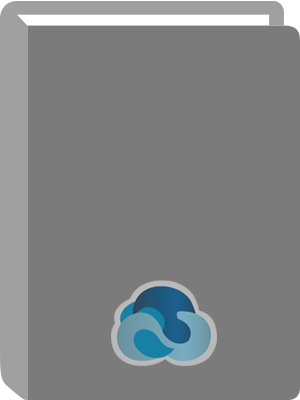
Operational Risk Management : A Practical Approach to Intelligent Data Analysis.
Title:
Operational Risk Management : A Practical Approach to Intelligent Data Analysis.
Author:
Kenett, Ron.
ISBN:
9780470972564
Personal Author:
Edition:
1st ed.
Physical Description:
1 online resource (327 pages)
Contents:
Operational Risk Management -- Contents -- Foreword -- Preface -- Introduction -- Notes on Contributors -- List of Acronyms -- PART I INTRODUCTION TO OPERATIONAL RISK MANAGEMENT -- 1 Risk management: a general view -- 1.1 Introduction -- 1.2 Definitions of risk -- 1.3 Impact of risk -- 1.4 Types of risk -- 1.5 Enterprise risk management -- 1.6 State of the art in enterprise risk management -- 1.6.1 The negative impact of risk silos -- 1.6.2 Technology's critical role -- 1.6.3 Bringing business into the fold -- 1.7 Summary -- References -- 2 Operational risk management: an overview -- 2.1 Introduction -- 2.2 Definitions of operational risk management -- 2.3 Operational risk management techniques -- 2.3.1 Risk identification -- 2.3.2 Control assurance -- 2.3.3 Risk event capture -- 2.3.4 Risk and control assessments -- 2.3.5 Key risk indicators -- 2.3.6 Issues and action management -- 2.3.7 Risk mitigation -- 2.4 Operational risk statistical models -- 2.5 Operational risk measurement techniques -- 2.5.1 The loss distribution approach -- 2.5.2 Scenarios -- 2.5.3 Balanced scorecards -- 2.6 Summary -- References -- PART II DATA FOR OPERATIONAL RISK MANAGEMENT AND ITS HANDLING -- 3 Ontology-based modelling and reasoning in operational risks -- 3.1 Introduction -- 3.1.1 Modules -- 3.1.2 Conceptual model -- 3.2 Generic and axiomatic ontologies -- 3.2.1 Proton extension -- 3.2.2 Temporal ontologies -- 3.3 Domain-independent ontologies -- 3.3.1 Company ontology -- 3.4 Standard reference ontologies -- 3.4.1 XBRL -- 3.4.2 BACH -- 3.4.3 NACE -- 3.5 Operational risk management -- 3.5.1 IT operational risks -- 3.6 Summary -- References -- 4 Semantic analysis of textual input -- 4.1 Introduction -- 4.2 Information extraction -- 4.2.1 Named entity recognition -- 4.3 The general architecture for text engineering -- 4.4 Text analysis components.
4.4.1 Document structure identification -- 4.4.2 Tokenisation -- 4.4.3 Sentence identification -- 4.4.4 Part of speech tagging -- 4.4.5 Morphological analysis -- 4.4.6 Stemming -- 4.4.7 Gazetteer lookup -- 4.4.8 Name recognition -- 4.4.9 Orthographic co-reference -- 4.4.10 Parsing -- 4.5 Ontology support -- 4.6 Ontology-based information extraction -- 4.6.1 An example application: market scan -- 4.7 Evaluation -- 4.8 Summary -- References -- 5 A case study of ETL for operational risks -- 5.1 Introduction -- 5.2 ETL (Extract, Transform and Load) -- 5.2.1 Related work -- 5.2.2 Modeling the conceptual ETL work -- 5.2.3 Modeling the execution of ETL -- 5.2.4 Pentaho data integration -- 5.3 Case study specification -- 5.3.1 Application scenario -- 5.3.2 Data sources -- 5.3.3 Data merging for risk assessment -- 5.3.4 The issues of data merging in MUSING -- 5.4 The ETL-based solution -- 5.4.1 Implementing the 'map merger' activity -- 5.4.2 Implementing the 'alarms merger' activity -- 5.4.3 Implementing the 'financial merger' activity -- 5.5 Summary -- References -- 6 Risk-based testing of web services -- 6.1 Introduction -- 6.2 Background -- 6.2.1 Risk-based testing -- 6.2.2 Web services progressive group testing -- 6.2.3 Semantic web services -- 6.3 Problem statement -- 6.4 Risk assessment -- 6.4.1 Semantic web services analysis -- 6.4.2 Failure probability estimation -- 6.4.3 Importance estimation -- 6.5 Risk-based adaptive group testing -- 6.5.1 Adaptive measurement -- 6.5.2 Adaptation rules -- 6.6 Evaluation -- 6.7 Summary -- References -- PART III OPERATIONAL RISK ANALYTICS -- 7 Scoring models for operational risks -- 7.1 Background -- 7.2 Actuarial methods -- 7.3 Scorecard models -- 7.4 Integrated scorecard models -- 7.5 Summary -- References -- 8 Bayesian merging and calibration for operational risks -- 8.1 Introduction -- 8.2 Methodological proposal.
