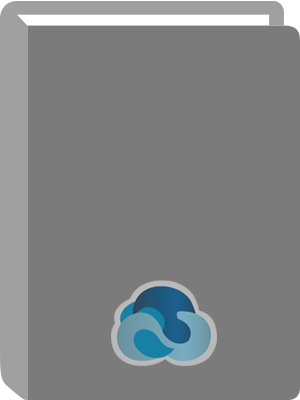
Biomolecular Networks : Methods and Applications in Systems Biology.
Title:
Biomolecular Networks : Methods and Applications in Systems Biology.
Author:
Chen, Luonan.
ISBN:
9780470488058
Personal Author:
Edition:
1st ed.
Physical Description:
1 online resource (420 pages)
Series:
Wiley Series in Bioinformatics Ser. ; v.10
Wiley Series in Bioinformatics Ser.
Contents:
BIOMOLECULAR NETWORKS -- CONTENTS -- PREFACE -- ACKNOWLEDGMENTS -- LIST OF ILLUSTRATIONS -- ACRONYMS -- 1 Introduction -- 1.1 Basic Concepts in Molecular Biology -- 1.1.1 Genomes, Genes, and DNA Replication Process -- 1.1.2 Transcription Process for RNA Synthesis -- 1.1.3 Translation Process for Protein Synthesis -- 1.2 Biomolecular Networks in Cells -- 1.3 Network Systems Biology -- 1.4 About This Book -- I GENE NETWORKS -- 2 Transcription Regulation: Networks and Models -- 2.1 Transcription Regulation and Gene Expression -- 2.1.1 Transcription and Gene Regulation -- 2.1.2 Microarray Experiments and Databases -- 2.1.3 ChIP-Chip Technology and Transcription Factor Databases -- 2.2 Networks in Transcription Regulation -- 2.3 Nonlinear Models Based on Biochemical Reactions -- 2.4 Integrated Models for Regulatory Networks -- 2.5 Summary -- 3 Reconstruction of Gene Regulatory Networks -- 3.1 Mathematical Models of Gene Regulatory Network -- 3.1.1 Boolean Networks -- 3.1.2 Bayesian Networks -- 3.1.3 Markov Networks -- 3.1.4 Differential Equations -- 3.2 Reconstructing Gene Regulatory Networks -- 3.2.1 Singular Value Decomposition -- 3.2.2 Model-Based Optimization -- 3.3 Inferring Gene Networks from Multiple Datasets -- 3.3.1 General Solutions and a Particular Solution of Network Structures for Multiple Datasets -- 3.3.2 Decomposition Algorithm -- 3.3.3 Numerical Validation -- 3.4 Gene Network-Based Drug Target Identification -- 3.4.1 Network Identification Methods -- 3.4.2 Linear Programming Framework -- 3.5 Summary -- 4 Inference of Transcriptional Regulatory Networks -- 4.1 Predicting TF Binding Sites and Promoters -- 4.2 Inference of Transcriptional Interactions -- 4.2.1 Differential Equation Methods -- 4.2.2 Bayesian Approaches -- 4.2.3 Data Mining and Other Methods -- 4.3 Identifying Combinatorial Regulations of TFs.
4.4 Inferring Cooperative Regulatory Networks -- 4.4.1 Mathematical Models -- 4.4.2 Estimating TF Activity -- 4.4.3 Linear Programming Models -- 4.4.4 Numerical Validation -- 4.5 Prediction of Transcription Factor Activity -- 4.5.1 Matrix Factorization -- 4.5.2 Nonlinear Models -- 4.6 Summary -- II PROTEIN INTERACTION NETWORKS -- 5 Prediction of Protein-Protein Interactions -- 5.1 Experimental Protein-Protein Interactions -- 5.2 Prediction of Protein-Protein Interactions -- 5.2.1 Association Methods -- 5.2.2 Maximum-Likelihood Estimation -- 5.2.3 Deterministic Optimization Approaches -- 5.3 Protein Interaction Prediction Based on Multidomain Pairs -- 5.3.1 Cooperative Domains, Strongly Cooperative Domains, Superdomains -- 5.3.2 Inference of Multidomain Interactions -- 5.3.3 Numerical Validation -- 5.3.4 Reconstructing Complexes by Multidomain Interactions -- 5.4 Domain Interaction Prediction Methods -- 5.4.1 Statistical Method -- 5.4.2 Domain Pair Exclusion Analysis -- 5.4.3 Parsimony Explanation Approaches -- 5.4.4 Integrative Approaches -- 5.5 Summary -- 6 Topological Structure of Biomolecular Networks -- 6.1 Statistical Properties of Biomolecular Networks -- 6.2 Evolution of Protein Interaction Networks -- 6.3 Hubs, Motifs, and Modularity in Biomolecular Networks -- 6.3.1 Network Centralities and Hubs -- 6.3.2 Network Modularity and Motifs -- 6.4 Explorative Roles of Hubs and Network Motifs -- 6.4.1 Dynamic Modularity Organized by Hubs and Network Motifs -- 6.4.2 Network Motifs Acting as Connectors between Pathways -- 6.5 Modularity Evaluation of Biomolecular Networks -- 6.5.1 Modularity Density D -- 6.5.2 Improving Module Resolution Limits by D -- 6.5.3 Equivalence between D and Kernel k Means -- 6.5.4 Extension of D to General Criteria: D(λ) and D(w) -- 6.5.5 Numerical Validation -- 6.6 Summary -- 7 Alignment of Biomolecular Networks.
