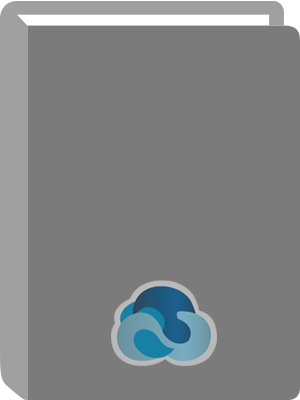
Enhance Your Business Applications : Simple Integration of Advanced Data Mining Functions.
Title:
Enhance Your Business Applications : Simple Integration of Advanced Data Mining Functions.
Author:
Redbooks, IBM.
Personal Author:
Physical Description:
1 online resource (348 pages)
Contents:
Front cover -- Contents -- Figures -- Tables -- Examples -- Notices -- Trademarks -- Preface -- The team that wrote this redbook -- Become a published author -- Comments welcome -- Part 1 Advanced data mining functions overview -- Chapter 1. Data mining functions in the database -- 1.1 The evolution of data mining -- 1.2 Data mining does not stand alone anymore -- 1.2.1 Faster time to market and closing the loop -- 1.2.2 Real-time analytics -- 1.2.3 Leveraging existing IT skills -- 1.2.4 Building repeatable processes and tasks -- 1.2.5 Efficiency and effectiveness -- 1.2.6 Cost reduction of mining analytics -- Chapter 2. Overview of the new data mining functions -- 2.1 Why relational database management system (RDBMS) functions -- 2.1.1 Easy use of automation and integration -- 2.1.2 Operational efficiency -- 2.1.3 Performance -- 2.1.4 Administrative efficiency -- 2.2 Scoring: Deploying data mining models -- 2.2.1 Scoring as an SQL extension -- 2.2.2 Batch mode and real time -- 2.2.3 Support for the new PMML 2.0 standard -- 2.2.4 Leveraging existing IT skills -- 2.3 Modeling: Building a mining model using SQL -- 2.3.1 Interoperability -- 2.3.2 Models in DB2 UDB -- 2.3.3 Support of the new PMML 2.0 standard -- 2.3.4 Required skills -- 2.4 Visualization: Understanding the data mining model -- 2.4.1 Interoperability -- 2.4.2 Choice in use -- 2.4.3 Multiplatform capability -- 2.4.4 Support of the new PMML 2.0 standard -- 2.4.5 Required skills -- 2.5 IM Modeling, Scoring, and Visualization interactions -- 2.5.1 The whole picture -- 2.5.2 Configurations -- 2.5.3 Positioning with Intelligent Miner for Data -- 2.6 Conclusion -- Chapter 3. Business scenario deployment examples -- 3.1 Customer profiling -- 3.1.1 Business benefits -- 3.2 Fraud detection -- 3.2.1 Business benefits -- 3.3 Campaign management -- 3.3.1 Business benefits.
3.4 Up-to-date promotion -- 3.4.1 Business benefits -- 3.5 Integrating the generic components -- 3.5.1 Generic environment and components -- 3.5.2 The method -- Part 2 Deploying data mining functions -- Chapter 4. Customer profiling example -- 4.1 The business issue -- 4.2 Mapping the business issue to data mining functions -- 4.3 The business application -- 4.4 Environment, components, and implementation flow -- 4.5 Step-by-step implementation -- 4.5.1 Configuration -- 4.5.2 Workbench data mining -- 4.5.3 Scoring -- 4.5.4 Application integration -- 4.6 Benefits -- 4.6.1 End-to-end implementation -- 4.6.2 DB2 mining functions next to the workbench -- 4.6.3 Real-time analytics -- 4.6.4 Automated and on demand for multi-channels -- Chapter 5. Fraud detection example -- 5.1 The business issue -- 5.2 Mapping the business issue to data mining functions -- 5.3 The business application -- 5.4 Environment, components, and implementation flow -- 5.5 Data to be used -- 5.5.1 Data extraction -- 5.5.2 Data manipulation and enrichment -- 5.6 Implementation in DB2 UDB V8.1 -- 5.6.1 Enabling database for modeling and scoring -- 5.6.2 Installing additional UDFs and stored procedures -- 5.6.3 Model building -- 5.7 Implementation in DB2 UDB V7.2 -- 5.7.1 Prerequisite: Initial model building -- 5.7.2 Data settings -- 5.7.3 Model parameter settings -- 5.7.4 Building the mining task -- 5.7.5 Running the model by calling a stored procedure -- 5.7.6 Scoring script generation -- 5.7.7 Applying the scoring model -- 5.7.8 Ranking and listing the five smallest clusters -- 5.7.9 Actionable result for investigation -- 5.7.10 Scheduling the job to run at regular intervals -- 5.8 Benefits -- 5.8.1 A system that adapts to changes in undesirable behavior -- 5.8.2 Fast deployment of fraud detection system -- 5.8.3 Better use of data mining resource.
