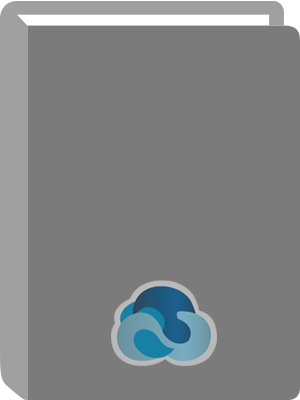
Data Mining in Drug Discovery.
Title:
Data Mining in Drug Discovery.
Author:
Kubinyi, Hugo.
ISBN:
9783527656011
Personal Author:
Edition:
1st ed.
Physical Description:
1 online resource (347 pages)
Series:
Methods and Principles in Medicinal Chemistry Ser. ; v.57
Methods and Principles in Medicinal Chemistry Ser.
Contents:
Data Mining in Drug Discovery -- Contents -- List of Contributors -- Preface -- A Personal Foreword -- Part One: Data Sources -- 1 Protein Structural Databases in Drug Discovery -- 1.1 The Protein Data Bank: The Unique Public Archive of Protein Structures -- 1.1.1 History and Background: AWealthy Resource for Structure-Based Computer-Aided Drug Design -- 1.1.2 Content, Format, and Quality of Data: Pitfalls and Challenges When Using PDB Files -- 1.1.2.1 The Content -- 1.1.2.2 The Format -- 1.1.2.3 The Quality and Uniformity of Data -- 1.2 PDB-Related Databases for Exploring Ligand-Protein Recognition -- 1.2.1 Databases in Parallel to the PDB -- 1.2.2 Collection of Binding Affinity Data -- 1.2.3 Focus on Protein-Ligand Binding Sites -- 1.3 The sc-PDB, a Collection of Pharmacologically Relevant Protein-Ligand Complexes -- 1.3.1 Database Setup and Content -- 1.3.2 Applications to Drug Design -- 1.3.2.1 Protein-Ligand Docking -- 1.3.2.2 Binding Site Detection and Comparisons -- 1.3.2.3 Prediction of Protein Hot Spots -- 1.3.2.4 Relationships between Ligands and Their Targets -- 1.3.2.5 Chemogenomic Screening for Protein-Ligand Fingerprints -- 1.4 Conclusions -- References -- 2 Public Domain Databases for Medicinal Chemistry -- 2.1 Introduction -- 2.2 Databases of Small Molecule Binding and Bioactivity -- 2.2.1 BindingDB -- 2.2.1.1 History, Focus, and Content -- 2.2.1.2 Browsing, Querying, and Downloading Capabilities -- 2.2.1.3 Linking with Other Databases -- 2.2.1.4 Special Tools and Data Sets -- 2.2.2 ChEMBL -- 2.2.2.1 History, Focus, and Content -- 2.2.2.2 Browsing, Querying, and Downloading Capabilities -- 2.2.2.3 Linking with Other Databases -- 2.2.2.4 Special Tools and Data Sets -- 2.2.3 PubChem -- 2.2.3.1 History, Focus, and Content -- 2.2.3.2 Browsing, Querying, and Downloading Capabilities -- 2.2.3.3 Linking with Other Databases.
2.2.3.4 Special Tools and Data Sets -- 2.2.4 Other Small Molecule Databases of Interest -- 2.3 Trends in Medicinal Chemistry Data -- 2.4 Directions -- 2.4.1 Strengthening the Databases -- 2.4.1.1 Coordination among Databases -- 2.4.1.2 Data Quality -- 2.4.1.3 Linking Journals and Databases -- 2.4.2 Next-Generation Capabilities -- 2.5 Summary -- References -- 3 Chemical Ontologies for Standardization, Knowledge Discovery, and Data Mining -- 3.1 Introduction -- 3.2 Background -- 3.2.1 The OBO Foundry: Ontologies in Biology and Medicine -- 3.2.2 Ontology Languages and Logical Expressivity -- 3.2.3 Ontology Interoperability and Upper-Level Ontologies -- 3.3 Chemical Ontologies -- 3.4 Standardization -- 3.5 Knowledge Discovery -- 3.6 Data Mining -- 3.7 Conclusions -- References -- 4 Building a Corporate Chemical Database Toward Systems Biology -- 4.1 Introduction -- 4.2 Setting the Scene -- 4.2.1 Concept of Molecule, Substance, and Batch -- 4.2.2 Challenge of Registering Diverse Data -- 4.3 Dealing with Chemical Structures -- 4.3.1 Chemical Cartridges -- 4.3.2 Uniqueness of Records -- 4.3.3 Use of Enhanced Stereochemistry -- 4.4 Increased Accuracy of the Registration of Data -- 4.4.1 Establishing Drawing Rules for Scientists -- 4.4.2 Standardization of Compound Representation -- 4.4.3 Three Roles and Two Staging Areas -- 4.4.4 Batch Reassignment -- 4.4.4.1 Unknown Compounds Management -- 4.4.5 Automatic Processes -- 4.5 Implementation of the Platform -- 4.5.1 Database -- 4.5.2 Software -- 4.5.3 Data Migration and Transformation of Names into Structures -- 4.6 Linking Chemical Information to Analytical Data -- 4.7 Linking Chemicals to Bioactivity Data -- 4.8 Conclusions -- References -- Part Two: Analysis and Enrichment -- 5 Data Mining of Plant Metabolic Pathways -- 5.1 Introduction -- 5.1.1 The Importance of Understanding Plant Metabolic Pathways.
