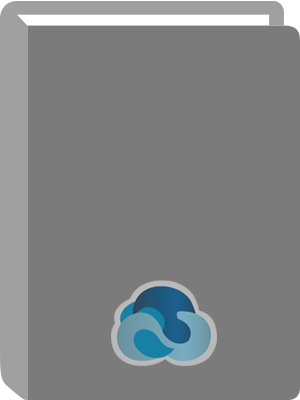
Fundamental Aspects of Operational Risk and Insurance Analytics : A Handbook of Operational Risk.
Title:
Fundamental Aspects of Operational Risk and Insurance Analytics : A Handbook of Operational Risk.
Author:
Cruz, Marcelo G.
ISBN:
9781118573020
Personal Author:
Edition:
1st ed.
Physical Description:
1 online resource (942 pages)
Series:
Wiley Handbooks in Financial Engineering and Econometrics
Contents:
Title Page -- Copyright Page -- Contents -- Preface -- Acronyms -- List of Distributions -- Chapter One OpRisk in Perspective -- 1.1 Brief History -- 1.2 Risk-Based Capital Ratios for Banks -- 1.3 The Basic Indicator and Standardized Approaches for OpRisk -- 1.4 The Advanced Measurement Approach -- 1.4.1 Internal Measurement Approach -- 1.4.2 Score Card Approach -- 1.4.3 Loss Distribution Approach -- 1.4.4 Requirements for AMA -- 1.5 General Remarks and Book Structure -- Chapter Two OpRisk Data and Governance -- 2.1 Introduction -- 2.2 OpRisk Taxonomy -- 2.2.1 Execution, Delivery, and Process Management -- 2.2.2 Clients, Products, and Business Practices -- 2.2.3 Business Disruption and System Failures -- 2.2.4 External Frauds -- 2.2.5 Internal Fraud -- 2.2.6 Employment Practices and Workplace Safety -- 2.2.7 Damage to Physical Assets -- 2.3 The Elements of the OpRisk Framework -- 2.3.1 Internal Loss Data -- 2.3.2 Setting a Collection Threshold and Possible Impacts -- 2.3.3 Completeness of Database (Under-reporting Events) -- 2.3.4 Recoveries and Near Misses -- 2.3.5 Time Period for Resolution of Operational Losses -- 2.3.6 Adding Costs to Losses -- 2.3.7 Provisioning Treatment of Expected Operational Losses -- 2.4 Business Environment and Internal Control Environment Factors (BEICFs) -- 2.4.1 Risk Control Self-Assessment (RCSA) -- 2.4.2 Key Risk Indicators -- 2.5 External Databases -- 2.6 Scenario Analysis -- 2.7 OpRisk Profile in Different Financial Sectors -- 2.7.1 Trading and Sales -- 2.7.2 Corporate Finance -- 2.7.3 Retail Banking -- 2.7.4 Insurance -- 2.7.5 Asset Management -- 2.7.6 Retail Brokerage -- 2.8 Risk Organization and Governance -- 2.8.1 Organization of Risk Departments -- 2.8.2 Structuring a Firm Wide Policy: Example of an OpRisk Policy -- 2.8.3 Governance -- Chapter Three Using OpRisk Data for Business Analysis.
3.1 Cost Reduction Programs in Financial Firms -- 3.2 Using OpRisk Data to Perform Business Analysis -- 3.2.1 The Risk of Losing Key Talents: OpRisk in Human Resources -- 3.2.2 OpRisk in Systems Development and Transaction Processing -- 3.3 Conclusions -- Chapter Four Stress-Testing OpRisk Capital and the Comprehensive Capital Analysis and Review (CCAR) -- 4.1 The Need for Stressing OpRisk Capital Even Beyond 99.9% -- 4.2 Comprehensive Capital Review and Analysis (CCAR) -- 4.3 OpRisk and Stress Tests -- 4.4 OpRisk in CCAR in Practice -- 4.5 Reverse Stress Test -- 4.6 Stressing OpRisk Multivariate Models-Understanding the Relationship Among Internal Control Factors and Their Impact on Operation -- Chapter Five Basic Probability Concepts in Loss Distribution Approach -- 5.1 Loss Distribution Approach -- 5.2 Quantiles and Moments -- 5.3 Frequency Distributions -- 5.4 Severity Distributions -- 5.4.1 Simple Parametric Distributions -- 5.4.2 Truncated Distributions -- 5.4.3 Mixture and Spliced Distributions -- 5.5 Convolutions and Characteristic Functions -- 5.6 Extreme Value Theory -- 5.6.1 EVT-Block Maxima -- 5.6.2 EVT-Random Number of Losses -- 5.6.3 EVT-Threshold Exceedances -- Chapter Six Risk Measures and Capital Allocation -- 6.1 Development of Capital Accords Base I, II and III -- 6.2 Measures of Risk -- 6.2.1 Coherent and Convex Risk Measures -- 6.2.2 Comonotonic Additive Risk Measures -- 6.2.3 Value-at-Risk -- 6.2.4 Expected Shortfall -- 6.2.5 Spectral Risk Measure -- 6.2.6 Higher-Order Risk Measures -- 6.2.7 Distortion Risk Measures -- 6.2.8 Elicitable Risk Measures -- 6.2.9 Risk Measure Accounting for Parameter Uncertainty -- 6.3 Capital Allocation -- 6.3.1 Coherent Capital Allocation -- 6.3.2 Euler Allocation -- 6.3.3 Standard Deviation -- 6.3.4 Expected Shortfall -- 6.3.5 Value-at-Risk -- 6.3.6 Allocation by Marginal Contributions.
