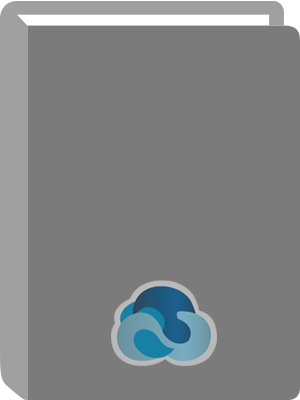
Advances in Multivariate Statistical Methods.
Title:
Advances in Multivariate Statistical Methods.
Author:
SenGupta, Ashis.
ISBN:
9789812838247
Personal Author:
Physical Description:
1 online resource (492 pages)
Series:
Statistical Science and Interdisciplinary Research, 4
Contents:
Contents -- Foreword -- Preface -- 1. High-Dimensional Discrete Statistical Models: UIP, MCP and CSI in Perspectives P. K. Sen -- 1.1. Introduction -- 1.2. Preliminary Notion -- 1.3. CATANOCOVA -- 1.4. UIP and CSI -- 1.5. Statistical Reasoning for HDDSM -- 1.6. UIP and MCP in HDDSM -- References -- 2. A Review of Multivariate Theory for High Dimensional Data with Fewer Observations M. S. Srivastava -- Contents -- 2.1. Introduction -- 2.2. Inference on the Mean Vector in One-Sample -- 2.2.1. Tests invariant under orthogonal and a non-zero scalar transformations -- 2.2.2. A Test invariant under scalar transformation of each component -- 2.2.3. Comparison of Power -- 2.3. Two-sample Tests -- 2.4. Multivariate Analysis of Variance (MANOVA) -- 2.5. Discriminant Analysis -- 2.6. Tests of Hypotheses on Covariance Matrices -- 2.6.1. One-sample case -- 2.6.1.1. Tests for the Sphericity Hypothesis -- 2.6.1.2. Testing that the covariance matrix is an identity matrix -- 2.6.1.3. Testing that the Covariance Matrix is a Diagonal Matrix -- 2.6.1.4. Testing that the covariance matrix is of intraclass correlation structure -- 2.6.1.5. Simulation results of power comparison and attained signifi- cance level -- 2.6.2. Two-Sample case -- 2.6.3. Testing the equality of covariances in MANOVA -- 2.7. Conclusion -- References -- 3. Model Based Penalized Clustering for Multivariate Data S. Ghosh and D. K. Dey -- 3.1. Introduction -- 3.2. Optimization Framework for Regularized K-means Clustering -- 3.3. Likelihood Formulation -- 3.4. Choices of Prior -- 3.5. Clustering Data Examples -- 3.5.1. Old faithful data: clustering analysis -- 3.5.2. Clustering result through correlation decomposition -- 3.5.3. Fisher's Iris Set: Clustering Analysis -- 3.6. Conclusion -- References -- 4. Jacobians Under Constraints and Statistical Bioinformatics K. V. Mardia -- Contents.
4.1. Introduction -- 4.2. Jacobians Under Constraints and the Haar Measure -- 4.3. The Euler-Angle Representation -- 4.4. The Jacobian -- 4.5. Discussion -- 4.6. Acknowledgements -- References -- 5. Cluster Validation for Microarray Data: An Appraisal V. Pihur, G. N. Brock and S. Datta -- Contents -- 5.1. Introduction -- 5.2. Validation Measures -- 5.2.1. Internal measures -- 5.2.1.1. Connectivity -- 5.2.1.2. Silhouette Width -- 5.2.1.3. Dunn Index -- 5.2.1.4. In-Group Proportion (IGP) -- 5.2.2. Stability measures -- 5.2.2.1. Average Proportion of Non-overlap (APN) -- 5.2.2.2. Average Distance (AD) -- 5.2.2.3. Average Distance between Means (ADM) -- 5.2.2.4. Figure of Merit (FOM) -- 5.2.3. Biological validation measures -- 5.2.3.1. Biological Homogeneity Index (BHI) -- 5.2.3.2. Biological Stability Index (BSI) -- 5.3. A Numerical Illustration -- 5.3.1. UPGMA -- 5.3.2. K-means -- 5.3.3. Diana -- 5.3.4. PAM -- 5.3.5. SOM -- 5.3.6. Model based clustering -- 5.3.7. Bayesian clustering -- 5.3.8. SOTA -- 5.3.9. Cluster validation results -- 5.4. Software for cluster validation -- 5.4.1. The clValid package -- 5.4.2. Other packages -- 5.5. Discussion -- References -- 6. Flexible Bivariate Circular Models B. C. Arnold and A. SenGupta -- Contents -- 6.1. Introduction -- 6.2. One Dimensional Circular Models -- 6.3. To Higher Dimensions Via Conditional Specification -- 6.4. Inference -- 6.5. Multivariate Extensions And Open Questions -- References -- 7. Optimal Text Space Representation of Student Essays Using Latent Semantic Analysis A. Villacorta and S. R. Jammalamadaka -- Contents -- 7.1. Introduction -- 7.2. Textual Decomposition and Circular Analysis -- 7.2.1. Vector space model and latent semantic analysis -- 7.2.2. Circular analysis -- 7.3. Optimal Vector Space Representation -- 7.3.1. Loss functions.