5.1.2 Pathway Modeling and Its Prerequisites -- 5.2 Pathway Representation -- 5.2.1 Compounds -- 5.2.1.1 The Importance of Having Uniquely Defined Molecules -- 5.2.1.2 Representation Formats -- 5.2.1.3 Key Chemical Compound Databases -- 5.2.2 Reactions -- 5.2.2.1 Definitions of Reactions -- 5.2.2.2 Importance of Stoichiometry and Mass Balance -- 5.2.2.3 Atom Tracing -- 5.2.2.4 Storing Enzyme Information: EC Numbers and Their Limitations -- 5.2.3 Pathways -- 5.2.3.1 How Are Pathways Defined? -- 5.2.3.2 Typical Size and Distinction between Pathways and Superpathways -- 5.3 Pathway Management Platforms -- 5.3.1 Kyoto Encyclopedia of Genes and Genomes (KEGG) -- 5.3.1.1 Database Structure in KEGG -- 5.3.1.2 Navigation through KEGG -- 5.3.2 The Pathway Tools Platform -- 5.3.2.1 Database Management in Pathway Tools -- 5.3.2.2 Content Creation and Management with Pathway Tools -- 5.3.2.3 Pathway Tools' Visualization Capability -- 5.4 Obtaining Pathway Information -- 5.4.1 "Ready-Made" Reference Pathway Databases and Their Contents -- 5.4.1.1 KEGG -- 5.4.1.2 MetaCyc and PlantCyc -- 5.4.1.3 MetaCrop -- 5.4.2 Integrating Databases and Issues Involved -- 5.4.2.1 Compound Ambiguity -- 5.4.2.2 Reaction Redundancy -- 5.4.2.3 Formats for Exchanging Pathway Data -- 5.4.3 Adding Information to Pathway Databases -- 5.4.3.1 Manual Curation -- 5.4.3.2 Automated Methods for Literature Mining -- 5.5 Constructing Organism-Specific Pathway Databases -- 5.5.1 Enzyme Identification -- 5.5.1.1 Reference Enzyme Databases -- 5.5.1.2 Enzyme Function Prediction Using Protein Sequence Information -- 5.5.1.3 Enzyme Function Inference Using 3D Protein Structure Information -- 5.5.2 Pathway Prediction from Available Enzyme Information -- 5.5.2.1 Pathway "Painting" Using KEGG Reference Maps -- 5.5.2.2 Pathway Reconstruction with Pathway Tools -- 5.5.3 Examples of Pathway Reconstruction.
5.6 Conclusions -- References -- 6 The Role of Data Mining in the Identification of Bioactive Compounds via High-Throughput Screening -- 6.1 Introduction to the HTS Process: the Role of Data Mining -- 6.2 Relevant Data Architectures for the Analysis of HTS Data -- 6.2.1 Conditions (Parameters) for Analysis of HTS Screens -- 6.2.1.1 Purity -- 6.2.1.2 Assay Conditions -- 6.2.1.3 Previous Performance of Samples -- 6.2.2 Data Aggregation System -- 6.3 Analysis of HTS Data -- 6.3.1 Analysis of Frequent Hitters and Undesirable Compounds in Hit Lists -- 6.3.2 Analysis of Cell-Based Screening Data Leading to Mode of Mechanism Hypotheses -- 6.4 Identification of New Compounds via Compound Set Enrichment and Docking -- 6.4.1 Identification of Hit Series and SAR from Primary Screening Data by Compound Set Enrichment -- 6.4.2 Molecular Docking -- 6.5 Conclusions -- References -- 7 The Value of Interactive Visual Analytics in Drug Discovery: An Overview -- 7.1 Creating Informative Visualizations -- 7.2 Lead Discovery and Optimization -- 7.2.1 Common Visualizations -- 7.2.1.1 SAR Tables -- 7.2.1.2 Scatter Plots -- 7.2.1.3 Histograms -- 7.2.2 Advanced Visualizations -- 7.2.2.1 Profile Charts -- 7.2.2.2 Dose-Response Curves -- 7.2.2.3 Heat Maps -- 7.2.3 Interactive Analysis -- 7.3 Genomics -- 7.3.1 Common Visualizations -- 7.3.1.1 Hierarchical Clustered Heat Map -- 7.3.1.2 Scatter Plot in Log Scale -- 7.3.1.3 Histograms and Box Plots for Quality Control -- 7.3.1.4 Karyogram (Chromosomal Map) -- 7.3.2 Advanced Visualizations -- 7.3.2.1 Metabolic Pathways -- 7.3.2.2 Gene Ontology Tree Maps -- 7.3.2.3 Clustered All to All "Heat Maps" (Triangular Heat Map) -- 7.3.3 Applications -- 7.3.3.1 Understanding Diseases by Comparing Healthy with Unhealthy Tissue or Patients -- 7.3.3.2 Measure Effects of Drug Treatment on a Cellular Level -- References.