6.3.7 Numerical Example -- Chapter Seven Estimation of Frequency and Severity Models -- 7.1 Frequentist Estimation -- 7.1.1 Parameteric Maximum Likelihood Method -- 7.1.2 Maximum Likelihood Method for Truncated and Censored Data -- 7.1.3 Expectation Maximization and Parameter Estimation -- 7.1.4 Bootstrap for Estimation of Parameter Accuracy -- 7.1.5 Indirect Inference-Based Likelihood Estimation -- 7.2 Bayesian Inference Approach -- 7.2.1 Conjugate Prior Distributions -- 7.2.2 Gaussian Approximation for Posterior (Laplace Type) -- 7.2.3 Posterior Point Estimators -- 7.2.4 Restricted Parameters -- 7.2.5 Noninformative Prior -- 7.3 Mean Square Error of Prediction -- 7.4 Standard Markov Chain Monte Carlo (MCMC) Methods -- 7.4.1 Motivation for Markov Chain Methods -- 7.4.2 Metropolis-Hastings Algorithm -- 7.4.3 Gibbs Sampler -- 7.4.4 Random Walk Metropolis-Hastings within Gibbs -- 7.5 Standard MCMC Guidelines for Implementation -- 7.5.1 Tuning, Burn-in, and Sampling Stages -- 7.5.2 Numerical Error -- 7.5.3 MCMC Extensions: Reducing Sample Autocorrelation -- 7.6 Advanced MCMC Methods -- 7.6.1 Auxiliary Variable MCMC Methods: Slice Sampling -- 7.6.2 Generic Univariate Auxiliary Variable Gibbs Sampler: Slice Sampler -- 7.6.3 Adaptive MCMC -- 7.6.4 Riemann-Manifold Hamiltonian Monte Carlo Sampler (Automated Local Adaption) -- 7.7 Sequential Monte Carlo (SMC) Samplers and Importance Sampling -- 7.7.1 Motivating OpRisk Applications for SMC Samplers -- 7.7.2 SMC Sampler Methodology and Components -- 7.7.3 Incorporating Partial Rejection Control into SMC Samplers -- 7.7.4 Finite Sample (Nonasymptotic) Accuracy for Particle Integration -- 7.8 Approximate Bayesian Computation (ABC) Methods -- 7.9 OpRisk Estimation and Modeling for Truncated Data -- 7.9.1 Constant Threshold - Poisson Process -- 7.9.2 Negative Binomial and Binomial Frequencies.
7.9.3 Ignoring Data Truncation -- 7.9.4 Threshold Varying in Time -- 7.9.5 Unknown and Stochastic Truncation Level -- Chapter Eight Model Selection and Goodness-of-Fit Testing for Frequency and Severity Models -- 8.1 Qualitative Model Diagnostic Tools -- 8.2 Tail Diagnostics -- 8.3 Information Criterion for Model Selection -- 8.3.1 Akaike Information Criterion for LDA Model Selection -- 8.3.2 Deviance Information Criterion -- 8.4 Goodness-of-Fit Testing for Model Choice (How to Account for Heavy Tails!) -- 8.4.1 Convergence Results of the Empirical Process for GOF Testing -- 8.4.2 Overview of Generic GOF Tests-Omnibus Distributional Tests -- 8.4.3 Kolmogorov-Smirnov Goodness-of-Fit Test and Weighted Variants: Testing in the Presence of Heavy Tails -- 8.4.4 Cramer-von-Mises Goodness-of-Fit Tests and Weighted Variants: Testing in the Presence of Heavy Tails -- 8.5 Bayesian Model Selection -- 8.5.1 Reciprocal Importance Sampling Estimator -- 8.5.2 Chib Estimator for Model Evidence -- 8.6 SMC Sampler Estimators of Model Evidence -- 8.7 Multiple Risk Dependence Structure Model Selection: Copula Choice -- 8.7.1 Approaches to Goodness-of-Fit Testing for Dependence Structures -- 8.7.2 Double Parameteric Bootstrap for Copula GOF -- Chapter Nine Flexible Parametric Severity Models: Basics -- 9.1 Motivation for Flexible Parametric Severity Loss Models -- 9.2 Context of Flexible Heavy-Tailed Loss Models in OpRisk and Insurance LDA Models -- 9.3 Empirical Analysis Justifying Heavy-Tailed Loss Models in OpRisk -- 9.4 Quantile Function Heavy-Tailed Severity Models -- 9.4.1 g-and-h Severity Model Family in OpRisk -- 9.4.2 Tail Properties of the g-and-h, g, h, and h-h Severity in OpRisk -- 9.4.3 Parameter Estimation for the g-and-h Severity in OpRisk -- 9.4.4 Bayesian Models for the g-and-h Severity in OpRisk.
