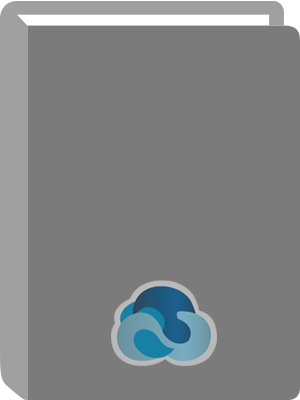
Optimum Experimental Designs, with SAS.
Title:
Optimum Experimental Designs, with SAS.
Author:
Atkinson, Anthony.
ISBN:
9780191537943
Personal Author:
Physical Description:
1 online resource (528 pages)
Series:
Oxford Statistical Science Series ; v.No. 34
Oxford Statistical Science Series
Contents:
Contents -- PART I: BACKGROUND -- 1 Introduction -- 1.1 Some Examples -- 1.2 Scope and Limitations -- 1.3 Background Reading -- 2 Some Key Ideas -- 2.1 Scaled Variables -- 2.2 Design Regions -- 2.3 Random Error -- 2.4 Unbiasedness, Validity, and Efficiency -- 3 Experimental Strategies -- 3.1 Objectives of the Experiment -- 3.2 Stages in Experimental Research -- 3.3 The Optimization of Yield -- 3.4 Further Reading -- 4 The Choice of a Model -- 4.1 Linear Models for One Factor -- 4.2 Non-linear Models -- 4.3 Interaction -- 4.4 Response Surface Models -- 5 Models and Least Squares -- 5.1 Simple Regression -- 5.2 Matrices and Experimental Design -- 5.3 Least Squares -- 5.4 Further Reading -- 6 Criteria for a Good Experiment -- 6.1 Aims of a Good Experiment -- 6.2 Confidence Regions and the Variance of Prediction -- 6.3 Contour Plots of Variances for Two-Factor Designs -- 6.4 Variance-Dispersion Graphs -- 6.5 Some Criteria for Optimum Experimental Designs -- 7 Standard Designs -- 7.1 Introduction -- 7.2 2[sup(m)] Factorial Designs -- 7.3 Blocking 2[sup(m)] Factorial Designs -- 7.4 2[sup(m-f)] Fractional Factorial Designs -- 7.5 Plackett-Burman Designs -- 7.6 Composite Designs -- 7.7 Standard Designs in SAS -- 7.8 Further Reading -- 8 The Analysis of Experiments -- 8.1 Introduction -- 8.2 Example 1.1 Revisited: The Desorption of Carbon Monoxide -- 8.3 Example 1.2 Revisited: The Viscosity of Elastomer Blends -- 8.4 Selecting Effects in Saturated Fractional Factorial Designs -- 8.5 Robust Design -- 8.6 Analysing Data with SAS -- PART II: THEORY AND APPLICATIONS -- 9 Optimum Design Theory -- 9.1 Continuous and Exact Designs -- 9.2 The General Equivalence Theorem -- 9.3 Exact Designs and the General Equivalence Theorem -- 9.4 Algorithms for Continuous Designs and the General Equivalence Theorem -- 9.5 Function Optimization and Continuous Design.
9.6 Finding Continuous Optimum Designs Using SAS/IML Software -- 10 Criteria of Optimality -- 10.1 A-, D-, and E-optimality -- 10.2 D[sub(A)]-optimality (Generalized D-optimality) -- 10.3 D[sub(S)]-optimality -- 10.4 c-optimality -- 10.5 Linear Optimality: C- and L-optimality -- 10.6 V-optimality: Average Variance -- 10.7 G-optimality -- 10.8 Compound Design Criteria -- 10.9 Compound D[sub(A)]-optimality -- 10.10 D-optimum Designs for Multivariate Responses -- 10.11 Further Reading and Other Criteria -- 11 D-optimum Designs -- 11.1 Properties of D-optimum Designs -- 11.2 The Sequential Construction of Optimum Designs -- 11.3 An Example of Design Efficiency: The Desorption of Carbon Monoxide. Example 1.1 Continued -- 11.4 Polynomial Regression in One Variable -- 11.5 Second-order Models in Several Variables -- 11.6 Further Reading -- 12 Algorithms for the Construction of Exact D-optimum Designs -- 12.1 Introduction -- 12.2 The Exact Design Problem -- 12.3 Basic Formulae for Exchange Algorithms -- 12.4 Sequential Algorithms -- 12.5 Non-sequential Algorithms -- 12.6 The KL and BLKL Exchange Algorithms -- 12.7 Example 12.2: Performance of an Internal Combustion Engine -- 12.8 Other Algorithms and Further Reading -- 13 Optimum Experimental Design with SAS -- 13.1 Introduction -- 13.2 Finding Exact Optimum Designs Using the OPTEX Procedure -- 13.3 Efficiencies and Coding in OPTEX -- 13.4 Finding Optimum Designs Over Continuous Regions Using SAS/IML Software -- 13.5 Finding Exact Optimum Designs Using the ADX Interface -- 14 Experiments with Both Qualitative and Quantitative Factors -- 14.1 Introduction -- 14.2 Continuous Designs -- 14.3 Exact Designs -- 14.4 Designs with Qualitative Factors in SAS -- 14.5 Further Reading -- 15 Blocking Response Surface Designs -- 15.1 Introduction -- 15.2 Models and Design Optimality -- 15.3 Orthogonal Blocking.