7.1 Biomolecular Networks from Multiple Species -- 7.2 Pairwise Alignment of Biomolecular Networks -- 7.2.1 Score-Based Algorithms -- 7.2.2 Evolution-Guided Method -- 7.2.3 Graph Matching Algorithm -- 7.3 Network Alignment by Mathematical Programming -- 7.3.1 Integer Programming Formulation -- 7.3.2 Components of the Integer Quadratic Programming Approach -- 7.3.3 Numerical Validation -- 7.4 Multiple Alignment of Biomolecular Networks -- 7.5 Subnetwork and Pathway Querying -- 7.6 Summary -- 8 Network-Based Prediction of Protein Function -- 8.1 Protein Function and Annotation -- 8.2 Protein Functional Module Detection -- 8.2.1 Distance-Based Clustering Methods -- 8.2.2 Graph Clustering Methods -- 8.2.3 Validation of Module Detection -- 8.3 Functional Linkage for Protein Function Annotation -- 8.3.1 Bayesian Approach -- 8.3.2 Hopfield Network Method -- 8.3.3 p-Value Method -- 8.3.4 Statistical Framework -- 8.4 Protein Function Prediction from High-Throughput Data -- 8.4.1 Neighborhood Approaches -- 8.4.2 Optimization Methods -- 8.4.3 Probabilistic Methods -- 8.4.4 Machine Learning Techniques -- 8.5 Function Annotation Methods for Domains -- 8.5.1 Domain Sources -- 8.5.2 Integration of Heterogeneous Data -- 8.5.3 Domain Function Prediction -- 8.5.4 Numerical Validation -- 8.6 Summary -- III METABOLIC NETWORKS AND SIGNALING NETWORKS -- 9 Metabolic Networks: Analysis, Reconstruction, and Application -- 9.1 Cellular Metabolism and Metabolic Pathways -- 9.2 Metabolic Network Analysis and Modeling -- 9.2.1 Flux Balance Analysis -- 9.2.2 Elementary Mode and Extreme Pathway Analysis -- 9.2.3 Modeling Metabolic Networks -- 9.3 Reconstruction of Metabolic Networks -- 9.3.1 Pathfinding Based on Reactions and Compounds -- 9.3.2 Stoichiometric Approaches Based on Flux Profiles -- 9.3.3 Inferring Biochemical Networks from Timecourse Data.
9.4 Drug Target Detection in Metabolic Networks -- 9.4.1 Drug Target Detection Problem -- 9.4.2 Integer Linear Programming Model -- 9.4.3 Numerical Validation -- 9.5 Summary -- 10 Signaling Networks: Modeling and Inference -- 10.1 Signal Transduction in Cellular Systems -- 10.2 Modeling of Signal Transduction Pathways -- 10.2.1 Differential Equation Models -- 10.2.2 Petri Net Models -- 10.3 Inferring Signaling Networks from High-Throughput Data -- 10.3.1 NetSearch Method -- 10.3.2 Ordering Signaling Components -- 10.3.3 Color-Coding Methods -- 10.4 Inferring Signaling Networks by Linear Programming -- 10.4.1 Integer Linear Programming Model -- 10.4.2 Significance Measures -- 10.4.3 Numerical Validation -- 10.4.4 Inferring Signaling Networks by Network Flow Models -- 10.5 Inferring Signaling Networks from Experimental Evidence -- 10.6 Summary -- 11 Other Topics and New Trends -- 11.1 Network-Based Protein Structural Analysis -- 11.2 Integration of Biomolecular Networks -- 11.3 Posttranscriptional Regulation of Noncoding RNAs -- 11.4 Biomolecular Interactions and Human Diseases -- 11.5 Summary -- REFERENCES -- INDEX.
Abstract:
LUONAN CHEN, PhD, is a full professor in the Department of Electrical Engineering and Electronics, Osaka Sangyo University, Osaka, Japan, and he is also the founding director of Institute of Systems Biology, Shanghai University, Shanghai, China. Dr. Chen's fields of interest include systems biology, bioinformatics, and nonlinear dynamics. RUI-SHENG WANG, PhD, is an assistant professor in the School of Information, Renmin University of China. Dr. Wang's research interests include bioinformatics, computational systems biology, and complex networks. XIANG-SUN ZHANG is a full research professor in the Institute of Applied Math-ematics, Chinese Academy of Sciences. Professor Zhang's research interests include bioinformatics, systems biology, optimization theory, and related computational mathematics.
Local Note:
Electronic reproduction. Ann Arbor, Michigan : ProQuest Ebook Central, 2017. Available via World Wide Web. Access may be limited to ProQuest Ebook Central affiliated libraries.
Genre:
Electronic Access:
Click to View