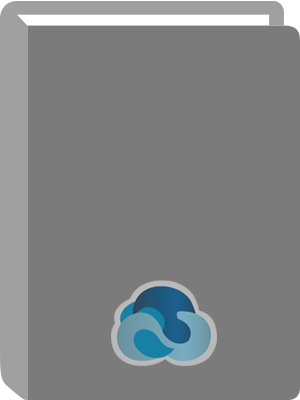
Least Squares Support Vector Machines.
Title:
Least Squares Support Vector Machines.
Author:
Suykens, Johan A. K.
ISBN:
9789812776655
Personal Author:
Physical Description:
1 online resource (310 pages)
Contents:
Contents -- Preface -- Chapter 1 Introduction -- 1.1 Multilayer perceptron neural networks -- 1.2 Regression and classification -- 1.3 Learning and generalization -- 1.3.1 Weight decay and effective number of parameters -- 1.3.2 Ridge regression -- 1.3.3 Bayesian learning -- 1.4 Principles of pattern recognition -- 1.4.1 Bayes rule and optimal classifier under Gaussian assumptions -- 1.4.2 Receiver operating characteristic -- 1.5 Dimensionality reduction methods -- 1.6 Parametric versus non-parametric approaches and RBF networks -- 1.7 Feedforward versus recurrent network models -- Chapter 2 Support Vector Machines -- 2.1 Maximal margin classification and linear SVMs -- 2.1.1 Margin -- 2.1.2 Linear SVM classifier: separable case -- 2.1.3 Linear SVM classifier: non-separable case -- 2.2 Kernel trick and Mercer condition -- 2.3 Nonlinear SVM classifiers -- 2.4 VC theory and structural risk minimization -- 2.4.1 Empirical risk versus generalization error -- 2.4.2 Structural risk minimization -- 2.5 SVMs for function estimation -- 2.5.1 SVM for linear function estimation -- 2.5.2 SVM for nonlinear function estimation -- 2.5.3 VC bound on generalization error -- 2.6 Modifications and extensions -- 2.6.1 Kernels -- 2.6.2 Extension to other convex cost functions -- 2.6.3 Algorithms -- 2.6.4 Parametric versus non-parametric approaches -- Chapter 3 Basic Methods of Least Squares Support Vector Machines -- 3.1 Least Squares Support Vector Machines for classification -- 3.2 Multi-class formulations -- 3.3 Link with Fisher discriminant analysis in feature space -- 3.3.1 Linear Fisher discriminant analysis -- 3.3.2 Fisher discriminant analysis in feature space -- 3.4 Solving the LS-SVM KKT system -- 3.4.1 Conjugate gradient iterative methods -- 3.4.2 LS-SVM classifiers: UCI benchmarking results.
3.5 Least Squares Support Vector Machines for function estimation -- 3.6 Links with regularization networks and Gaussian processes -- 3.6.1 RKHS and reproducing property -- 3.6.2 Representer theorem and regularization networks -- 3.6.3 Gaussian processes -- 3.7 Sparseness by pruning -- Chapter 4 Bayesian Inference for LS-SVM Models -- 4.1 Bayesian inference for LS-SVM classifiers -- 4.1.1 Definition of network model parameters and hyperparameters -- 4.1.2 Bayes rule and levels of inference -- 4.1.3 Probabilistic interpretation of LS-SVM classifiers (Level 1) -- 4.1.4 Inference of the hyperparameters (Level 2) -- 4.1.5 Inference of kernel parameters and model comparison (Level 3) -- 4.1.6 Design of the LS-SVM classifier in the Bayesian evidence framework -- 4.1.7 Example and benchmarking results -- 4.2 Bayesian inference for LS-SVM regression -- 4.2.1 Probabilistic interpretation of LS-SVM regressors (Level 1): predictive mean and error bars -- 4.2.2 Inference of hyperparameters (Level 2) -- 4.2.3 Inference of kernel parameters and model comparison (Level 3) -- 4.3 Input selection by automatic relevance determination -- Chapter 5 Robustness -- 5.1 Noise model assumptions and robust statistics -- 5.2 Weighted LS-SVMs -- 5.3 Robust cross-validation -- 5.3.1 M-Estimators -- 5.3.2 L-Estimators -- 5.3.3 Efficiency-robustness trade-off -- 5.3.4 A robust and efficient cross-validation score function -- Chapter 6 Large Scale Problems -- 6.1 Low rank approximation methods -- 6.1.1 Nystrom method -- 6.1.2 Incomplete Cholesky factorization -- 6.2 Fixed Size LS-SVMs -- 6.2.1 Estimation in primal weight space -- 6.2.2 Active selection of support vectors -- 6.3 Basis construction in the feature space -- 6.4 Combining submodels -- 6.4.1 Committee network approach -- 6.4.2 Multilayer networks of LS-SVMs.
