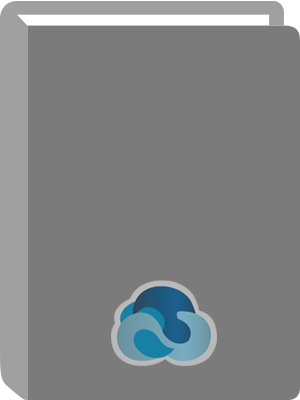
Techniques and Applications of Hyperspectral Image Analysis.
Title:
Techniques and Applications of Hyperspectral Image Analysis.
Author:
Grahn, Hans.
ISBN:
9780470010877
Personal Author:
Edition:
1st ed.
Physical Description:
1 online resource (402 pages)
Contents:
Techniques and Applications of Hyperspectral Image Analysis -- Contents -- Preface -- List of Contributors -- List of Abbreviations -- 1 Multivariate Images, Hyperspectral Imaging: Background and Equipment -- 1.1 Introduction -- 1.2 Digital Images, Multivariate Images and Hyperspectral Images -- 1.3 Hyperspectral Image Generation -- 1.3.1 Introduction -- 1.3.2 Point Scanning Imaging -- 1.3.3 Line Scanning Imaging -- 1.3.4 Focal Plane Scanning Imaging -- 1.4 Essentials of Image Analysis Connecting Scene and Variable Spaces -- References -- 2 Principles of Multivariate Image Analysis (MIA) in Remote Sensing, Technology and Industry -- 2.1 Introduction -- 2.1.1 MIA Approach: Synopsis -- 2.2 Dataset Presentation -- 2.2.1 Master Dataset: Rationale -- 2.2.2 Montmorency Forest, Quebec, Canada: Forestry Background -- 2.3 Tools in MIA -- 2.3.1 MIA Score Space Starting Point -- 2.3.2 Colour-slice Contouring in Score Cross-plots: a 3-D Histogram -- 2.3.3 Brushing: Relating Different Score Cross-plots -- 2.3.4 Joint Normal Distribution (or Not) -- 2.3.5 Local Models/Local Modelling: a Link to Classification -- 2.4 MIA Analysis Concept: Master Dataset Illustrations -- 2.4.1 A New Topographic Map Analogy -- 2.4.2 MIA Topographic Score Space Delineation of Single Classes -- 2.4.3 MIA Delineation of End-member Mixing Classes -- 2.4.4 Which to Use? When? How? -- 2.4.5 Scene-space Sampling in Score Space -- 2.5 Conclusions -- References -- 3 Clustering and Classification in Multispectral Imaging for Quality Inspection of Postharvest Products -- 3.1 Introduction to Multispectral Imaging in Agriculture -- 3.1.1 Measuring Quality -- 3.1.2 Spectral Imaging in Agriculture -- 3.2 Unsupervised Classification of Multispectral Images -- 3.2.1 Unsupervised Classification with FCM -- 3.2.2 FCM Clustering -- 3.2.3 cFCM Clustering -- 3.2.4 csiFCM.
