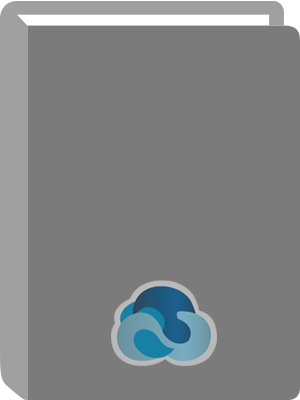
Analyzing the Large Number of Variables in Biomedical and Satellite Imagery : Microarrays and Images.
Title:
Analyzing the Large Number of Variables in Biomedical and Satellite Imagery : Microarrays and Images.
Author:
Good, Phillip I.
ISBN:
9780470937259
Personal Author:
Edition:
1st ed.
Physical Description:
1 online resource (199 pages)
Contents:
ANALYZING THE LARGE NUMBER OF VARIABLES IN BIOMEDICAL AND SATELLITE IMAGERY -- CONTENTS -- Preface -- 1 VERY LARGE ARRAYS -- 1.1 Applications -- 1.2 Problems -- 1.3 Solutions -- 2 PERMUTATION TESTS -- 2.1 Two-Sample Comparison -- 2.1.1 Blocks -- 2.2 k-Sample Comparison -- 2.3 Computing The p-Value -- 2.3.1 Monte Carlo Method -- 2.3.2 An R Program -- 2.4 Multiple-Variable Comparisons -- 2.4.1 Euclidean Distance Matrix Analysis -- 2.4.2 Hotelling's T2 -- 2.4.3 Mantel's U -- 2.4.4 Combining Univariate Tests -- 2.4.5 Gene Set Enrichment Analysis -- 2.5 Categorical Data -- 2.6 Software -- 2.7 Summary -- 3 APPLYING THE PERMUTATION TEST -- 3.1 Which Variables Should Be Included? -- 3.2 Single-Value Test Statistics -- 3.2.1 Categorical Data -- 3.2.2 A Multivariate Comparison Based on a Summary Statistic -- 3.2.3 A Multivariate Comparison Based on Variants of Hotelling's T2 -- 3.2.4 Adjusting for Covariates -- 3.2.5 Pre-Post Comparisons -- 3.2.6 Choosing a Statistic: Time-Course Microarrays -- 3.3 Recommended Approaches -- 3.4 To Learn More -- 4 BIOLOGICAL BACKGROUND -- 4.1 Medical Imaging -- 4.1.1 Ultrasound -- 4.1.2 EEG/MEG -- 4.1.3 Magnetic Resonance Imaging -- 4.1.3.1 MRI -- 4.1.3.2 fMRI -- 4.1.4 Positron Emission Tomography -- 4.2 Microarrays -- 4.3 To Learn More -- 5 MULTIPLE TESTS -- 5.1 Reducing the Number of Hypotheses to Be Tested -- 5.1.1 Normalization -- 5.1.2 Selection Methods -- 5.1.2.1 Univariate Statistics -- 5.1.2.2 Which Statistic? -- 5.1.2.3 Heuristic Methods -- 5.1.2.4 Which Method? -- 5.2 Controlling the OverAll Error Rate -- 5.2.1 An Example: Analyzing Data from Microarrays -- 5.3 Controlling the False Discovery Rate -- 5.3.1 An Example: Analyzing Time-Course Data from Microarrays -- 5.4 Gene Set Enrichment Analysis -- 5.5 Software for Performing Multiple Simultaneous Tests -- 5.5.1 AFNI -- 5.5.2 Cyber-T -- 5.5.3 dChip.
