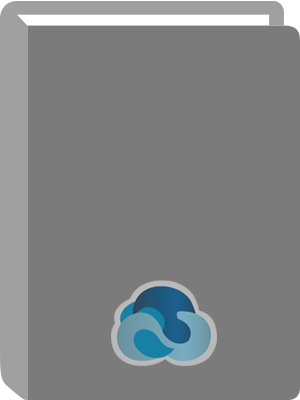
Multiscale Modeling of Cancer : An Integrated Experimental and Mathematical Modeling Approach.
Title:
Multiscale Modeling of Cancer : An Integrated Experimental and Mathematical Modeling Approach.
Author:
Cristini, Vittorio.
ISBN:
9780511799129
Personal Author:
Physical Description:
1 online resource (300 pages)
Contents:
Cover -- Half-title -- Title -- Copyright -- Contents -- List of contributors -- Preface -- Acknowledgements -- Notation -- Sets and set operations -- Number systems and operations -- Vectors, matrices, and tensors -- Topology -- Differentiation and integration -- Special functions -- Probability and statistics -- I Theory -- 1 Introduction -- 2 Biological background -- 2.1 Key molecular and cellular biology -- 2.1.1 Tissue microarchitecture and maintenance -- 2.1.2 Cellular adhesion and cell sorting -- 2.1.3 Subcellular structure -- 2.1.4 Cell cycle, proliferation, and apoptosis -- 2.1.5 Genetics, gene expression, and cell signaling -- 2.1.6 Cell motility -- 2.1.7 Hypoxia, necrosis, and calcification -- 2.2 The biology of cancer -- 2.2.1 Carcinogenesis -- 2.2.2 Avascular solid-tumor growth -- 2.2.3 Interaction with the microenvironment -- 2.2.4 Vascular growth and metastasis -- 2.3 Concluding remarks -- 3 Continuum tumor modeling: single phase -- 3.1 Introduction -- 3.1.1 Background -- 3.2 Avascular tumor growth -- 3.2.1 Model without necrosis -- 3.2.2 Growth in heterogeneous tissue -- 3.2.3 Effect of stress -- 3.3 Vascularized tumor growth -- 3.3.1 Background -- 3.3.2 Modeling of angiogenesis -- 3.3.3 Basic model -- 3.3.4 Coupling of tumor growth with angiogenesis -- 3.4 Conclusion -- 4 Analysis and calibration of single-phase continuum tumor models -- 4.1 Regimes of growth -- 4.2 Linear analysis -- 4.3 Nonlinear results -- 4.4 Model calibration from experimental data -- 4.5 Tumor growth with chemotaxis -- 4.6 Tumor growth with necrosis -- 4.7 Tumor growth in heterogeneous microenvironments -- 4.8 Vascular tumor growth -- 4.9 Conclusion -- 5 Continuum tumor modeling: multiphase -- 5.1 Background -- 5.2 General conservation equations -- 5.3 A solid--liquid biphasic model -- 5.4 Liquid--liquid mixture model I.
5.5 Liquid--liquid mixture model II -- 5.6 A special case of the generalized Darcy's law liquid--liquid mixture model -- 5.7 Fluxes and velocities -- 5.8 Mass-exchange terms -- 5.9 Diffusion of cell substrates -- 5.10 Mutation of tumor-cell species -- 5.11 Nonlinear results: avascular growth -- 5.11.1 Morphological instability as an invasive mechanism -- 5.11.2 Chemotaxis as an invasive mechanism -- 5.12 Modeling angiogenesis in three dimensions -- 5.12.1 Background -- 5.12.2 Biased circular random walk -- 5.12.3 Branches and anastomoses -- 5.13 Nonlinear results: vascularized tumor growth -- 6 Discrete cell modeling -- 6.1 A brief review of discrete modeling in cancer biology -- 6.1.1 Lattice-based models -- 6.1.2 Lattice-free models -- 6.1.3 Comparison with continuum methods -- 6.1.4 Some discrete modeling examples -- 6.2 An agent-based cell modeling framework -- 6.2.1 A brief review of exponential random variables and Poisson processes -- 6.2.2 A family of potential functions -- 6.2.3 Cell states -- 6.2.4 Forces acting on the cells -- 6.3 Subcellular modeling -- 6.4 Dynamic coupling with the microenvironment -- 6.5 A brief analysis of the volume-averaged model behavior -- 6.6 Numerical examples from breast cancer -- 6.6.1 Baseline calibrated run -- 6.6.2 Impact of hypoxic survival time -- 6.6.3 Impact of the cell lysis time -- 6.6.4 Impact of background oxygen decay rate -- 6.6.5 Impact of cell motility -- 6.7 Conclusions -- 7 Hybrid continuum--discrete tumor models -- 7.1 Background -- 7.2 General concept and conservation laws -- 7.2.1 Conservation laws in continuum models -- 7.2.2 Conservation laws in discrete models -- 7.2.3 ``Inertialess'' assumption -- 7.2.4 Linking discrete and continuum variables -- 7.3 Mass and momentum exchange -- 7.3.1 The continuum-to-discrete (C2D) conversion -- 7.3.2 The discrete-to-continuum (D2C) conversion.
