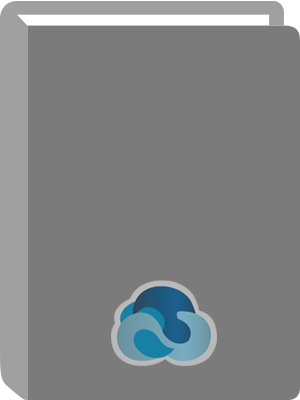
Forecasting Volatility in the Financial Markets.
Title:
Forecasting Volatility in the Financial Markets.
Author:
Satchell, Stephen.
ISBN:
9780080471426
Personal Author:
Edition:
3rd ed.
Physical Description:
1 online resource (428 pages)
Series:
Quantitative Finance
Contents:
Front Cover -- Forecasting Volatility in the Financial Markets -- Copyright Page -- Table of Contents -- List of contributors -- Preface to Third Edition -- Introduction -- Chapter 1 Volatility modelling and forecasting in finance -- 1.1 Introduction -- 1.2 Autoregressive moving average models -- 1.3 Changes in volatility -- 1.3.1 Volatility in financial time series: stylized facts -- 1.3.2 The basic set-up -- 1.4 ARCH models -- 1.4.1 Generalized ARCH -- 1.4.2 Integrated ARCH -- 1.4.3 Exponential ARCH -- 1.4.4 ARCH-M model -- 1.4.5 Fractionally integrated ARCH -- 1.4.6 Other univariate ARCH formulations -- 1.4.7 Multivariate ARCH models -- 1.5 Stochastic variance models -- 1.5.1 From continuous time financial models to discrete time SV models -- 1.5.2 Persistence and the SV model -- 1.5.3 Long memory SV models -- 1.5.4 Risk-return trade-off in SV models -- 1.5.5 Multivariate SV models -- 1.6 Structural changes in the underlying process -- 1.6.1 Regime switching models -- 1.6.2 Extensions of the regime switching models -- 1.7 Threshold models -- 1.7.1 Self-exciting threshold models -- 1.7.2 Open loop threshold models -- 1.7.3 Closed loop threshold models -- 1.7.4 Smooth threshold autoregressive models -- 1.7.5 Identification in SETAR models -- 1.7.6 A threshold AR(1) model -- 1.7.7 A threshold MA model -- 1.7.8 Threshold models and asymmetries in volatility -- 1.7.9 Testing for non-linearity -- 1.7.10 Threshold estimation and prediction of TAR models -- 1.8 Volatility forecasting -- 1.8.1 Volatility forecasting based on time-series models -- 1.8.2 Volatility forecasting based on option ISD (Implied Standard Deviation) -- 1.9 Conclusion -- References and further reading -- Notes -- Chapter 2 What good is a volatility model? -- Abstract -- 2.1 Introduction -- 2.1.1 Notation -- 2.1.2 Types of volatility models.
2.2 Stylized facts about asset price volatility -- 2.2.1 Volatility exhibits persistence -- 2.2.2 Volatility is mean reverting -- 2.2.3 Innovations may have an asymmetric impact on volatility -- 2.2.4 Exogenous variables may influence volatility -- 2.2.5 Tail probabilities -- 2.2.6 Forecast evaluation -- 2.3 An empirical example -- 2.3.1 Summary of the data -- 2.3.2 A volatility model -- 2.3.3 Mean reversion and persistence in volatility -- 2.3.4 An asymmetric volatility model -- 2.3.5 A model with exogenous volatility regressors -- 2.3.6 Aggregation of volatility models -- 2.4 Conclusions and challenges for future research -- References -- Notes -- Chapter 3 Applications of portfolio variety -- Abstract -- 3.1 Introduction -- 3.2 Some applications of variety -- 3.3 Empirical research on variety -- 3.4 Variety and risk estimation -- 3.5 Variety as an explanation of active management styles -- 3.6 Summary -- References -- Chapter 4 A comparison of the properties of realized variance for the FTSE 100 and FTSE 250 equity indices -- 4.1 Introduction -- 4.2 Data -- 4.3 Theory and empirical methodology -- 4.3.1 Realized variance -- 4.3.2 Optimal sampling frequency -- 4.3.3 Estimation -- 4.3.4 Forecasting -- 4.4 Initial data analysis -- 4.5 Estimation and forecasting results -- 4.5.1 GARCH/EGARCH estimation -- 4.5.2 GARCH/EGARCH forecasting -- 4.6 Conclusion -- References -- Notes -- Appendices -- Appendix 4.1: VBA code for RV computation -- Appendix 4.2: EViews output for specification of mean equation -- Chapter 5 An investigation of the relative performance of GARCH models versus simple rules in forecasting volatility -- Abstract -- 5.1 Introduction -- 5.1.1 Measuring volatility -- 5.1.2 Features of volatility -- 5.2 Volatility forecasting methods -- 5.2.1 ARIMA models -- 5.2.2 ARCH/GARCH -- 5.3 Assessing forecasting performance.