Chapter 7 LS-SVM for Unsupervised Learning -- 7.1 Support Vector Machines and linear PCA analysis -- 7.1.1 Classical principal component analysis formulation -- 7.1.2 Support vector machine formulation to linear PCA -- 7.1.3 Including a bias term -- 7.1.4 The reconstruction problem -- 7.2 An LS-SVM approach to kernel PCA -- 7.3 Links with density estimation -- 7.4 Kernel CCA -- 7.4.1 Classical canonical correlation analysis formulation -- 7.4.2 Support vector machine formulation to linear CCA -- 7.4.3 Extension to kernel CCA -- Chapter 8 LS-SVM for Recurrent Networks and Control -- 8.1 Recurrent Least Squares Support Vector Machines -- 8.1.1 From feedforward to recurrent LS-SVM formulations -- 8.1.2 A simplification to the problem -- 8.1.3 Example: trajectory learning of chaotic systems -- 8.2 LS-SVMs and optimal control -- 8.2.1 The N-stage optimal control problem -- 8.2.2 LS-SVMs as controllers within the N-stage optimal control problem -- 8.2.3 Alternative formulation and stability issues -- 8.2.4 Illustrative examples -- Appendix A -- Bibliography -- List of Symbols -- Acronyms -- Index.
Abstract:
This book focuses on Least Squares Support Vector Machines (LS-SVMs) which are reformulations to standard SVMs. LS-SVMs are closely related to regularization networks and Gaussian processes but additionally emphasize and exploit primal-dual interpretations from optimization theory. The authors explain the natural links between LS-SVM classifiers and kernel Fisher discriminant analysis. Bayesian inference of LS-SVM models is discussed, together with methods for imposing sparseness and employing robust statistics. The framework is further extended towards unsupervised learning by considering PCA analysis and its kernel version as a one-class modelling problem. This leads to new primal-dual support vector machine formulations for kernel PCA and kernel CCA analysis. Furthermore, LS-SVM formulations are given for recurrent networks and control. In general, support vector machines may pose heavy computational challenges for large data sets. For this purpose, a method of fixed size LS-SVM is proposed where the estimation is done in the primal space in relation to a Nyström sampling with active selection of support vectors. The methods are illustrated with several examples. Sample Chapter(s). Introduction (330 KB). Contents: Support Vector Machines; Basic Methods of Least Squares Support Vector Machines; Bayesian Inference for LS-SVM Models; Robustness; Large Scale Problems; LS-SVM for Unsupervised Learning; LS-SVM for Recurrent Networks and Control. Readership: Graduate students and researchers in neural networks; machine learning; data-mining; signal processing; circuit, systems and control theory; pattern recognition; and statistics.
Local Note:
Electronic reproduction. Ann Arbor, Michigan : ProQuest Ebook Central, 2017. Available via World Wide Web. Access may be limited to ProQuest Ebook Central affiliated libraries.
Genre:
Electronic Access:
Click to View