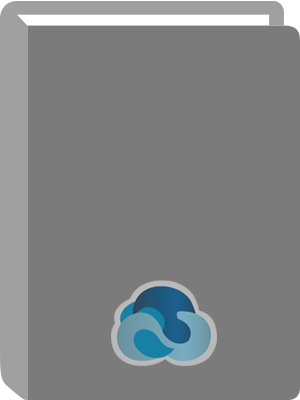
Data Analysis.
Title:
Data Analysis.
Author:
Govaert, Gerard.
ISBN:
9780470610312
Personal Author:
Edition:
1st ed.
Physical Description:
1 online resource (343 pages)
Contents:
Data Analysis -- Contents -- Preface -- Chapter 1. Principal Component Analysis: Application to Statistical Process Control -- 1.1. Introduction -- 1.2. Data table and related subspaces -- 1.2.1. Data and their characteristics -- 1.2.2. The space of statistical units -- 1.2.3. Variables space -- 1.3. Principal component analysis -- 1.3.1. The method -- 1.3.2. Principal factors and principal components -- 1.3.3. Principal factors and principal components properties -- 1.4. Interpretation of PCA results -- 1.4.1. Quality of representations onto principal planes -- 1.4.2. Axis selection -- 1.4.3. Internal interpretation -- 1.4.4. External interpretation: supplementary variables and individuals -- 1.5. Application to statistical process control -- 1.5.1. Introduction -- 1.5.2. Control charts and PCA -- 1.6. Conclusion -- 1.7. Bibliography -- Chapter 2. Correspondence Analysis: Extensions and Applications to the Statistical Analysis of Sensory Data -- 2.1. Correspondence analysis -- 2.1.1. Data, example, notations -- 2.1.2. Questions: independence model -- 2.1.3. Intensity, significance and nature of a relationship between two qualitative variables -- 2.1.4. Transformation of the data -- 2.1.5. Two clouds -- 2.1.6. Factorial analysis of X -- 2.1.7. Aid to interpretation -- 2.1.8. Some properties -- 2.1.9. Relationships to the traditional presentation -- 2.1.10. Example: recognition of three fundamental tastes -- 2.2. Multiple correspondence analysis -- 2.2.1. Data, notations and example -- 2.2.2. Aims -- 2.2.3. MCA and CA -- 2.2.4. Spaces, clouds and metrics -- 2.2.5. Properties of the clouds in CA of the CDT -- 2.2.6. Transition formulae -- 2.2.7. Aid for interpretation -- 2.2.8. Example: relationship between two taste thresholds -- 2.3. An example of application at the crossroads of CA and MCA -- 2.3.1. Data.
2.3.2. Questions: construction of the analyzed table -- 2.3.3. Properties of the CA of the analyzed table -- 2.3.4. Results -- 2.4. Conclusion: two other extensions -- 2.4.1. Internal correspondence analysis -- 2.4.2. Multiple factor analysis (MFA) -- 2.5. Bibliography -- Chapter 3. Exploratory Projection Pursuit -- 3.1. Introduction -- 3.2. General principles -- 3.2.1. Background -- 3.2.2. What is an interesting projection? -- 3.2.3. Looking for an interesting projection -- 3.2.4. Inference -- 3.2.5. Outliers -- 3.3. Some indexes of interest: presentation and use -- 3.3.1. Projection indexes based on entropy measures -- 3.3.2. Projection indexes based on L2 distances -- 3.3.3. Chi-squared type indexes -- 3.3.4. Indexes based on the cumulative empirical function -- 3.4. Generalized principal component analysis -- 3.4.1. Theoretical background -- 3.4.2. Practice -- 3.4.3. Some precisions -- 3.5. Example -- 3.6. Further topics -- 3.6.1. Other indexes, other structures -- 3.6.2. Unsupervised classification -- 3.6.3. Discrete data -- 3.6.4. Related topics -- 3.6.5. Computation -- 3.7. Bibliography -- Chapter 4. The Analysis of Proximity Data -- 4.1. Introduction -- 4.2. Representation of proximity data in a metric space -- 4.2.1. Four illustrative examples -- 4.2.2. Definitions -- 4.3. Isometric embedding and projection -- 4.3.1. An example of computations -- 4.3.2. The additive constant problem -- 4.3.3. The case of observed dissimilarity measures blurred by noise -- 4.4. Multidimensional scaling and approximation -- 4.4.1. The parametric MDS model -- 4.4.2. The Shepard founding heuristics -- 4.4.3. The majorization approach -- 4.4.4. Extending MDS to a semi-parametric setting -- 4.5. A fielded application -- 4.5.1. Principal coordinates analysis -- 4.5.2. Dimensionality for the representation space -- 4.5.3. The scree test.
