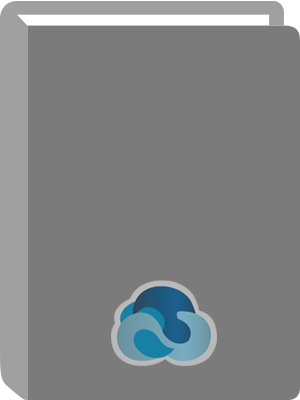
Developing Econometrics.
Title:
Developing Econometrics.
Author:
Tong, Hengqing.
ISBN:
9781119954248
Personal Author:
Edition:
1st ed.
Physical Description:
1 online resource (487 pages)
Contents:
Developing Econometrics -- Contents -- Foreword -- Preface -- Acknowledgements -- 1 Introduction -- 1.1 Nature and Scope of Econometrics -- 1.1.1 What is Econometrics and Why Study Econometrics? -- 1.1.2 Econometrics and Scientific Credibility of Business and Economic Decisions -- 1.2 Types of Economic Problems, Types of Data, and Types of Models -- 1.2.1 Experimental Data from a Marketing Experiment -- 1.2.2 Cross-Section Data: National Sample Survey Data on Consumer Expenditure -- 1.2.3 Non-Experimental Data Taken from Secondary Sources: The Case of Pharmaceutical Industry in India -- 1.2.4 Loan Default Risk of a Customer and the Problem Facing Decision on a Loan Application -- 1.2.5 Panel Data: Performance of Banks in India by the Type of Ownership after Economic Reforms -- 1.2.6 Single Time Series Data: The Bombay Stock Exchange (BSE) Index -- 1.2.7 Multiple Time Series Data: Stock Prices in BRIC Countries -- 1.3 Pattern Recognition and Exploratory Data Analysis -- 1.3.1 Some Basic Issues in Econometric Modeling -- 1.3.2 Exploratory Data Analysis Using Correlations and Scatter Diagrams: The Relative Importance of Managerial Function and Labor -- 1.3.3 Cleaning and Reprocessing Data to Discover Patterns: BSE Index Data -- 1.4 Econometric Modeling: The Roadmap of This Book -- 1.4.1 The Econometric Modeling Strategy -- 1.4.2 Plan of the Book -- Electronic References for Chapter 1 -- References -- 2 Independent Variables in Linear Regression Models -- 2.1 Brief Review of Linear Regression -- 2.1.1 Brief Review of Univariate Linear Regression -- 2.1.2 Brief Review of Multivariate Linear Regression -- 2.2 Selection of Independent Variable and Stepwise Regression -- 2.2.1 Principles of Selection of Independent Variables -- 2.2.2 Stepwise Regression -- 2.3 Multivariate Data Transformation and Polynomial Regression.
2.3.1 Linear Regression after Multivariate Data Transformation -- 2.3.2 Polynomial Regression on an Independent Variable -- 2.3.3 Multivariable Polynomial Regression -- 2.4 Column Multicollinearity in Design Matrix and Ridge Regression -- 2.4.1 Effect of Column Multicollinearity of Design Matrix -- 2.4.2 Ridge Regression -- 2.4.3 Ridge Trace Analysis and Ridge Parameter Selection -- 2.4.4 Generalized Ridge Regression -- 2.5 Recombination of Independent Variable and Principal Components Regression -- 2.5.1 Concept of Principal Components Regression -- 2.5.2 Determination of Principal Component -- Electronic References for Chapter 2 -- References -- 3 Alternative Structures of Residual Error in Linear Regression Models -- 3.1 Heteroscedasticity: Consequences and Tests for Its Existence -- 3.1.1 Consequences of Heteroscedasticity -- 3.1.2 Tests for Heteroscedasticity -- 3.2 Generalized Linear Model with Covariance Being a Diagonal Matrix -- 3.2.1 Diagonal Covariance Matrix and Weighted Least Squares -- 3.2.2 Model with Two Unknown Variances -- 3.2.3 Multiplicative Heteroscedastic Model -- 3.3 Autocorrelation in a Linear Model -- 3.3.1 Linear Model with First-Order Residual Autoregression -- 3.3.2 Autoregressive Conditional Heteroscedasticity (ARCH) Model -- 3.4 Generalized Linear Model with Positive Definite Covariance Matrix -- 3.4.1 Model Definition, Parameter Estimation and Hypothesis Tests -- 3.4.2 Some Equivalent Conditions -- 3.5 Random Effects and Variance Component Model -- 3.5.1 Random Effect Regression Model -- 3.5.2 The Variance Component Model -- 3.5.3 Analysis of Variance Method to Solve Variance Component Model -- 3.5.4 Minimum Norm Quadratic Unbiased Estimation (MINQUE) to Solve Variance Component -- 3.5.5 Maximum Likelihood Method to Solve Variance Component Model -- Electronic References for Chapter 3 -- References.
