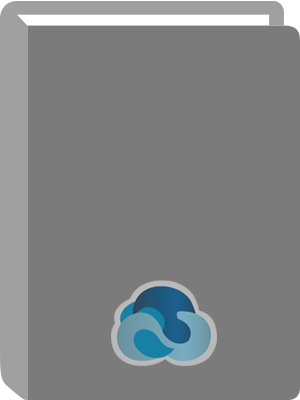
Simplicity, Complexity and Modelling.
Title:
Simplicity, Complexity and Modelling.
Author:
Christie, Mike.
ISBN:
9781119951452
Personal Author:
Edition:
1st ed.
Physical Description:
1 online resource (221 pages)
Series:
Statistics in Practice ; v.114
Statistics in Practice
Contents:
Simplicity, Complexity and Modelling -- Contents -- Preface -- Acknowledgements -- Contributing authors -- 1 Introduction -- 1.1 The origins of the SCAM project -- 1.2 The scope of modelling in the modern world -- 1.3 The different professions and traditions engaged in modelling -- 1.4 Different types of models -- 1.5 Different purposes for modelling -- 1.6 The purpose of the book -- 1.7 Overview of the chapters -- References -- 2 Statistical model selection -- 2.1 Introduction -- 2.2 Explanation or prediction? -- 2.3 Levels of uncertainty -- 2.4 Bias-variance trade-off -- 2.5 Statistical models -- 2.5.1 Within-model inference -- 2.6 Model comparison -- 2.7 Bayesian model comparison -- 2.7.1 Model uncertainty -- 2.7.2 Laplace approximation -- 2.8 Penalized likelihood -- 2.8.1 Bayesian information criterion -- 2.9 The Akaike information criterion -- 2.9.1 Inconsistency of AIC -- 2.10 Significance testing -- 2.11 Many variables -- 2.12 Data-driven approaches -- 2.12.1 Cross-validation -- 2.12.2 Prequential analysis -- 2.13 Model selection or model averaging? -- References -- 3 Modelling in drug development -- 3.1 Introduction -- 3.2 The nature of drug development and scope for statistical modelling -- 3.3 Simplicity versus complexity in phase III trials -- 3.3.1 The nature of phase III trials -- 3.3.2 The case for simplicity in analysing phase III trials -- 3.3.3 The case for complexity in modelling clinical trials -- 3.4 Some technical issues -- 3.4.1 The effect of covariate adjustment in linear models -- 3.4.2 The effect of covariate adjustment in non-linear models -- 3.4.3 Random effects in multi-centre trials -- 3.4.4 Subgroups and interactions -- 3.4.5 Bayesian approaches -- 3.5 Conclusion -- 3.6 Appendix: The effect of covariate adjustment on the variance multiplier in least squares -- References.
4 Modelling with deterministic computer models -- 4.1 Introduction -- 4.2 Metamodels and emulators for computationally expensive simulators -- 4.2.1 Gaussian processes emulators -- 4.2.2 Multivariate outputs -- 4.3 Uncertainty analysis -- 4.4 Sensitivity analysis -- 4.4.1 Variance-based sensitivity analysis -- 4.4.2 Value of information -- 4.5 Calibration and discrepancy -- 4.6 Discussion -- References -- 5 Modelling future climates -- 5.1 Introduction -- 5.2 What is the risk from climate change? -- 5.3 Climate models -- 5.4 An anatomy of uncertainty -- 5.4.1 Aleatoric uncertainty -- 5.4.2 Epistemic uncertainty -- 5.5 Simplicity and complexity -- 5.6 An example: The collapse of the thermohaline circulation -- 5.7 Conclusions -- References -- 6 Modelling climate change impacts for adaptation assessments -- 6.1 Introduction -- 6.1.1 Climate impact assessment -- 6.2 Modelling climate change impacts: From world development paths to localized impacts -- 6.2.1 Greenhouse gas emissions -- 6.2.2 Climate models -- 6.2.3 Downscaling -- 6.2.4 Regional/local climate change impacts -- 6.3 Discussion -- 6.3.1 Multiple routes of uncertainty assessment -- 6.3.2 What is the appropriate balance between simplicity and complexity? -- References -- 7 Modelling in water distribution systems -- 7.1 Introduction -- 7.2 Water distribution system models -- 7.2.1 Water distribution systems -- 7.2.2 WDS hydraulic models -- 7.2.3 Uncertainty in WDS hydraulic modelling -- 7.3 Calibration of WDS hydraulic models -- 7.3.1 Calibration problem -- 7.3.2 Existing approaches -- 7.3.3 Case study -- 7.4 Sampling design for calibration -- 7.4.1 Sampling design problem -- 7.4.2 Existing approaches -- 7.4.3 Case study -- 7.5 Summary and conclusions -- References -- 8 Modelling for flood risk management -- 8.1 Introduction -- 8.2 Flood risk management -- 8.2.1 Long-term change.