5.4 The performance of different forecasting models - empirical results -- 5.5 MSE of linear filter forecasts - theory -- 5.5.1 MSE of moving average methods -- 5.5.2 MSE under GARCH returns -- 5.6 Linear filter vs GARCH forecasts -- 5.6.1 MSE of linear filter forecasts -- 5.6.2 MSE of GARCH forecasts - simulation -- 5.6.3 Discussion of MSE results -- 5.6.4 The effects of leptokurtosis -- 5.7 Other methods of measuring true volatility -- 5.8 Empirical section - UK stock-market volatility -- 5.8.1 Data -- 5.8.2 Methodology -- 5.8.3 Results -- 5.8.4 Summary of results -- 5.9 Conclusion -- References -- Notes -- Chapter 6 Stochastic volatility and option pricing -- Summary -- 6.1 Introduction -- 6.2 The stochastic volatility (SV) model -- 6.2.1 The discrete-time stochastic autoregressive volatility model -- 6.2.2 The continuous-time stochastic volatility model -- 6.2.3 The jump-diffusion model with stochastic volatility -- 6.3 Option pricing under stochastic volatility -- 6.3.1 Pricing options under SV and jump: closed form solutions -- 6.3.2 Pricing options under SV and jump: Monte Carlo simulation -- 6.3.3 Implications of stochastic volatility and jumps on option prices -- 6.4 Estimation of stochastic volatility models -- 6.4.1 Indirect inference: a general estimation approach -- 6.4.2 Efficient method-of-moments (EMM): an efficient estimation approach -- 6.5 Forecasting stochastic volatility -- 6.5.1 Underlying volatility and implied volatility -- 6.5.2 Forecasting volatility based on standard volatility models -- 6.5.3 Forecasting underlying volatility using implied volatility -- 6.6 Conclusion -- 6.7 Appendix: derivation of option pricing formula for general jump-diffusion process with stochastic volatility and stochastic interest rates -- References -- Notes -- Chapter 7 Modelling slippage: an application to the bund futures contract.
7.1 Introduction -- 7.2 Data description -- 7.3 Slippage -- 7.4 Empirical evidence and econometric challenges -- 7.5 Conclusion -- 7.6 Appendix Regression results -- References -- Notes -- Chapter 8 Real trading volume and price action in the foreign exchange markets -- Summary -- 8.1 The currency market -- 8.2 Description of the EBS system -- 8.3 Empirical study of recorded transactions -- 8.4 Number of transactions, volume and volatility -- 8.5 Liquidity cost (Profit) -- 8.6 Liquidity cost and volume -- 8.7 Liquidity cost and time of the day -- 8.8 Final remarks -- References -- Notes -- Chapter 9 Implied risk-neutral probability density functions from option prices: a central bank perspective -- 9.1 Introduction -- 9.2 The relationship between option prices and RND functions -- 9.3 The Black-Scholes formula and its RND function -- 9.4 The implied volatility smile curve -- 9.5 Estimating implied terminal RND functions -- 9.6 Application of the two-lognormal mixture approach to options on short-term interest rates -- 9.7 Monetary policy uses of the information contained in implied RND functions -- 9.7.1 Validating the two-lognormal mixture distribution approach -- 9.7.2 Assessing monetary conditions -- 9.7.3 Assessing monetary credibility -- 9.7.4 Assessing the timing and effectiveness of monetary operations -- 9.7.5 Identifying market anomalies -- 9.8 Using the implied RND function as a volatility forecasting tool -- 9.8.1 Calculating RND-based uncertainty measures -- 9.8.2 Results -- 9.9 Conclusions -- References -- Notes -- Chapter 10 Hashing GARCH: a reassessment of volatility forecasting performance -- Summary -- 10.1 Introduction -- 10.2 ARCH forecasts and properties -- 10.3 Forecasting performance evaluation -- 10.3.1 Statistics-based evaluation -- 10.3.2 Utility-based evaluation -- 10.3.3 Profit-based/preference-free evaluation.