7.3.2. Testing hypotheses using circular analysis of variance -- 7.4. Results -- 7.4.1. Strict grade matching -- 7.4.2. Three level partition -- 7.4.3. Binary partitions -- 7.4.4. Comparison of orderings -- 7.4.5. Analysis of variance -- 7.5. Conclusions -- 7.6. Acknowledgements -- References -- 8. Linear Regression for Random Measures M. M. Rao -- Contents -- 8.1. Introduction -- 8.2. Conditioning Concepts and Regression -- 8.3. Regression for Random Measures -- 8.4. Regression for Random Integrals -- 8.5. Final Remarks -- References -- 9. Mixed Multivariate Models for Random Sums and Maxima T. J. Kozubowski, A. K. Panorska and F. Biondi -- Contents -- 9.1. Introduction -- 9.2. The BEG Model -- 9.2.1. Marginal distributions -- 9.2.2. Conditional distributions -- 9.2.2.1. The conditional distribution of (X -- N) given N > n -- 9.2.2.2. The conditional distribution of (X -- N) given X > u -- 9.2.3. Moments -- 9.2.4. Representations -- 9.2.5. Stability properties -- 9.2.6. Estimation -- 9.3. The BTLG Model -- 9.3.1. Marginal distributions -- 9.3.2. Conditional distributions -- 9.3.2.1. The conditional distribution of (Y -- N) given N > n -- 9.3.2.2. The conditional distribution of (Y -- N) given Y > u -- 9.3.3. Moments -- 9.3.4. Representations -- 9.3.5. Stability properties -- 9.3.6. Estimation -- 9.4. Extensions -- 9.4.1. The BGNB model -- 9.4.1.1. Conditional distributions -- 9.4.1.2. Moments and related parameters -- 9.4.1.3. Representations -- 9.4.2. The BGTLNB model -- 9.4.2.1. Conditional distributions -- 9.4.2.2. Moments and related parameters -- 9.4.2.3. Representations -- 9.5. Acknowledgements -- References -- 10. Estimation of the Multivariate Box-Cox Transformation Parameters M. Rahman and L. M. Pearson -- Contents -- 10.1. Introduction -- 10.2. Box-Cox Transformation -- 10.3. Maximum Likelihood Estimation Using The Newton-Raphson Method.