8.2.2 Uncertainty -- 8.3 Multi-purpose management -- 8.4 Modelling for flood risk management -- 8.4.1 Source -- 8.4.2 Pathway -- 8.4.3 Receptors -- 8.4.4 An example of a system model: Towyn -- 8.5 Model choice -- 8.6 Conclusions -- References -- 9 Uncertainty quantification and oil reservoir modelling -- 9.1 Introduction -- 9.2 Bayesian framework -- 9.2.1 Solution errors -- 9.3 Quantifying uncertainty in prediction of oil recovery -- 9.3.1 Stochastic sampling algorithms -- 9.3.2 Computing uncertainties from multiple history matched models -- 9.4 Inverse problems and reservoir model history matching -- 9.4.1 Synthetic problems -- 9.4.2 Imperial college fault model -- 9.4.3 Comparison of algorithms on a real field example -- 9.5 Selecting appropriate detail in models -- 9.5.1 Adaptive multiscale estimation -- 9.5.2 Bayes factors -- 9.5.3 Application of solution error modelling -- 9.6 Summary -- References -- 10 Modelling in radioactive waste disposal -- 10.1 Introduction -- 10.2 The radioactive waste problem -- 10.2.1 What is radioactive waste? -- 10.2.2 How much radioactive waste is there? -- 10.2.3 What are the options for long-term management of radioactive waste? -- 10.3 The treatment of uncertainty in radioactive waste disposal -- 10.3.1 Deep geological disposal -- 10.3.2 Repository performance assessment -- 10.3.3 Modelling -- 10.3.4 Model verification and validation -- 10.3.5 Strategies for dealing with uncertainty -- 10.4 Summary and conclusions -- References -- 11 Issues for modellers -- 11.1 What are models and what are they useful for? -- 11.2 Appropriate levels of complexity -- 11.3 Uncertainty -- 11.3.1 Model inputs and parameter uncertainty -- 11.3.2 Model uncertainty -- References -- Glossary -- Index.
Abstract:
Edited by Mike Christie, Institute of Petroleum Engineering, Heriot Watt University, Edinburgh, UK Andrew Cliffe, School of Mathematical Sciences, University of Nottingham, UK Philip Dawid, Centre for Mathematical Sciences, University of Cambridge, UK Stephen Senn, Department of Statistics, University of Glasgow, UK Several points of disagreement exist between different modelling traditions as to whether complex models are always better than simpler models, as to how to combine results from different models and how to propagate model uncertainty into forecasts. This book represents the result of collaboration between scientists from many disciplines to show how these conflicts can be resolved. Key Features: Introduces important concepts in modelling, outlining different traditions in the use of simple and complex modelling in statistics. Provides numerous case studies on complex modelling, such as climate change, flood risk and new drug development. Concentrates on varying models, including flood risk analysis models, the petrol industry forecasts and summarizes the evolution of water distribution systems. Written by experienced statisticians and engineers in order to facilitate communication between modellers in different disciplines. Provides a glossary giving terms commonly used in different modelling traditions. This book provides a much-needed reference guide to approaching statistical modelling. Scientists involved with modelling complex systems in areas such as climate change, flood prediction and prevention, financial market modelling and systems engineering will benefit from this book. It will also be a useful source of modelling case histories.
Local Note:
Electronic reproduction. Ann Arbor, Michigan : ProQuest Ebook Central, 2017. Available via World Wide Web. Access may be limited to ProQuest Ebook Central affiliated libraries.
Subject Term:
Genre:
Electronic Access:
Click to View