3.2.5 Combining Spectral and Spatial Information -- 3.2.6 sgFCM Clustering -- 3.3 Supervised Classification of Multispectral Images -- 3.3.1 Multivariate Image Analysis for Training Set Selection -- 3.3.2 FEMOS -- 3.3.3 Experiment with a Multispectral Image of Pine and Spruce Wood -- 3.3.4 Clustering with FEMOS Procedure -- 3.4 Visualization and Coloring of Segmented Images and Graphs: Class Coloring -- 3.5 Conclusions -- References -- 4 Self-modeling Image Analysis with SIMPLISMA -- 4.1 Introduction -- 4.2 Materials and Methods -- 4.2.1 FTIR Microscopy -- 4.2.2 SIMS Imaging of a Mixture of Palmitic and Stearic Acids on Aluminum foil -- 4.2.3 Data Analysis -- 4.3 Theory -- 4.4 Results and Discussion -- 4.4.1 FTIR Microscopy Transmission Data of a Polymer Laminate -- 4.4.2 FTIR Reflectance Data of a Mixture of Aspirin and Sugar -- 4.4.3 SIMS Imaging of a Mixture of Palmitic and Stearic Acids on Aluminum Foil -- 4.5 Conclusions -- References -- 5 Multivariate Analysis of Spectral Images Composed of Count Data -- 5.1 Introduction -- 5.2 Example Datasets and Simulations -- 5.3 Component Analysis -- 5.4 Orthogonal Matrix Factorization -- 5.4.1 PCA and Related Methods -- 5.4.2 PCA of Arbitrary Factor Models -- 5.4.3 Maximum Likelihood PCA (MLPCA) -- 5.4.4 Weighted PCA (WPCA) -- 5.4.5 Principal Factor Analysis (PFA) -- 5.4.6 Selecting the Number of Components -- 5.5 Maximum Likelihood Based Approaches -- 5.5.1 Poisson Non-negative Matrix Factorization (PNNMF) -- 5.5.2 Iteratively Weighted Least Squares (IWLS) -- 5.5.3 NNMF: Gaussian Case (Approximate Noise) -- 5.5.4 Factored NNMF: Gaussian Case (Approximate Data) -- 5.5.5 Alternating Least Squares (ALS) -- 5.5.6 Performance Comparisons -- 5.6 Conclusions -- Acknowledgements -- References -- 6 Hyperspectral Image Data Conditioning and Regression Analysis -- 6.1 Introduction.
6.2 Terminology 1286.3 Multivariate Image -- 6.3 Multivariate Image Regression -- 6.3.1 Regression Diagnostics -- 6.3.2 Differences between Normal Calibration and Image Calibration -- 6.4 Data Conditioning -- 6.4.1 Reflectance Transformation and Standardization -- 6.4.2 Spectral Transformations -- 6.4.3 Data Clean-up -- 6.4.4 Data Conditioning Summary -- 6.5 PLS Regression Optimization -- 6.5.1 Data Subset Selection -- 6.5.2 Pseudorank Determination -- 6.6 Regression Examples -- 6.6.1 Artificial Ternary Mixture -- 6.6.2 Commercial Cheese Samples -- 6.6.3 Wheat Straw Wax -- 6.7 Conclusions -- Acknowledgements -- References -- 7 Principles of Image Cross-validation (ICV): Representative Segmentation of Image Data Structures -- 7.1 Introduction -- 7.2 Validation Issues -- 7.2.1 2-way -- 7.2.2 MIA/MIR -- 7.3 Case Studies -- 7.3.1 Case 1: Full Y-image -- 7.3.2 Case 2: Critical Segmentation Issues -- 7.3.3 Case 3: Y-composite -- 7.3.4 Case 4: Image Data Structure Sampling -- 7.4 Discussion and Conclusions -- 7.5 Reflections on 2-way Cross-validation -- References -- 8 Detection, Classification, and Quantification in Hyperspectral Images Using Classical Least Squares Models -- 8.1 Introduction -- 8.2 CLS Models -- 8.2.1 CLS -- 8.2.2 ELS -- 8.2.3 GLS -- 8.3 Detection, Classification, and Quantification -- 8.3.1 Detection -- 8.3.2 Classification -- 8.3.3 Quantification -- 8.4 Conclusions -- Acknowledgements -- References -- 9 Calibration Standards and Image Calibration -- 9.1 Introduction -- 9.2 The Need for Calibration in General -- 9.3 The Need for Image Calibration -- 9.4 Resolution in Hyperspectral Images -- 9.5 Spectroscopic Definitions -- 9.6 Calibration Standards -- 9.7 Calibration in Hyperspectral Images -- 9.8 Conclusions -- References -- 10 Multivariate Movies and their Applications in Pharmaceutical and Polymer Dissolution Studies.