10.4. Maximization of the Multivariate Shapiro-WilkW Statistic -- 10.5. Simulation Study -- References -- 11. Generation of Multivariate Densities R. N. Rattihalli and A. N. Basugade -- Contents -- 11.1. Introduction -- 11.2. Generation of Densities by Contour Transformation -- 11.2.1. Bivariate densities obtained by two i.i.d. standard normal variates -- 11.2.2. General form of densities -- 11.2.3. Multivariate models obtained from circular contoured densities -- 11.3. Acknowledgement -- References -- 12. Smooth Estimation of Multivariate Distribution and Density Functions Y. P. Chaubey -- 12.1. Intoduction -- 12.2. Hille's Theorem: Univariate Case -- 12.2.1. Smooth estimation of Survival and Density Functions -- 12.3. Smooth Estimators of Other Functionals -- 12.3.1. Censored Data -- 12.3.2. Other Applications -- 12.3.3. Generalized Smoothing Lemma and Applications -- 12.4. Multivariate Generalization of Hille's Lemma -- 12.5. Further Developments -- 12.5.1. Quantile Estimation -- 12.5.2. Other Problems -- Acknowledgments -- References -- 13. Estimation Using Quantile Function Structure with Emphasis on Weibull Distribution G. D. Kollia, G. S. Mudholkar and D. K. Srivastava -- Contents -- 13.1. Introduction -- 13.2. The Approach in the General Setting -- 13.2.1. Construction of the pivotal statistics -- 13.2.2. The Jackknifed L-statistic and its variance estimate -- 13.2.3. The estimators -- 13.3. Estimation of Weibull Parameters -- 13.3.1. Closed form L-estimators -- 13.4. Comparisons -- 13.4.1. Competitors -- 13.4.2. A Monte Carlo experiment and results -- 13.5. Conclusions and Miscellaneous Remarks -- 13.6. Acknowledgments -- References -- 14. On Optimal Estimating Functions in the Presence of Nuisance Parameters P. Mukhopadhyay -- Contents -- 14.1. Introduction -- 14.2. An Optimal Estimating Function.
14.3. The Case of More Than One Nuisance Parameter -- 14.4. Examples -- References -- 15. Inference in Exponential Family Regression Models Under Certain Shape Constraints M. Banerjee -- Contents -- 15.1. Introduction and Background -- 15.1.1. Conditionally parametric response models: least squares and maximum likelihood estimates -- 15.2. Discrepancy Statistics for Testing The Null Hypothesis -- 15.2.1. Relevant stochastic processes and derived functionals -- 15.3. Limit Distributions for The Discrepancy Statistics and Methodological Implications -- 15.3.1. Incorporating further shape constraints -- 15.4. Concluding Discussion -- 15.5. Proof of Theorem 1 -- 15.6. Acknowledgements -- References -- 16. Study of Optimal Adaptive Rule in Testing Problem S. K. Bhandari, R. Dutta and R. G. Niyogi -- Contents -- 16.1. Introduction -- 16.2. Preliminaries and Main Results: Simple Hypothesis Case -- 16.2.1. Procedure I -- 16.2.2. Procedure II -- 16.2.3. Simulation studies (tables and results) -- 16.3. Appendix -- 16.4. Acknowledgement -- References -- 17. The G-IG Analogies and Robust Tests for Inverse Gaussian Scale Parameters G. S. Mudholkar, H. Wang and R. Natarajan -- Contents -- 17.1. Introduction -- 17.2. G-IG Analogies -- 17.3. Inferences Regarding Inverse Gaussian Scale Parameters -- 17.4. A Monte Carlo Study -- References -- 18. Clusterwise Regression Using Dirichlet Mixtures C. Kang and S. Ghosal -- Contents -- 18.1. Introduction -- 18.2. Method Description -- 18.3. Simulation Study -- 18.3.1. One dimension -- 18.3.2. Two dimension -- 18.3.3. Higher dimension -- 18.4. Conclusions -- 18.5. Acknowledgments -- References -- 19. Bayesian Analysis of Rank Data Using SIR A. K. Laha and S. Dongaonkar -- Contents -- 19.1. Introduction -- 19.2. One Transposition Error -- 19.3. Disjoint Transposition Errors -- 19.4. Cyclic Errors -- 19.5. The General Case.
19.6. Example.
Abstract:
This volume contains a collection of research articles on multivariate statistical methods, encompassing both theoretical advances and emerging applications in a variety of scientific disciplines. It serves as a tribute to Professor S N Roy, an eminent statistician who has made seminal contributions to the area of multivariate statistical methods, on his birth centenary. In the area of emerging applications, the topics include bioinformatics, categorical data and clinical trials, econometrics, longitudinal data analysis, microarray data analysis, sample surveys, statistical process control, etc. Researchers, professionals and advanced graduates will find the book an essential resource for modern developments in theory as well as for innovative and emerging important applications in the area of multivariate statistical methods.
Local Note:
Electronic reproduction. Ann Arbor, Michigan : ProQuest Ebook Central, 2017. Available via World Wide Web. Access may be limited to ProQuest Ebook Central affiliated libraries.
Genre:
Electronic Access:
Click to View