15.4 Related Problems and Literature -- 15.5 Optimum Block Designs in SAS -- 16 Mixture Experiments -- 16.1 Introduction -- 16.2 Models and Designs for Mixture Experiments -- 16.3 Constrained Mixture Experiments -- 16.4 Mixture Experiments with Other Factors -- 16.5 Blocking Mixture Experiments -- 16.6 The Amount of a Mixture -- 16.7 Optimum Mixture Designs in SAS -- 16.8 Further Reading -- 17 Non-linear Models -- 17.1 Some Examples -- 17.2 Parameter Sensitivities and D-optimum Designs -- 17.3 Strategies for Local Optimality -- 17.4 Sampling Windows -- 17.5 Locally c-optimum Designs -- 17.6 The Analysis of Non-linear Experiments -- 17.7 A Sequential Experimental Design -- 17.8 Design for Diffierential Equation Models -- 17.9 Multivariate Designs -- 17.10 Optimum Designs for Non-linear Models in SAS -- 17.11 Further Reading -- 18 Bayesian Optimum Designs -- 18.1 Introduction -- 18.2 A General Equivalence Theorem Incorporating Prior Information -- 18.3 Bayesian D-optimum Designs -- 18.4 Bayesian c-optimum Designs -- 18.5 Sampled Parameter Values -- 18.6 Discussion -- 19 Design Augmentation -- 19.1 Failure of an Experiment -- 19.2 Design Augmentation and Equivalence Theory -- 19.3 Examples of Design Augmentation -- 19.4 Exact Optimum Design Augmentation -- 19.5 Design Augmentation in SAS -- 19.6 Further Reading -- 20 Model Checking and Designs for Discriminating Between Models -- 20.1 Introduction -- 20.2 Parsimonious Model Checking -- 20.3 Examples of Designs for Model Checking -- 20.4 Example 20.3. A Non-linear Model for Crop Yield and Plant Density -- 20.5 Exact Model Checking Designs in SAS -- 20.6 Discriminating Between Two Models -- 20.7 Sequential Designs for Discriminating Between Two Models -- 20.8 Developments of T-optimality -- 20.9 Nested Linear Models and D[sub(s)]-optimum Designs -- 20.10 Exact T-optimum Designs in SAS.
20.11 The Analysis of T-optimum Designs -- 20.12 Further Reading -- 21 Compound Design Criteria -- 21.1 Introduction -- 21.2 Design Efficiencies -- 21.3 Compound Design Criteria -- 21.4 Polynomials in One Factor -- 21.5 Model Building and Parameter Estimation -- 21.6 Non-linear Models -- 21.7 Discrimination Between Models -- 21.8 DT-Optimum Designs -- 21.9 CD-Optimum Designs -- 21.10 Optimizing Compound Design Criteria in SAS -- 21.11 Further Reading -- 22 Generalized Linear Models -- 22.1 Introduction -- 22.2 Weighted Least Squares -- 22.3 Generalized Linear Models -- 22.4 Models and Designs for Binomial Data -- 22.5 Optimum Design for Gamma Models -- 22.6 Designs for Generalized Linear Models in SAS -- 22.7 Further Reading -- 23 Response Transformation and Structured Variances -- 23.1 Introduction -- 23.2 Transformation of the Response -- 23.3 Design for a Response Transformation -- 23.4 Response Transformations in Non-linear models -- 23.5 Robust and Compound Designs -- 23.6 Structured Mean-Variance Relationships -- 24 Time-dependent Models with Correlated Observations -- 24.1 Introduction -- 24.2 The Statistical Model -- 24.3 Numerical Example -- 24.4 Multiple Independent Series -- 24.5 Discussion and Further Reading -- 25 Further Topics -- 25.1 Introduction -- 25.2 Crossover Designs -- 25.3 Biased-coin Designs for Clinical Trials -- 25.4 Adaptive Designs for Clinical Trials -- 25.5 Population Designs -- 25.6 Designs Over Time -- 25.7 Neural Networks -- 25.8 In Brief -- 26 Exercises -- Bibliography -- Author Index -- A -- B -- C -- D -- E -- F -- G -- H -- J -- K -- L -- M -- N -- O -- P -- R -- S -- T -- U -- V -- W -- Y -- Z -- Subject Index -- A -- B -- C -- D -- E -- F -- G -- H -- I -- J -- L -- M -- N -- O -- P -- Q -- R -- S -- T -- U -- V -- W -- X -- Z.
Abstract:
This text focuses on optimum experimental design using SAS, a powerful software package that provides a complete set of statistical tools including analysis of variance, regression, categorical data analysis, and multivariate analysis. SAS codes, results, plots, numerous figures and tables are provided, along with a fully supported website. - ;Experiments on patients, processes or plants all have random error, making statistical methods essential for their efficient design and analysis. This book presents the theory and methods of optimum experimental design, making them available through the use of SAS programs. Little previous statistical knowledge is assumed. The first part of the book stresses the importance of models in the analysis of data and introduces least squares fitting and simple optimum experimental designs. The second part presents a more detailed discussion of the general theory and of a wide variety of experiments. The book stresses the use of SAS to provide hands-on solutions for the construction of designs in both standard and non-standard situations. The mathematical theory of the designs is. developed in parallel with their construction in SAS, so providing motivation for the development of the subject. Many chapters cover self-contained topics drawn from science, engineering and pharmaceutical investigations, such as response surface designs, blocking of experiments, designs for mixture experiments and for nonlinear and generalized linear models. Understanding is aided by the provision of "SAS tasks" after most chapters as well as by more traditional exercises and a fully. supported website. The authors are leading experts in key fields and this book is ideal for statisticians and scientists in academia, research and the process and pharmaceutical industries. -.
Local Note:
Electronic reproduction. Ann Arbor, Michigan : ProQuest Ebook Central, 2017. Available via World Wide Web. Access may be limited to ProQuest Ebook Central affiliated libraries.
Genre:
Electronic Access:
Click to View