5.5.4 ExactFDR -- 5.5.5 GESS -- 5.5.6 HaploView -- 5.5.7 MatLab -- 5.5.8 R -- 5.5.9 SAM -- 5.5.10 ParaSam -- 5.6 Summary -- 5.7 To Learn More -- 6 THE BOOTSTRAP -- 6.1 Samples and Populations -- 6.2 Precision of an Estimate -- 6.2.1 R Code -- 6.2.2 Applying the Bootstrap -- 6.2.3 Bootstrap Reproducibility Index -- 6.2.4 Estimation in Regression Models -- 6.3 Confidence Intervals -- 6.3.1 Testing for Equivalence -- 6.3.2 Parametric Bootstrap -- 6.3.3 Blocked Bootstrap -- 6.3.4 Balanced Bootstrap -- 6.3.5 Adjusted Bootstrap -- 6.3.6 Which Test? -- 6.4 Determining Sample Size -- 6.4.1 Establish a Threshold -- 6.5 Validation -- 6.5.1 Cluster Analysis -- 6.5.2 Correspondence Analysis -- 6.6 Building a Model -- 6.7 How Large Should The Samples Be? -- 6.8 Summary -- 6.9 To Learn More -- 7 CLASSIFICATION METHODS -- 7.1 Nearest Neighbor Methods -- 7.2 Discriminant Analysis -- 7.3 Logistic Regression -- 7.4 Principal Components -- 7.5 Naive Bayes Classifier -- 7.6 Heuristic Methods -- 7.7 Decision Trees -- 7.7.1 A Worked-Through Example -- 7.8 Which Algorithm Is Best for Your Application? -- 7.8.1 Some Further Comparisons -- 7.8.2 Validation Versus Cross-validation -- 7.9 Improving Diagnostic Effectiveness -- 7.9.1 Boosting -- 7.9.2 Ensemble Methods -- 7.9.3 Random Forests -- 7.10 Software for Decision Trees -- 7.11 Summary -- 8 APPLYING DECISION TREES -- 8.1 Photographs -- 8.2 Ultrasound -- 8.3 MRI Images -- 8.4 EEGs and EMGs -- 8.5 Misclassification Costs -- 8.6 Receiver Operating Characteristic -- 8.7 When the Categories Are As Yet Undefined -- 8.7.1 Unsupervised Principal Components Applied to fMRI -- 8.7.2 Supervised Principal Components Applied to Microarrays -- 8.8 Ensemble Methods -- 8.9 Maximally Diversified Multiple Trees -- 8.10 Putting It All Together -- 8.11 Summary -- 8.12 To Learn More -- Glossary of Biomedical Terminology.
Glossary of Statistical Terminology -- Appendix: An R Primer -- R1 Getting Started -- R1.1 R Functions -- R1.2 Vector Arithmetic -- R2 Store and Retrieve Data -- R2.1 Storing and Retrieving Files from Within R -- R2.2 The Tabular Format -- R2.3 Comma Separated Format -- R3 Resampling -- R3.1 The While Command -- R4 Expanding R's Capabilities -- R4.1 Downloading Libraries of R Functions -- R4.2 Programming Your Own Functions -- Bibliography -- Author Index -- Subject Index.
Abstract:
This book grew out of an online interactive offered through statcourse.com, and it soon became apparent to the author that the course was too limited in terms of time and length in light of the broad backgrounds of the enrolled students. The statisticians who took the course needed to be brought up to speed both on the biological context as well as on the specialized statistical methods needed to handle large arrays. Biologists and physicians, even though fully knowledgeable concerning the procedures used to generate microaarrays, EEGs, or MRIs, needed a full introduction to the resampling methods-the bootstrap, decision trees, and permutation tests, before the specialized methods applicable to large arrays could be introduced. As the intended audience for this book consists both of statisticians and of medical and biological research workers as well as all those research workers who make use of satellite imagery including agronomists and meteorologists, the book provides a step-by-step approach to not only the specialized methods needed to analyze the data from microarrays and images, but also to the resampling methods, step-down multi-comparison procedures, multivariate analysis, as well as data collection and pre-processing. While many alternate techniques for analysis have been introduced in the past decade, the author has selected only those techniques for which software is available along with a list of the available links from which the software may be purchased or downloaded without charge. Topical coverage includes: very large arrays; permutation tests; applying permutation tests; gathering and preparing data for analysis; multiple tests; bootstrap; applying the bootstrap; classification methods; decision trees; and applying decision trees.
Local Note:
Electronic reproduction. Ann Arbor, Michigan : ProQuest Ebook Central, 2017. Available via World Wide Web. Access may be limited to ProQuest Ebook Central affiliated libraries.
Genre:
Electronic Access:
Click to View