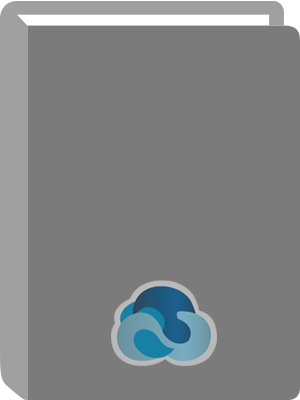
Data Analysis : A Bayesian Tutorial.
Title:
Data Analysis : A Bayesian Tutorial.
Author:
Sivia, Devinderjit.
ISBN:
9780191546709
Personal Author:
Edition:
2nd ed.
Physical Description:
1 online resource (259 pages)
Contents:
Contents -- PART I: THE ESSENTIALS -- 1. The basics -- 1.1 Introduction: deductive logic versus plausible reasoning -- 1.2 Probability: Cox and the rules for consistent reasoning -- 1.3 Corollaries: Bayes' theorem and marginalization -- 1.4 Some history: Bayes, Laplace and orthodox statistics -- 1.5 Outline of book -- 2. Parameter estimation I -- 2.1 Example 1: is this a fair coin? -- 2.2 Reliabilities: best estimates, error-bars and confidence intervals -- 2.3 Example 2: Gaussian noise and averages -- 2.4 Example 3: the lighthouse problem -- 3. Parameter estimation II -- 3.1 Example 4: amplitude of a signal in the presence of background -- 3.2 Reliabilities: best estimates, correlations and error-bars -- 3.3 Example 5: Gaussian noise revisited -- 3.4 Algorithms: a numerical interlude -- 3.5 Approximations: maximum likelihood and least-squares -- 3.6 Error-propagation: changing variables -- 4. Model selection -- 4.1 Introduction: the story of Mr A and Mr B -- 4.2 Example 6: how many lines are there? -- 4.3 Other examples: means, variance, dating and so on -- 5. Assigning probabilities -- 5.1 Ignorance: indifference and transformation groups -- 5.2 Testable information: the principle of maximum entropy -- 5.3 MaxEnt examples: some common pdfs -- 5.4 Approximations: interconnections and simplifications -- 5.5 Hangups: priors versus likelihoods -- PART II: ADVANCED TOPICS -- 6. Non-parametric estimation -- 6.1 Introduction: free-form solutions -- 6.2 MaxEnt: images, monkeys and a non-uniform prior -- 6.3 Smoothness: fuzzy pixels and spatial correlations -- 6.4 Generalizations: some extensions and comments -- 7. Experimental design -- 7.1 Introduction: general issues -- 7.2 Example 7: optimizing resolution functions -- 7.3 Calibration, model selection and binning -- 7.4 Information gain: quantifying the worth of an experiment.
8. Least-squares extensions -- 8.1 Introduction: constraints and restraints -- 8.2 Noise scaling: a simple global adjustment -- 8.3 Outliers: dealing with erratic data -- 8.4 Background removal -- 8.5 Correlated noise: avoiding over-counting -- 8.6 Log-normal: least-squares for magnitude data -- 9. Nested sampling -- 9.1 Introduction: the computational problem -- 9.2 Nested sampling: the basic idea -- 9.3 Generating a new object by random sampling -- 9.4 Monte Carlo sampling of the posterior -- 9.5 How many objects are needed? -- 9.6 Simulated annealing -- 10. Quantification -- 10.1 Exploring an intrinsically non-uniform prior -- 10.2 Example: ON/OFF switching -- 10.3 Estimating quantities -- 10.4 Final remarks -- A. Gaussian integrals -- A.1 The univariate case -- A.2 The bivariate extension -- A.3 The multivariate generalization -- B. Cox's derivation of probability -- B.1 Lemma 1: associativity equation -- B.2 Lemma 2: negation -- Bibliography -- Index -- A -- B -- C -- D -- E -- F -- G -- H -- I -- J -- K -- L -- M -- N -- O -- P -- Q -- R -- S -- T -- U -- V -- W -- X.
Abstract:
One of the strengths of this book is the author's ability to motivate the use of Bayesian methods through simple yet effective examples. - Katie St. Clair MAA Reviews.
Local Note:
Electronic reproduction. Ann Arbor, Michigan : ProQuest Ebook Central, 2017. Available via World Wide Web. Access may be limited to ProQuest Ebook Central affiliated libraries.
Genre:
Added Author:
Electronic Access:
Click to View