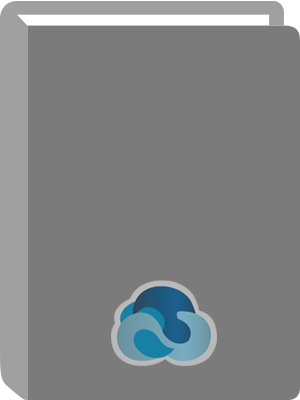
Extreme Events : Robust Portfolio Construction in the Presence of Fat Tails.
Title:
Extreme Events : Robust Portfolio Construction in the Presence of Fat Tails.
Author:
Kemp, Malcolm.
ISBN:
9780470976791
Personal Author:
Edition:
1st ed.
Physical Description:
1 online resource (336 pages)
Series:
The Wiley Finance Series ; v.617
The Wiley Finance Series
Contents:
Extreme Events -- Contents -- Preface -- Acknowledgements -- Abbreviations -- Notation -- 1 Introduction -- 1.1 Extreme events -- 1.2 The portfolio construction problem -- 1.3 Coping with really extreme events -- 1.4 Risk budgeting -- 1.5 Elements designed to maximise benefit to readers -- 1.6 Book structure -- 2 Fat Tails - In Single (i.e., Univariate) Return Series -- 2.1 Introduction -- 2.2 A fat tail relative to what? -- 2.3 Empirical examples of fat-tailed behaviour in return series -- 2.3.1 Introduction -- 2.3.2 Visualising fat tails -- 2.3.3 Behaviour of individual bonds and bond indices -- 2.3.4 Behaviour of equity indices -- 2.3.5 Currencies and other asset types -- 2.4 Characterising fat-tailed distributions by their moments -- 2.4.1 Introduction -- 2.4.2 Skew and kurtosis -- 2.4.3 The (fourth-moment) Cornish-Fisher approach -- 2.4.4 Weaknesses of the Cornish-Fisher approach -- 2.4.5 Improving on the Cornish-Fisher approach -- 2.4.6 Statistical tests for non-Normality -- 2.4.7 Higher order moments and the Omega function -- 2.5 What causes fat tails? -- 2.5.1 Introduction -- 2.5.2 The Central Limit Theorem -- 2.5.3 Ways in which the Central Limit Theorem can break down -- 2.6 Lack of diversification -- 2.7 A time-varying world -- 2.7.1 Introduction -- 2.7.2 Distributional mixtures -- 2.7.3 Time-varying volatility -- 2.7.4 Regime shifts -- 2.8 Stable distributions -- 2.8.1 Introduction -- 2.8.2 Defining characteristics -- 2.8.3 The Generalised Central Limit Theorem -- 2.8.4 Quantile-quantile plots of stable distributions -- 2.9 Extreme value theory (EVT) -- 2.9.1 Introduction -- 2.9.2 Extreme value distributions -- 2.9.3 Tail probability densities -- 2.9.4 Estimation of and inference from tail index values -- 2.9.5 Issues with extreme value theory -- 2.10 Parsimony -- 2.11 Combining different possible source mechanisms.
2.12 The practitioner perspective -- 2.12.1 Introduction -- 2.12.2 Time-varying volatility -- 2.12.3 Crowded trades -- 2.12.4 Liquidity risk -- 2.12.5 'Rational' behaviour versus 'bounded rational' behaviour -- 2.12.6 Our own contribution to the picture -- 2.13 Implementation challenges -- 2.13.1 Introduction -- 2.13.2 Smoothing of return series -- 2.13.3 Time clocks and non-constant time period lengths -- 2.13.4 Price or other data rather than return data -- 2.13.5 Economic sensitivities that change through time -- 3 Fat Tails - In Joint (i.e., Multivariate) Return Series -- 3.1 Introduction -- 3.2 Visualisation of fat tails in multiple return series -- 3.3 Copulas and marginals - Sklar's theorem -- 3.3.1 Introduction -- 3.3.2 Fractile-fractile (i.e., quantile-quantile box) plots -- 3.3.3 Time-varying volatility -- 3.4 Example analytical copulas -- 3.4.1 Introduction -- 3.4.2 The Gaussian copula -- 3.4.3 The t-copula -- 3.4.4 Archimedean copulas -- 3.5 Empirical estimation of fat tails in joint return series -- 3.5.1 Introduction -- 3.5.2 Disadvantages of empirically fitting the copula -- 3.5.3 Multi-dimensional quantile-quantile plots -- 3.6 Causal dependency models -- 3.7 The practitioner perspective -- 3.8 Implementation challenges -- 3.8.1 Introduction -- 3.8.2 Series of different lengths -- 3.8.3 Non-coincidently timed series -- 3.8.4 Cluster analysis -- 3.8.5 Relative entropy and nonlinear cluster analysis -- 4 Identifying Factors That Significantly Influence Markets -- 4.1 Introduction -- 4.2 Portfolio risk models -- 4.2.1 Introduction -- 4.2.2 Fundamental models -- 4.2.3 Econometric models -- 4.2.4 Statistical risk models -- 4.2.5 Similarities and differences between risk models -- 4.3 Signal extraction and principal components analysis -- 4.3.1 Introduction -- 4.3.2 Principal components analysis.