10.1 Introduction -- 10.1.1 Introducing the Time Axis -- 10.1.2 Data Structure and Reduction -- 10.1.3 Compression of Spectra -- 10.1.4 Space Dimensions -- 10.1.5 Time Dimension -- 10.1.6 Simultaneous Compression of all Variables -- 10.2 Applications: Solvent Diffusion and Pharmaceutical Studies -- 10.2.1 Solvent Diffusion in Polymers -- 10.2.2 Optical and NMR Studies -- 10.2.3 Line Imaging -- 10.2.4 Global MIR Imaging Studies of Solvent Intake -- 10.3 Drug Release -- 10.3.1 ATR-FTIR Imaging -- 10.4 Conclusions -- Acknowledgement -- References -- 11 Multivariate Image Analysis of Magnetic Resonance Images: Component Resolution with the Direct Exponential Curve Resolution Algorithm (DECRA) -- 11.1 Introduction -- 11.2 DECRA Approach -- 11.3 DECRA Algorithm -- 11.4 1H Relaxation -- 11.5 T1 Transformation -- 11.6 Imaging Methods -- 11.7 Phantom Images -- 11.7.1 T2 Series -- 11.7.2 T1 Series -- 11.8 Brain Images -- 11.8.1 T2 Series -- 11.8.2 T1 Series -- 11.9 Regression Analysis -- 11.10 Conclusions -- References -- 12 Hyperspectral Imaging Techniques: an Attractive Solution for the Analysis of Biological and Agricultural Materials -- 12.1 Introduction -- 12.2 Sample Characterization and Chemical Species Distribution -- 12.2.1 Analysis of Fruit -- 12.2.2 Analysis of Kernels -- 12.2.3 Analysis of Food and Feed Mixtures -- 12.3 Detecting Contamination and Defects in Agro-food Products -- 12.3.1 Detecting Contamination in Meat Products -- 12.3.2 Detecting Contamination and Defects in Fruit -- 12.3.3 Detecting Contamination and Defects in Cereals -- 12.3.4 Detecting Contamination in Compound Feed -- 12.4 Other Agronomic and Biological Applications -- 12.5 Conclusion -- References.
13 Application of Multivariate Image Analysis in Nuclear Medicine: Principal Component Analysis (PCA) on Dynamic Human Brain Studies with Positron Emission Tomography (PET) for Discrimination of Areas of Disease at High Noise Levels -- 13.1 Introduction -- 13.2 PET -- 13.2.1 History -- 13.2.2 Principles -- 13.2.3 Scanning Modes in PET -- 13.2.4 Analysis of PET Data/Images -- 13.3 PCA -- 13.3.1 History -- 13.3.2 Definition -- 13.3.3 Pre-processing and Scaling -- 13.3.4 Noise Pre-normalization -- 13.4 Application of PCA in PET -- 13.4.1 SWPCA -- 13.4.2 VWPCA -- 13.4.3 MVWPCA -- 13.5 Conclusions -- References -- 14 Near Infrared Chemical Imaging: Beyond the Pictures -- 14.1 Introduction -- 14.2 Data Measurement -- 14.3 Selection of Samples and Acquisition Schemes -- 14.4 What, How Much and Where -- 14.5 Data Analysis and the Underlying Structure of the Data -- 14.6 Imaging with Statistically Meaningful Spatial Dimensions -- 14.7 Chemical Contrast -- 14.8 Measure, Count and Compare -- 14.9 Correlating Data to Sample Performance and/or Behavior: the Value of NIRCI Data -- 14.10 Conclusions -- References -- Index -- Plates.
Abstract:
Techniques and Applications of Hyperspectral Image Analysis gives an introduction to the field of image analysis using hyperspectral techniques, and includes definitions and instrument descriptions. Other imaging topics that are covered are segmentation, regression and classification. The book discusses how high quality images of large data files can be structured and archived. Imaging techniques also demand accurate calibration, and are covered in sections about multivariate calibration techniques. The book explains the most important instruments for hyperspectral imaging in more technical detail. A number of applications from medical and chemical imaging are presented and there is an emphasis on data analysis including modeling, data visualization, model testing and statistical interpretation.
Local Note:
Electronic reproduction. Ann Arbor, Michigan : ProQuest Ebook Central, 2017. Available via World Wide Web. Access may be limited to ProQuest Ebook Central affiliated libraries.
Genre:
Added Author:
Electronic Access:
Click to View