8.3 Application -- 8.4 Summary -- References -- 9 Measures of association applied to operational risks -- 9.1 Introduction -- 9.2 The arules R script library -- 9.3 Some examples -- 9.3.1 Market basket analysis -- 9.3.2 PBX system risk analysis -- 9.3.3 A bank's operational risk analysis -- 9.4 Summary -- References -- PART IV OPERATIONAL RISK APPLICATIONS AND INTEGRATION WITH OTHER DISCIPLINES -- 10 Operational risk management beyond AMA: new ways to quantify non-recorded losses -- 10.1 Introduction -- 10.1.1 The near miss and opportunity loss project -- 10.1.2 The 'near miss/opportunity loss' service -- 10.1.3 Advantage to the user -- 10.1.4 Outline of the chapter -- 10.2 Non-recorded losses in a banking context -- 10.2.1 Opportunity losses -- 10.2.2 Near misses -- 10.2.3 Multiple losses -- 10.3 Methodology -- 10.3.1 Measure the non-measured -- 10.3.2 IT events vs. operational loss classes -- 10.3.3 Quantification of opportunity losses: likelihood estimates -- 10.3.4 Quantification of near misses: loss approach level -- 10.3.5 Reconnection of multiple losses -- 10.4 Performing the analysis: a case study -- 10.4.1 Data availability: source databases -- 10.4.2 IT OpR ontology -- 10.4.3 Critical path of IT events: Bayesian networks -- 10.4.4 Steps of the analysis -- 10.4.5 Outputs of the service -- 10.5 Summary -- References -- 11 Combining operational risks in financial risk assessment scores -- 11.1 Interrelations between financial risk management and operational risk management -- 11.2 Financial rating systems and scoring systems -- 11.3 Data management for rating and scoring -- 11.4 Use case: business retail ratings for assessment of probabilities of default -- 11.5 Use case: quantitative financial ratings and prediction of fraud -- 11.6 Use case: money laundering and identification of the beneficial owner -- 11.7 Summary -- References.
12 Intelligent regulatory compliance Marcus Spies, Rolf Gubser and Markus Schacher -- 12.1 Introduction to standards and specifications for business governance -- 12.2 Specifications for implementing a framework for business governance -- 12.2.1 Business motivation model -- 12.2.2 Semantics of business vocabulary and business rules -- 12.3 Operational risk from a BMM/SBVR perspective -- 12.4 Intelligent regulatory compliance based on BMM and SBVR -- 12.4.1 Assessing influencers -- 12.4.2 Identify risks and potential rewards -- 12.4.3 Develop risk strategies -- 12.4.4 Implement risk strategy -- 12.4.5 Outlook: build adaptive IT systems -- 12.5 Generalization: capturing essential concepts of operational risk in UML and BMM -- 12.6 Summary -- References -- 13 Democratisation of enterprise risk management -- 13.1 Democratisation of advanced risk management services -- 13.2 Semantic-based technologies and enterprise-wide risk management -- 13.3 An enterprise-wide risk management vision -- 13.4 Integrated risk self-assessment: a service to attract customers -- 13.5 A real-life example in the telecommunications industry -- 13.6 Summary -- References -- 14 Operational risks, quality, accidents and incidents -- 14.1 The convergence of risk and quality management -- 14.2 Risks and the Taleb quadrants -- 14.3 The quality ladder -- 14.4 Risks, accidents and incidents -- 14.5 Operational risks in the oil and gas industry -- 14.6 Operational risks: data management, modelling and decision making -- 14.7 Summary -- References -- Index.
Abstract:
Models and methods for operational risks assessment and mitigation are gaining importance in financial institutions, healthcare organizations, industry, businesses and organisations in general. This book introduces modern Operational Risk Management and describes how various data sources of different types, both numeric and semantic sources such as text can be integrated and analyzed. The book also demonstrates how Operational Risk Management is synergetic to other risk management activities such as Financial Risk Management and Safety Management. Operational Risk Management: a practical approach to intelligent data analysis provides practical and tested methodologies for combining structured and unstructured, semantic-based data, and numeric data, in Operational Risk Management (OpR) data analysis. Key Features: The book is presented in four parts: 1) Introduction to OpR Management, 2) Data for OpR Management, 3) OpR Analytics and 4) OpR Applications and its Integration with other Disciplines. Explores integration of semantic, unstructured textual data, in Operational Risk Management. Provides novel techniques for combining qualitative and quantitative information to assess risks and design mitigation strategies. Presents a comprehensive treatment of "near-misses" data and incidents in Operational Risk Management. Looks at case studies in the financial and industrial sector. Discusses application of ontology engineering to model knowledge used in Operational Risk Management. Many real life examples are presented, mostly based on the MUSING project co-funded by the EU FP6 Information Society Technology Programme. It provides a unique multidisciplinary perspective on the important and evolving topic of Operational Risk Management. The book will be useful to operational risk practitioners, risk managers in banks, hospitals and industry
looking for modern approaches to risk management that combine an analysis of structured and unstructured data. The book will also benefit academics interested in research in this field, looking for techniques developed in response to real world problems.
Local Note:
Electronic reproduction. Ann Arbor, Michigan : ProQuest Ebook Central, 2017. Available via World Wide Web. Access may be limited to ProQuest Ebook Central affiliated libraries.
Genre:
Added Author:
Electronic Access:
Click to View