4.5.4. Recourse to simulations -- 4.5.5. Validation of results -- 4.5.6. The use of exogenous information for interpreting the output configuration -- 4.5.7. Introduction to stochastic modeling in MDS -- 4.6. Bibliography -- Chapter 5. Statistical Modeling of Functional Data -- 5.1. Introduction -- 5.2. Functional framework -- 5.2.1. Functional random variable -- 5.2.2. Smoothness assumption -- 5.2.3. Smoothing splines -- 5.3. Principal components analysis -- 5.3.1. Model and estimation -- 5.3.2. Dimension and smoothing parameter selection -- 5.3.3. Some comments on discretization effects -- 5.3.4. PCA of climatic time series -- 5.4. Linear regression models and extensions -- 5.4.1. Functional linear models -- 5.4.2. Principal components regression -- 5.4.3. Roughness penalty approach -- 5.4.4. Smoothing parameters selection -- 5.4.5. Some notes on asymptotics -- 5.4.6. Generalized linear models and extensions -- 5.4.7. Land use estimation with the temporal evolution of remote sensing data -- 5.5. Forecasting -- 5.5.1. Functional autoregressive process -- 5.5.2. Smooth ARH(1) -- 5.5.3. Locally ARH(1) processes -- 5.5.4. Selecting smoothing parameters -- 5.5.5. Some asymptotic results -- 5.5.6. Prediction of climatic time series -- 5.6. Concluding remarks -- 5.7. Bibliography -- Chapter 6. Discriminant Analysis -- 6.1. Introduction -- 6.2. Main steps in supervised classification -- 6.2.1. The probabilistic framework -- 6.2.2. Sampling schemes -- 6.2.3. Decision function estimation strategies -- 6.2.4. Variables selection -- 6.2.5. Assessing the misclassification error rate -- 6.2.6. Model selection and resampling techniques -- 6.3. Standard methods in supervised classification -- 6.3.1. Linear discriminant analysis -- 6.3.2. Logistic regression -- 6.3.3. The K nearest neighbors method -- 6.3.4. Classification trees.
6.3.5. Single hidden layer back-propagation network -- 6.4. Recent advances -- 6.4.1. Parametric methods -- 6.4.2. Radial basis functions -- 6.4.3. Boosting -- 6.4.4. Support vector machines -- 6.5. Conclusion -- 6.6. Bibliography -- Chapter 7. Cluster Analysis -- 7.1. Introduction -- 7.2. General principles -- 7.2.1. The data -- 7.2.2. Visualizing clusters -- 7.2.3. Types of classification -- 7.2.4. Objectives of clustering -- 7.3. Hierarchical clustering -- 7.3.1. Agglomerative hierarchical clustering (AHC) -- 7.3.2. Agglomerative criteria -- 7.3.3. Example -- 7.3.4. Ward's method or minimum variance approach -- 7.3.5. Optimality properties -- 7.3.6. Using hierarchical clustering -- 7.4. Partitional clustering: the k -means algorithm -- 7.4.1. The algorithm -- 7.4.2. k-means: a family of methods -- 7.4.3. Using the k-means algorithm -- 7.5. Miscellaneous clustering methods -- 7.5.1. Dynamic cluster method -- 7.5.2. Fuzzy clustering -- 7.5.3. Constrained clustering -- 7.5.4. Self-organizing map -- 7.5.5. Clustering variables -- 7.5.6. Clustering high-dimensional datasets -- 7.6. Block clustering -- 7.6.1. Binary data -- 7.6.2. Contingency table -- 7.6.3. Continuous data -- 7.6.4. Some remarks -- 7.7. Conclusion -- 7.8. Bibliography -- Chapter 8. Clustering and the Mixture Model -- 8.1. Probabilistic approaches in cluster analysis -- 8.1.1. Introduction -- 8.1.2. Parametric approaches -- 8.1.3. Non-parametric methods -- 8.1.4. Validation -- 8.1.5. Notation -- 8.2. The mixture model -- 8.2.1. Introduction -- 8.2.2. The model -- 8.2.3. Estimation of parameters -- 8.2.4. Number of components -- 8.2.5. Identifiability -- 8.3. EM algorithm -- 8.3.1. Introduction -- 8.3.2. Complete data and complete-data likelihood -- 8.3.3. Principle -- 8.3.4. Application to mixture models -- 8.3.5. Properties -- 8.3.6. EM: an alternating optimization algorithm.