8 Using Chemoinformatics Tools from R -- 8.1 Introduction -- 8.2 System Call -- 8.2.1 Prerequisite -- 8.2.2 The Command System() -- 8.2.3 Example, Command Edition, and Outputs -- 8.3 Shared Library Call -- 8.3.1 Shared Library -- 8.3.2 Name Mangling and Calling Convention -- 8.3.3 dyn.load and dyn.unload -- 8.3.4 .C and .Fortran -- 8.3.5 Example -- 8.3.6 Compilation -- 8.4 Wrapping -- 8.4.1 Why Wrapping -- 8.4.2 Using R Internals -- 8.4.3 How to Keep an SEXP Alive -- 8.4.4 Binding to C/C++ Libraries -- 8.5 Java Archives -- 8.5.1 The Package rJava -- 8.5.2 The Package rcdk -- 8.6 Conclusions -- References -- Part Three: Applications to Polypharmacology -- 9 Content Development Strategies for the Successful Implementation of Data Mining Technologies -- 9.1 Introduction -- 9.2 Knowledge Challenges in Drug Discovery -- 9.3 Case Studies -- 9.3.1 Thomson Reuters Integrity -- 9.3.1.1 Knowledge Areas -- 9.3.1.2 Search Fields -- 9.3.1.3 Data Management Features -- 9.3.1.4 Use of Integrity in the Industry and Academia -- 9.3.2 ChemBioBank -- 9.3.3 Molecular Libraries Program -- 9.4 Knowledge-Based Data Mining Technologies -- 9.4.1 Problem Transformation Methods -- 9.4.2 Algorithm Adaptation Methods -- 9.4.3 Training a Mechanism of Action Model -- 9.5 Future Trends and Outlook -- References -- 10 Applications of Rule-Based Methods to Data Mining of Polypharmacology Data Sets -- 10.1 Introduction -- 10.2 Materials and Methods -- 10.2.1 Data Set Preparation -- 10.2.2 Preparation of the σ-1 Binders Data Set -- 10.2.3 Association Rules -- 10.2.4 Novel Hybrid Structures by Fragment Swapping -- 10.3 Results -- 10.3.1 Rules Generation and Extraction -- 10.3.1.1 Rules Describing the Polypharmacology Space -- 10.3.1.2 Optimization of σ-1 with Selectivity Over D2 -- 10.3.1.3 Optimization of σ-1 with Selectivity over D2 and 5HT2 -- 10.4 Discussion -- 10.5 Conclusions.
References.
Abstract:
Written for drug developers rather than computer scientists, this monograph adopts a systematic approach to mining scientifi c data sources, covering all key steps in rational drug discovery, from compound screening to lead compound selection and personalized medicine. Clearly divided into four sections, the first part discusses the different data sources available, both commercial and non-commercial, while the next section looks at the role and value of data mining in drug discovery. The third part compares the most common applications and strategies for polypharmacology, where data mining can substantially enhance the research effort. The final section of the book is devoted to systems biology approaches for compound testing. Throughout the book, industrial and academic drug discovery strategies are addressed, with contributors coming from both areas, enabling an informed decision on when and which data mining tools to use for one's own drug discovery project.
Local Note:
Electronic reproduction. Ann Arbor, Michigan : ProQuest Ebook Central, 2017. Available via World Wide Web. Access may be limited to ProQuest Ebook Central affiliated libraries.
Genre:
Electronic Access:
Click to View