5.8.4 A repeatable data mining process in a production environment -- 5.8.5 Enhanced communication -- 5.8.6 Leveraged IT skills for advanced analytical application -- 5.8.7 Actionable result -- Chapter 6. Campaign management solution examples -- 6.1 Campaign management overview -- 6.2 Trigger-based marketing -- 6.2.1 The business issue -- 6.2.2 Mapping the business issue to data mining functions -- 6.2.3 The business application -- 6.2.4 Environment, components, and implementation flow -- 6.2.5 Step-by-step implementation -- 6.2.6 Benefits -- 6.3 Retention campaign -- 6.3.1 The business issue -- 6.3.2 Mapping the business issue to data mining functions -- 6.3.3 The business application -- 6.3.4 Environment, components, and implementation flow -- 6.3.5 Step-by-step implementation -- 6.3.6 Benefits -- 6.4 Cross-selling campaign -- 6.4.1 The business issue -- 6.4.2 Mapping the business issue to data mining functions -- 6.4.3 The business application -- 6.4.4 Environment, components, and implementation flow -- 6.4.5 Step-by-step implementation -- 6.4.6 Other considerations -- Chapter 7. Up-to-date promotion example -- 7.1 The business issue -- 7.2 Mapping the business issue to data mining functions -- 7.3 The business application -- 7.4 Environment, components, and implementation flow -- 7.5 Step-by-step implementation -- 7.5.1 Configuration -- 7.5.2 Data model -- 7.5.3 Modeling -- 7.5.4 Application integration -- 7.6 Benefits -- 7.6.1 Automating models: Easy to use -- 7.6.2 Calibration: New data = new model -- Chapter 8. Other possibilities of integration -- 8.1 Real-time scoring on the Web (using Web analytics) -- 8.1.1 The business issue -- 8.1.2 Mapping the business issue to data mining functions -- 8.1.3 The business application -- 8.1.4 Integration with the application example -- 8.2 Business Intelligence integration.
8.2.1 Integration with DB2 OLAP -- 8.2.2 Integration with QMF -- 8.3 Integration with e-commerce -- 8.4 Integration with WebSphere Personalization -- 8.5 Integration using Java -- 8.5.1 Online scoring with IM Scoring Java Beans -- 8.5.2 Typical business issues -- 8.5.3 Mapping to mining functions using IM Scoring Java Beans -- 8.5.4 The business application -- 8.5.5 Integration with the application example -- 8.6 Conclusion -- Part 3 Configuring the DB2 functions for data mining -- Chapter 9. IM Scoring functions for existing mining models -- 9.1 Scoring functions -- 9.1.1 Scoring mining models -- 9.1.2 Scoring results -- 9.2 IM Scoring configuration steps -- 9.3 Step-by-step configuration -- 9.3.1 Configuring the DB2 UDB instance -- 9.3.2 Configuring the database -- 9.3.3 Exporting models from the modeling environment -- 9.3.4 Importing the data mining model in the relational database management system (RDBMS) -- 9.3.5 Scoring the data -- 9.3.6 Exploiting the results -- 9.4 Conclusion -- Chapter 10. Building the mining models using IM Modeling functions -- 10.1 IM Modeling functions -- 10.2 Data mining process with IM Modeling -- 10.3 Configuring a database for mining -- 10.3.1 Enabling the DB2 UDB instance for modeling -- 10.3.2 Configuring the individual database for modeling -- 10.3.3 IM Modeling in DB2 UDB V8.1 -- 10.4 Specifying mining data -- 10.4.1 Defining mining settings -- 10.4.2 Defining mining tasks -- 10.4.3 Building and storing mining models -- 10.4.4 Testing the classification models -- 10.4.5 Working with mining models and test results -- 10.5 Hybrid modeling -- 10.6 Conclusion -- Chapter 11. Using IM Visualization functions -- 11.1 IM Visualization functions -- 11.1.1 Common and different tasks -- 11.1.2 Applets or Java API -- 11.2 Configuration settings -- 11.2.1 Loading a model from a local file system.
11.2.2 Loading a model from a database -- 11.3 Using IM Visualizers -- 11.3.1 Using IM Visualizers as applets -- 11.3.2 Complete example script -- 11.4 Examples of IM Visualization -- Part 4 Appendixes -- Appendix A. SQL script to configure database for data mining function -- Appendix B. SQL scripts for the customer profiling scenario -- Script to create and load the customer segment table -- Script to score new customers -- Appendix C. SQL scripts for the fraud detection scenario -- Script to prepare the data -- Script to build the data mining model -- Script to score the data -- Script to get the scoring results -- Appendix D. SQL scripts for the retention campaign scenario -- Script to create a table -- Script to import the data mining model with PMML file -- Script to create a view of the resulting score -- Script to create a table with the resulting score -- Appendix E. SQL scripts for the up-to-date promotion scenario -- Script for function to build the associations rule model -- Script for a function that transforms the resulting rule model -- Script to build the rules model -- Script to extract rules to a table -- Appendix F. UDF to create data mining models -- Appendix G. UDF to extract rules from a model to a table -- Appendix H. Embedding an IM Visualization applet -- Syntax to embed the IM Visualization applet -- Parameters to use -- Appendix I. IM Scoring Java Bean code example -- Source code of IM Scoring Java Bean -- Setting up the environment variables: The paths.bat file -- Appendix J. Demographic clustering: Technical differences -- Appendix K. Additional material -- Locating the Web material -- Using the Web material -- System requirements for downloading the Web material -- How to use the Web material -- Glossary -- Abbreviations and acronyms -- Related publications -- IBM Redbooks -- Other resources -- Referenced Web sites.
How to get IBM Redbooks.
Local Note:
Electronic reproduction. Ann Arbor, Michigan : ProQuest Ebook Central, 2017. Available via World Wide Web. Access may be limited to ProQuest Ebook Central affiliated libraries.
Genre:
Electronic Access:
Click to View