10.4 The pathology of ARCH forecast evaluation -- 10.4.1 A simulation experiment -- 10.5 Some general results -- 10.5.1 The compound normal -- 10.5.2 The Gram-Charlier class -- 10.6 Conclusions -- 10.7 Appendix -- 10.7.1 Mean and variance of ln(v2) -- 10.7.2 Proof of proposition 2 -- References -- Notes -- Chapter 11 Implied volatility forecasting: a comparison of different procedures including fractionally integrated models with applications to UK equity -- Summary -- 11.1 Introduction -- 11.2 Data -- 11.3 Models for volatility -- 11.3.1 GARCH models -- 11.3.2 Log-ARFIMA models -- 11.3.3 Moving average methods -- 11.4 Out-of-sample forecasting performance tests -- 11.4.1 Forecasting procedure -- 11.4.2 Results -- 11.5 Conclusion -- References -- Notes -- Chapter 12 GARCH predictions and the predictions of option prices -- 12.1 Prediction of GARCH models -- 12.2 Numerical results -- 12.3 Application to option pricing models -- References -- Notes -- Chapter 13 Volatility forecasting in a tick data model -- Summary -- 13.1 Introduction -- 13.2 The modelling framework -- 13.3 The functional form of v -- 13.4 Discussion and conclusions -- References -- Chapter 14 An econometic model of downside risk -- Summary -- 14.1 Introduction -- 14.2 Overview of semivariance -- 14.2.1 Introduction to semivariance -- 14.2.2 Limitations of variance as a risk measure -- 14.2.3 Limitations of semivariance -- 14.2.4 Asymmetry of returns and semivariance -- 14.3 Risk modelling techniques -- 14.3.1 The ARCH class of models -- 14.4 Dynamic models of semivariance -- 14.4.1 ARCH-semivariance (ARCH-SV) -- 14.4.2 Extension of model and other issues -- 14.4.3 A generalized ARCH-SV model -- 14.4.4 Estimation of the model -- 14.4.5 Extensions of the GARCH-SV model (GARCH-SV(M)) -- 14.4.6 The differential impact of lagged volatility.
14.4.7 Semivariance and asymmetric ARCH models.
Abstract:
This new edition of Forecasting Volatility in the Financial Markets assumes that the reader has a firm grounding in the key principles and methods of understanding volatility measurement and builds on that knowledge to detail cutting-edge modelling and forecasting techniques. It provides a survey of ways to measure risk and define the different models of volatility and return. Editors John Knight and Stephen Satchell have brought together an impressive array of contributors who present research from their area of specialization related to volatility forecasting. Readers with an understanding of volatility measures and risk management strategies will benefit from this collection of up-to-date chapters on the latest techniques in forecasting volatility. Chapters new to this third edition: * What good is a volatility model? Engle and Patton * Applications for portfolio variety Dan diBartolomeo * A comparison of the properties of realized variance for the FTSE 100 and FTSE 250 equity indices Rob Cornish * Volatility modeling and forecasting in finance Xiao and Aydemir * An investigation of the relative performance of GARCH models versus simple rules in forecasting volatility Thomas A. Silvey * Leading thinkers present newest research on volatility forecasting *International authors cover a broad array of subjects related to volatility forecasting *Assumes basic knowledge of volatility, financial mathematics, and modelling.
Local Note:
Electronic reproduction. Ann Arbor, Michigan : ProQuest Ebook Central, 2017. Available via World Wide Web. Access may be limited to ProQuest Ebook Central affiliated libraries.
Genre:
Added Author:
Electronic Access:
Click to View