4 Discrete Variables and Nonlinear Regression Model -- 4.1 Regression Model When Independent Variables are Categorical -- 4.1.1 Problem About Wage and Gender Differences -- 4.1.2 Structural Changes in the Savings Function (Use of Categorical Variables in Combination with Continuous Variables) -- 4.1.3 Cross Section Analysis -- 4.1.4 Seasonal Analysis Model -- 4.2 Models with Categorical or Discrete Dependent Variables -- 4.2.1 Linear Model with Binary Dependent Variable -- 4.2.2 Logit Regression Model -- 4.2.3 Probit Regression Model -- 4.2.4 Tobit Regression Model -- 4.3 Nonlinear Regression Model and Its Algorithm -- 4.3.1 The Least Squares Estimate for Nonlinear Regression Model -- 4.3.2 Maximum Likelihood Estimation of Nonlinear Regression Model -- 4.3.3 Equivalence of Maximum Likelihood Estimation and Least Squares Estimation -- 4.4 Nonlinear Regression Models in Practice -- 4.4.1 Growth Curve Models -- 4.4.2 Box-Cox Transformation Model -- 4.4.3 Survival Data and Failure Rate Model -- 4.4.4 Total Factor Productivity (TFP) -- Electronic References for Chapter 4 -- References -- 5 Nonparametric and Semiparametric Regression Models -- 5.1 Nonparametric Regression and Weight Function Method -- 5.1.1 The Concept of Nonparametric Regression -- 5.1.2 Weight Function Method -- 5.2 Semiparametric Regression Model -- 5.2.1 Linear Semiparametric Regression Model -- 5.2.2 Single-Index Semiparametric Regression Model -- 5.3 Stochastic Frontier Regression Model -- 5.3.1 Stochastic Frontier Linear Regression Model and Asymptotically Efficient Estimator of Its Parameters -- 5.3.2 Semiparametric Stochastic Frontier Model -- Electronic References for Chapter 5 -- References -- 6 Simultaneous Equations Models and Distributed Lag Models -- 6.1 Simultaneous Equations Models and Inconsistency of OLS Estimators.
6.1.1 Demand-and-Supply Model, Keynesian Model and Wage-Price Model (Phillips Curve) -- 6.1.2 Macroeconomic IS Model, LM Model and Klein's Econometric Model -- 6.1.3 Inconsistency of OLS Estimation -- 6.2 Statistical Inference for Simultaneous Equations Models -- 6.2.1 Indirect Least Squares and Generalized Least Squares -- 6.2.2 Two Stage Least Squares -- 6.3 The Concepts of Lag Regression Models -- 6.3.1 Consumption Lag -- 6.3.2 Inflation Lag -- 6.3.3 Deposit Re-Creation -- 6.4 Finite Distributed Lag Models -- 6.4.1 Estimation of Distributed Lag Models When the Lag Length is Known and Finite -- 6.4.2 The Determination of Distributed Lag Length -- 6.5 Infinite Distributed Lag Models -- 6.5.1 Adaptive Expectations Model and Partial Adjustment Model -- 6.5.2 Koyck Transformation and Estimation of Geometric Lag Models -- Electronic References for Chapter 6 -- References -- 7 Stationary Time Series Models -- 7.1 Auto-Regression Model AR( p) -- 7.1.1 AR( p) Model and Stationarity -- 7.1.2 Auto-Covariance Function and Autocorrelation Function of AR( p) Model -- 7.1.3 Spectral Density of AR( p) Model and Partial Correlation Coefficient -- 7.1.4 Estimation of Parameters for AR(p) Model with Known Order p -- 7.1.5 Order Identification for AR( p) Process -- 7.2 Moving Average Model MA(q) -- 7.2.1 MA(q) Model and Its Properties -- 7.2.2 Parameter Estimation of MA(q) Model When the Order q is Known -- 7.2.3 Spectral Density Estimation for MA(q) Process -- 7.2.4 Order Identification for MA(q) Process -- 7.3 Auto-Regressive Moving-Average Process ARMA( p, q) -- 7.3.1 ARMA(p, q) Model and Its Properties -- 7.3.2 Parameter Estimations for ARMA(p, q) Model -- 7.3.3 Test for ARMA(p, q) Model -- 7.3.4 Order Identification for ARMA( p, q) Model -- 7.3.5 Univariate Time Series Modeling: The Basic Issues and Approaches -- Electronic References for Chapter 7 -- References.