7.3.3 Momentum exchange through continuum--discrete interactions -- 7.3.4 Summary of the hybrid modeling framework -- 7.4 A hybrid model of multicellular tumor spheroids (MCTSs) -- 7.4.1 The continuum and the discrete components -- 7.4.2 Continuum--discrete coupling mechanism -- 7.4.3 Results -- 7.4.4 Discussion -- 7.5 A hybrid model of vascularized tumor growth -- 7.5.1 The continuum and the discrete components -- 7.5.2 The C2D conversion process -- 7.5.3 The D2C conversion process -- 7.5.4 Momentum exchange through continuum--discrete interactions -- 7.5.5 Results -- 7.5.6 Discussion -- 8 Numerical schemes -- 8.1 Review of the multiphase mixture model -- 8.2 Uniform mesh discretization -- 8.2.1 The discrete gradient operator d -- 8.2.2 Treatment of the advection terms -- 8.2.3 Discretization of the boundary conditions -- 8.2.4 Mesh discretization across interfaces -- 8.2.5 Treatment of the source terms -- 8.3 Multigrid mesh hierarchy and block-structured adaptive mesh -- 8.3.1 Block-structured adaptive-mesh refinement -- 8.3.2 Initialization of the refined meshes -- 8.4 Multigrid method on adaptive meshes: the MLAT--FAS scheme -- 8.4.1 Operators and sources -- 8.4.2 The MLAT--FAS V-cycle method -- 8.4.3 Smoothing procedures -- 8.4.4 Restriction and interpolation -- 8.4.5 Criteria for mesh refinement -- II Applications -- 9 Continuum tumor modeling: a multidisciplinary approach -- 9.1 Introduction -- 9.1.1 Avascular growth -- 9.1.2 Vascularized tumors -- 9.1.3 Clinical observations -- 9.2 Application to brain cancer -- 9.2.1 Avascular growth -- 9.2.2 Vascularized tumors -- 9.2.3 Clinical observations -- 9.3 Modeling outlook -- 10 Agent-based modeling of breast cancer -- 10.1 Introduction -- 10.1.1 Biology of breast-duct epithelium -- 10.1.2 Biology of DCIS -- 10.2 Adaptation of the agent model -- 10.2.1 Oxygen and metabolism -- 10.2.2 Duct geometry.
10.2.3 Intraductal oxygen diffusion -- 10.2.4 Numerical method -- 10.3 Patient-specific calibration with patient data -- 10.3.1 Estimating ``universal'' parameters -- 10.3.2 Calibrating patient-specific parameters -- 10.3.3 Sample patient calibration and verification -- 10.4 Case studies -- 10.4.1 Estimating difficult physical parameters -- 10.4.2 Generating and testing hypotheses -- 10.4.3 Calibrating multiscale modeling frameworks: preliminary results -- 10.5 Concluding remarks -- 11 Conclusion -- References -- Index.
Abstract:
Uniquely connecting theory with experimental data, this book highlights the potential of advanced computational methods in cancer research.
Local Note:
Electronic reproduction. Ann Arbor, Michigan : ProQuest Ebook Central, 2017. Available via World Wide Web. Access may be limited to ProQuest Ebook Central affiliated libraries.
Genre:
Added Author:
Electronic Access:
Click to View