4.3.3 The theory behind principal components analysis -- 4.3.4 Weighting schemas -- 4.3.5 Idiosyncratic risk -- 4.3.6 Random matrix theory -- 4.3.7 Identifying principal components one at a time -- 4.4 Independent components analysis -- 4.4.1 Introduction -- 4.4.2 Practical algorithms -- 4.4.3 Non-Normality and projection pursuit -- 4.4.4 Truncating the answers -- 4.4.5 Extracting all the un-mixing weights at the same time -- 4.4.6 Complexity pursuit -- 4.4.7 Gradient ascent -- 4.5 Blending together principal components analysis and independent components analysis -- 4.5.1 Introduction -- 4.5.2 Including both variance and kurtosis in the importance criterion -- 4.5.3 Eliminating signals from the remaining dataset -- 4.5.4 Normalising signal strength -- 4.6 The potential importance of selection effects -- 4.6.1 Introduction -- 4.6.2 Quantifying the possible impact of selection effects -- 4.6.3 Decomposition of fat-tailed behaviour -- 4.7 Market dynamics -- 4.7.1 Introduction -- 4.7.2 Linear regression -- 4.7.3 Difference equations -- 4.7.4 The potential range of behaviours of linear difference equations -- 4.7.5 Multivariate linear regression -- 4.7.6 'Chaotic' market dynamics -- 4.7.7 Modelling market dynamics using nonlinear methods -- 4.7.8 Locally linear time series analysis -- 4.8 Distributional mixtures -- 4.8.1 Introduction -- 4.8.2 Gaussian mixture models and the expectation-maximisation algorithm -- 4.8.3 k-means clustering -- 4.8.4 Generalised distributional mixture models -- 4.8.5 Regime shifts -- 4.9 The practitioner perspective -- 4.10 Implementation challenges -- 4.10.1 Introduction -- 4.10.2 Local extrema -- 4.10.3 Global extrema -- 4.10.4 Simulated annealing and genetic portfolio optimisation -- 4.10.5 Minimisation/maximisation algorithms -- 4.10.6 Run time constraints -- 5 Traditional Portfolio Construction Techniques.
5.1 Introduction -- 5.2 Quantitative versus qualitative approaches? -- 5.2.1 Introduction -- 5.2.2 Viewing any process through the window of portfolio optimisation -- 5.2.3 Quantitative versus qualitative insights -- 5.2.4 The characteristics of pricing/return anomalies -- 5.3 Risk-return optimisation -- 5.3.1 Introduction -- 5.3.2 Mean-variance optimisation -- 5.3.3 Formal mathematical notation -- 5.3.4 The Capital Asset Pricing Model (CAPM) -- 5.3.5 Alternative models of systematic risk -- 5.4 More general features of mean-variance optimisation -- 5.4.1 Introduction -- 5.4.2 Monotonic changes to risk or return measures -- 5.4.3 Constraint-less mean-variance optimisation -- 5.4.4 Alpha-beta separation -- 5.4.5 The importance of choice of universe -- 5.4.6 Dual benchmarks -- 5.5 Manager selection -- 5.6 Dynamic optimisation -- 5.6.1 Introduction -- 5.6.2 Portfolio insurance -- 5.6.3 Stochastic optimal control and stochastic programming techniques -- 5.7 Portfolio construction in the presence of transaction costs -- 5.7.1 Introduction -- 5.7.2 Different types of transaction costs -- 5.7.3 Impact of transaction costs on portfolio construction -- 5.7.4 Taxes -- 5.8 Risk budgeting -- 5.8.1 Introduction -- 5.8.2 Information ratios -- 5.9 Backtesting portfolio construction techniques -- 5.9.1 Introduction -- 5.9.2 Different backtest statistics for risk-reward trade-offs -- 5.9.3 In-sample versus out-of-sample backtesting -- 5.9.4 Backtesting risk models -- 5.9.5 Backtesting risk-return models -- 5.9.6 Transaction costs -- 5.10 Reverse optimisation and implied view analysis -- 5.10.1 Implied alphas -- 5.10.2 Consistent implementation of investment ideas across portfolios -- 5.11 Portfolio optimisation with options -- 5.12 The practitioner perspective -- 5.13 Implementation challenges -- 5.13.1 Introduction -- 5.13.2 Optimisation algorithms.