8.4. Clustering and the mixture model -- 8.4.1. The two approaches -- 8.4.2. Classification likelihood -- 8.4.3. The CEM algorithm -- 8.4.4. Comparison of the two approaches -- 8.4.5. Fuzzy clustering -- 8.5. Gaussian mixture model -- 8.5.1. The model -- 8.5.2. CEM algorithm -- 8.5.3. Spherical form, identical proportions and volumes -- 8.5.4. Spherical form, identical proportions but differing volumes -- 8.5.5. Identical covariance matrices and proportions -- 8.6. Binary variables -- 8.6.1. Data -- 8.6.2. Binary mixture model -- 8.6.3. Parsimonious model -- 8.6.4. Example of application -- 8.7. Qualitative variables -- 8.7.1. Data -- 8.7.2. The model -- 8.7.3. Parsimonious model -- 8.8. Implementation -- 8.8.1. Choice of model and of the number of classes -- 8.8.2. Strategies for use -- 8.8.3. Extension to particular situations -- 8.9. Conclusion -- 8.10. Bibliography -- Chapter 9. Spatial Data Clustering -- 9.1. Introduction -- 9.1.1. The spatial data clustering problem -- 9.1.2. Examples of applications -- 9.2. Non-probabilistic approaches -- 9.2.1. Using spatial variables -- 9.2.2. Transformation of variables -- 9.2.3. Using a matrix of spatial distances -- 9.2.4. Clustering with contiguity constraints -- 9.3. Markov random fields as models -- 9.3.1. Global methods and Bayesian approaches -- 9.3.2. Markov random fields -- 9.3.3. Markov fields for observations and classes -- 9.3.4. Supervised segmentation -- 9.4. Estimating the parameters for a Markov field -- 9.4.1. Supervised estimation -- 9.4.2. Unsupervised estimation with EM -- 9.4.3. Classification likelihood and inertia with spatial smoothing -- 9.4.4. Other methods of unsupervised estimation -- 9.5. Application to numerical ecology -- 9.5.1. The problem -- 9.5.2. The model: Potts field and Bernoulli distributions -- 9.5.3. Estimating the parameters -- 9.5.4. Resulting clustering.
9.6. Bibliography.
Abstract:
The first part of this book is devoted to methods seeking relevant dimensions of data. The variables thus obtained provide a synthetic description which often results in a graphical representation of the data. After a general presentation of the discriminating analysis, the second part is devoted to clustering methods which constitute another method, often complementary to the methods described in the first part, to synthesize and to analyze the data. The book concludes by examining the links existing between data mining and data analysis.
Local Note:
Electronic reproduction. Ann Arbor, Michigan : ProQuest Ebook Central, 2017. Available via World Wide Web. Access may be limited to ProQuest Ebook Central affiliated libraries.
Genre:
Electronic Access:
Click to View