and hypotheses testing -- 5.3.4 Quantifying the degree of heterogeneity of effect sizes -- 5.3.5 When the sampling covariances are not known -- 5.4 Mixed-effects model -- 5.4.1 Explained variance -- 5.5 Structural equation modeling approach -- 5.5.1 Fixed-effects model -- 5.5.2 Random-effects model -- 5.5.3 Mixed-effects model -- 5.6 Extensions: mediation and moderation models on the effect sizes -- 5.6.1 Regression model -- 5.6.2 Mediating model -- 5.6.3 Moderating model -- 5.7 Illustrations using R -- 5.7.1 BCG vaccine for preventing tuberculosis -- 5.7.2 Standardized mean differences between males and females on life satisfaction and life control -- 5.7.3 Mediation and moderation models -- 5.8 Concluding remarks and further readings -- References -- Chapter 6 Three-level meta-analysis -- 6.1 Introduction -- 6.1.1 Examples of dependent effect sizes with unknown degree of dependence -- 6.1.2 Common methods to handling dependent effect sizes -- 6.2 Three-level model -- 6.2.1 Random-effects model -- 6.2.2 Mixed-effects model -- 6.3 Structural equation modeling approach -- 6.3.1 Two representations of the same model -- 6.3.2 Random-effects model -- 6.3.3 Mixed-effects model -- 6.4 Relationship between the multivariate and the three-level meta-analyses -- 6.4.1 Three-level meta-analysis as a special case of the multivariate meta-analysis -- 6.4.2 Approximating a multivariate meta-analysis with a three-level meta-analysis -- 6.4.3 Three-level multivariate meta-analysis -- 6.5 Illustrations using R -- 6.5.1 Inspecting the data -- 6.5.2 Fitting a random-effects model.
6.5.3 Obtaining the likelihood-based confidence interval -- 6.5.4 Testing τ(3)2 = 0 -- 6.5.5 Testing τ(2)2 = 0 -- 6.5.6 Testing τ(2)2 = τ(3)2 -- 6.5.7 Testing types of proposals (grant versus fellowship) -- 6.5.8 Testing the effect of the year of application -- 6.5.9 Testing the country effect -- 6.6 Concluding remarks and further readings -- References -- Chapter 7 Meta-analytic structural equation modeling -- 7.1 Introduction -- 7.1.1 Meta-analytic structural equation modeling as a possible solution for conflicting research findings -- 7.1.2 Basic steps for conducting a meta-analytic structural equation modeling -- 7.2 Conventional approaches -- 7.2.1 Univariate approaches -- 7.2.2 Generalized least squares approach -- 7.3 Two-stage structural equation modeling: fixed-effects models -- 7.3.1 Stage 1 of the analysis: pooling correlation matrices -- 7.3.2 Stage 2 of the analysis: fitting structural models -- 7.3.3 Subgroup analysis -- 7.4 Two-stage structural equation modeling: random-effects models -- 7.4.1 Stage 1 of the analysis: pooling correlation matrices -- 7.4.2 Stage 2 of the analysis: fitting structural models -- 7.5 Related issues -- 7.5.1 Multiple-group structural equation modeling versus meta-analytic structural equation modeling -- 7.5.2 Fixed-effects model: two-stage structural equation modeling versus generalized least squares -- 7.5.3 Alternative random-effects models -- 7.5.4 Maximum likelihood estimation versus restricted (or residual) maximum likelihood estimation -- 7.5.5 Correlation coefficient versus Fisher's z score -- 7.5.6 Correction for unreliability -- 7.6 Illustrations using R -- 7.6.1 A higher-order confirmatory factor analytic model for the Big Five model -- 7.6.2 A regression model on SAT (Math).
7.6.3 A path model for cognitive ability to supervisor rating -- 7.7 Concluding remarks and further readings -- References -- Chapter 8 Advanced topics in SEM-based meta-analysis -- 8.1 Restricted (or residual) maximum likelihood estimation -- 8.1.1 Reasons for and against the maximum likelihood estimation -- 8.1.2 Applying the restricted (or residual) maximum likelihood estimation in SEM-based meta-analysis -- 8.1.3 Implementation in structural equation modeling -- 8.2 Missing values in the moderators -- 8.2.1 Types of missing mechanisms -- 8.2.2 Common methods to handling missing data -- 8.2.3 Maximum likelihood estimation -- 8.3 Illustrations using R -- 8.3.1 Restricted (or residual) maximum likelihood estimation -- 8.3.2 Missing values in the moderators -- 8.4 Concluding remarks and further readings -- References -- Chapter 9 Conducting meta-analysis with Mplus -- 9.1 Introduction -- 9.2 Univariate meta-analysis -- 9.2.1 Fixed-effects model -- 9.2.2 Random-effects model -- 9.2.3 Mixed-effects model -- 9.2.4 Handling missing values in moderators -- 9.3 Multivariate meta-analysis -- 9.3.1 Fixed-effects model -- 9.3.2 Random-effects model -- 9.3.3 Mixed-effects model -- 9.3.4 Mediation and moderation models on the effect sizes -- 9.4 Three-level meta-analysis -- 9.4.1 Random-effects model -- 9.4.2 Mixed-effects model -- 9.5 Concluding remarks and further readings -- References -- Appendix A A brief introduction to R, OpenMx, and metaSEM packages -- A.1 R -- A.2 OpenMx -- A.3 metaSEM -- References -- Index -- EULA.
Abstract:
Presents a novel approach to conducting meta-analysis using structural equation modeling. Structural equation modeling (SEM) and meta-analysis are two powerful statistical methods in the educational, social, behavioral, and medical sciences. They are often treated as two unrelated topics in the literature. This book presents a unified framework on analyzing meta-analytic data within the SEM framework, and illustrates how to conduct meta-analysis using the metaSEM package in the R statistical environment. Meta-Analysis: A Structural Equation Modeling Approach begins by introducing the importance of SEM and meta-analysis in answering research questions. Key ideas in meta-analysis and SEM are briefly reviewed, and various meta-analytic models are then introduced and linked to the SEM framework. Fixed-, random-, and mixed-effects models in univariate and multivariate meta-analyses, three-level meta-analysis, and meta-analytic structural equation modeling, are introduced. Advanced topics, such as using restricted maximum likelihood estimation method and handling missing covariates, are also covered. Readers will learn a single framework to apply both meta-analysis and SEM. Examples in R and in Mplus are included. This book will be a valuable resource for statistical and academic researchers and graduate students carrying out meta-analyses, and will also be useful to researchers and statisticians using SEM in biostatistics. Basic knowledge of either SEM or meta-analysis will be helpful in understanding the materials in this book.
Local Note:
Electronic reproduction. Ann Arbor, Michigan : ProQuest Ebook Central, 2017. Available via World Wide Web. Access may be limited to ProQuest Ebook Central affiliated libraries.
Genre:
Electronic Access:
Click to View