5.13.3 Sensitivity to the universe from which ideas are drawn -- 6 Robust Mean-Variance Portfolio Construction -- 6.1 Introduction -- 6.2 Sensitivity to the input assumptions -- 6.2.1 Introduction -- 6.2.2 Estimation error -- 6.2.3 Mitigating estimation error in the covariance matrix -- 6.2.4 Exact error estimates -- 6.2.5 Monte Carlo error estimation -- 6.2.6 Sensitivity to the structure of the smaller principal components -- 6.3 Certainty equivalence, credibility weighting and Bayesian statistics -- 6.3.1 Introduction -- 6.3.2 Bayesian statistics -- 6.3.3 Applying Bayesian statistics in practice -- 6.4 Traditional robust portfolio construction approaches -- 6.4.1 Introduction -- 6.4.2 The Black-Litterman approach -- 6.4.3 Applying the Black-Litterman approach in practice -- 6.4.4 Opinion pooling -- 6.4.5 Impact of non-Normality when using more complex risk measures -- 6.4.6 Using the Herfindahl Hirshman Index (HHI) -- 6.5 Shrinkage -- 6.5.1 Introduction -- 6.5.2 'Shrinking' the sample means -- 6.5.3 'Shrinking' the covariance matrix -- 6.6 Bayesian approaches applied to position sizes -- 6.6.1 Introduction -- 6.6.2 The mathematics -- 6.7 The 'universality' of Bayesian approaches -- 6.8 Market consistent portfolio construction -- 6.9 Resampled mean-variance portfolio optimisation -- 6.9.1 Introduction -- 6.9.2 Different types of resampling -- 6.9.3 Monte Carlo resampling -- 6.9.4 Monte Carlo resampled optimisation without portfolio constraints -- 6.9.5 Monte Carlo resampled optimisation with portfolio constraints -- 6.9.6 Bootstrapped resampled efficiency -- 6.9.7 What happens to the smaller principal components? -- 6.10 The practitioner perspective -- 6.11 Implementation challenges -- 6.11.1 Introduction -- 6.11.2 Monte Carlo simulation - changing variables and importance sampling -- 6.11.3 Monte Carlo simulation - stratified sampling.
6.11.4 Monte Carlo simulation - quasi (i.e., sub-) random sequences.
Abstract:
Taking due account of extreme events when constructing portfolios of assets or liabilities is a key discipline for market professionals. Extreme events are a fact of life in how markets operate. In Extreme Events: Robust Portfolio Construction in the Presence of Fat Tails, leading expert Malcolm Kemp shows readers how to analyse market data to uncover fat-tailed behaviour, how to incorporate expert judgement in the handling of such information, and how to refine portfolio construction methodologies to make portfolios less vulnerable to extreme events or to benefit more from them. This is the only text that combines a comprehensive treatment of modern risk budgeting and portfolio construction techniques with the specific refinements needed for them to handle extreme events. It explains in a logical sequence what constitutes fat-tailed behaviour and why it arises, how we can analyse such behaviour, at aggregate, sector or instrument level, and how we can then take advantage of this analysis. Along the way, it provides a rigorous, comprehensive and clear development of traditional portfolio construction methodologies applicable if fat-tails are absent. It then explains how to refine these methodologies to accommodate real world behaviour. Throughout, the book highlights the importance of expert opinion, showing that even the most data-centric portfolio construction approaches ultimately depend on practitioner assumptions about how the world might behave. The book includes: Key concepts and methods involved in analysing extreme events A comprehensive treatment of mean-variance investing, Bayesian methods, market consistent approaches, risk budgeting, and their application to manager and instrument selection A systematic development of the refinements needed to traditional portfolio construction methodologies to cater for fat-tailed behaviour Latest
developments in stress testing and back testing methodologies A strong focus on the practical implementation challenges that can arise at each step in the process and on how to overcome these challenges "Understanding how to model and analyse the risk of extreme events is a crucial part of the risk management process. This book provides a set of techniques that allow practitioners to do this comprehensively." Paul Sweeting, Professor of Actuarial Science, University of Kent "How can the likeliness of crises affect the construction of portfolios? This question is highly topical in times where we still have to digest the last financial collapse. Malcolm Kemp gives the answer. His book is highly recommended to experts as well as to students in the financial field." Christoph Krischanitz, President Actuarial Association of Austria, Chairman WG "Market Consistency" of Groupe Consultatif.
Local Note:
Electronic reproduction. Ann Arbor, Michigan : ProQuest Ebook Central, 2017. Available via World Wide Web. Access may be limited to ProQuest Ebook Central affiliated libraries.
Genre:
Electronic Access:
Click to View