8 Multivariate and Nonstationary Time Series Models -- 8.1 Multivariate Stationary Time Series Model -- 8.1.1 General Description of Multivariable Stationary Time Series Model -- 8.1.2 Estimation of Mean and Autocovariance Function of Multivariate Stationary Time Series -- 8.1.3 Vector Autoregression Model of Order p: VAR( p) -- 8.1.4 Wold Decomposition and Impulse-Response -- 8.1.5 Variance Decomposition with VAR( p) -- 8.1.6 Granger Causality with VAR(p) Specification -- 8.2 Nonstationary Time Series -- 8.2.1 Stochastic Trends and Unit Root Processes -- 8.2.2 Test for Unit Root Hypothesis -- 8.3 Cointegration and Error Correction -- 8.3.1 The Concept and Representation of Cointegration -- 8.3.2 Simultaneous (Structural) Equation System (SES) and Vector Auto Regression (VAR) -- 8.3.3 Cointegration and Error Correction Representation -- 8.3.4 Estimation of Parameters of Cointegration Process -- 8.3.5 Test of Hypotheses on the Number of Cointegrating Equations -- 8.4 Autoregression Conditional Heteroscedasticity in Time Series -- 8.4.1 ARCH Model -- 8.4.2 Generalized ARCH Model-GARCH Model -- 8.4.3 Other Generalized Forms of ARCH Model -- 8.5 Mixed Models of Multivariate Regression with Time Series -- 8.5.1 Mixed Model of Multivariate Regression with Time Series -- 8.5.2 Mixed Model of Multivariate Regression and Cointegration with Time Series -- Electronic References for Chapter 8 -- References -- 9 Multivariate Statistical Analysis and Data Analysis -- 9.1 Model of Analysis of Variance -- 9.1.1 Single Factor Analysis of Variance Model -- 9.1.2 Two Factor Analysis of Variance with Non-Repeated Experiment -- 9.1.3 Two Factor Analysis of Variance with Repeated Experiment -- 9.2 Other Multivariate Statistical Analysis Models -- 9.2.1 Discriminate Analysis Model -- 9.2.2 Factor Analysis Model.
9.2.3 Principal Component Analysis and Multidimensional Scaling Method.
Abstract:
Statistical Theories and Methods with Applications to Economics and Business highlights recent advances in statistical theory and methods that benefit econometric practice. It deals with exploratory data analysis, a prerequisite to statistical modelling and part of data mining. It provides recently developed computational tools useful for data mining, analysing the reasons to do data mining and the best techniques to use in a given situation. Provides a detailed description of computer algorithms. Provides recently developed computational tools useful for data mining Highlights recent advances in statistical theory and methods that benefit econometric practice. Features examples with real life data. Accompanying software featuring DASC (Data Analysis and Statistical Computing). Essential reading for practitioners in any area of econometrics; business analysts involved in economics and management; and Graduate students and researchers in economics and statistics.
Local Note:
Electronic reproduction. Ann Arbor, Michigan : ProQuest Ebook Central, 2017. Available via World Wide Web. Access may be limited to ProQuest Ebook Central affiliated libraries.
Genre:
Electronic Access:
Click to View