9.5 Generalized Beta Family of Heavy-Tailed Severity Models -- 9.5.1 Generalized Beta Family Type II Severity Models in OpRisk -- 9.5.2 Sub families of the Generalized Beta Family Type II Severity Models -- 9.5.3 Mixture Representations of the Generalized Beta Family Type II Severity Models -- 9.5.4 Estimation in the Generalized Beta Family Type II Severity Models -- 9.6 Generalized Hyperbolic Families of Heavy-Tailed Severity Models -- 9.6.1 Tail Properties and Infinite Divisibility of the Generalized Hyperbolic Severity Models -- 9.6.2 Subfamilies of the Generalized Hyperbolic Severity Models -- 9.6.3 Normal Inverse Gaussian Family of Heavy-Tailed Severity Models -- 9.7 Halphen Family of Flexible Severity Models: GIG and Hyperbolic -- 9.7.1 Halphen Type A: Generalized Inverse Gaussian Family of Flexible Severity Models -- 9.7.2 Halphen Type B and IB Families of Flexible Severity Models -- Chapter Ten Dependence Concepts -- 10.1 Introduction to Concepts in Dependence for OpRisk and Insurance -- 10.2 Dependence Modeling Within and Between LDA Model Structures -- 10.2.1 Where Can One Introduce Dependence Between LDA Model Structures? -- 10.2.2 Understanding Basic Impacts of Dependence Modeling Between LDA Components in Multiple Risks -- 10.3 General Notions of Dependence -- 10.4 Dependence Measures -- 10.4.1 Linear Correlation -- 10.4.2 Rank Correlation Measures -- 10.5 Tail Dependence Parameters, Functions, and Tail Order Functions -- 10.5.1 Tail Dependence Coefficients -- 10.5.2 Tail Dependence Functions and Orders -- 10.5.3 A Link Between Orthant Extreme Dependence and Spectral Measures: Tail Dependence -- Chapter Eleven Dependence Models -- 11.1 Introduction to Parametric Dependence Modeling Through a Copula -- 11.2 Copula Model Families for OpRisk -- 11.2.1 Gaussian Copula -- 11.2.2 t-Copula -- 11.2.3 Archimedean Copulas.
11.2.4 Archimedean Copula Generators and the Laplace Transform of a Non-Negative Random Variable.
Abstract:
A one-stop guide for the theories, applications, and statistical methodologies essential to operational risk Providing a complete overview of operational risk modeling and relevant insurance analytics, Fundamental Aspects of Operational Risk and Insurance Analytics: A Handbook of Operational Risk offers a systematic approach that covers the wide range of topics in this area. Written by a team of leading experts in the field, the handbook presents detailed coverage of the theories, applications, and models inherent in any discussion of the fundamentals of operational risk, with a primary focus on Basel II/III regulation, modeling dependence, estimation of risk models, and modeling the data elements. Fundamental Aspects of Operational Risk and Insurance Analytics: A Handbook of Operational Risk begins with coverage on the four data elements used in operational risk framework as well as processing risk taxonomy. The book then goes further in-depth into the key topics in operational risk measurement and insurance, for example diverse methods to estimate frequency and severity models. Finally, the book ends with sections on specific topics, such as scenario analysis; multifactor modeling; and dependence modeling. A unique companion with Advances in Heavy Tailed Risk Modeling: A Handbook of Operational Risk, the handbook also features: Discussions on internal loss data and key risk indicators, which are both fundamental for developing a risk-sensitive framework Guidelines for how operational risk can be inserted into a firm's strategic decisions A model for stress tests of operational risk under the United States Comprehensive Capital Analysis and Review (CCAR) program A valuable reference for financial engineers, quantitative analysts, risk managers, and large-scale consultancy groups advising banks on their internal systems, the handbook is
also useful for academics teaching postgraduate courses on the methodology of operational risk.
Local Note:
Electronic reproduction. Ann Arbor, Michigan : ProQuest Ebook Central, 2017. Available via World Wide Web. Access may be limited to ProQuest Ebook Central affiliated libraries.
Genre:
Electronic Access:
Click to View