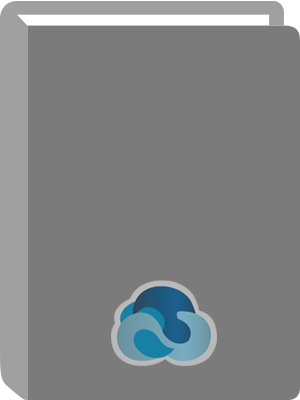
Graph-theoretic Techniques For Web Content Mining.
Title:
Graph-theoretic Techniques For Web Content Mining.
Author:
Schenker, Adam.
ISBN:
9789812569455
Personal Author:
Physical Description:
1 online resource (249 pages)
Contents:
Preface -- Acknowledgements -- Contents -- Chapter 1 Introduction to Web Mining -- 1.1 Overview of Web Mining Methodologies -- 1.2 Traditional Information Retrieval Techniques -- 1.2.1 Vector-based distance measures -- 1.2.2 Special considerations for web documents -- 1.3 Overview of Remaining Chapters -- Chapter 2 Graph Similarity Techniques -- 2.1 Graph and Subgraph Isomorphism -- 2.2 Graph Edit Distance -- 2.3 Maximum Common Subgraph / Minimum Common Supergraph Approach -- 2.4 State Space Search Approach -- 2.5 Probabilistic Approach -- 2.6 Distance Preservation Approach -- 2.7 Relaxation Approaches -- 2.8 Mean and Median of Graphs -- 2.9 Summary -- Chapter 3 Graph Models for Web Documents -- 3.1 Pre-Processing -- 3.2 Graph Representations of Web Documents -- 3.3 Complexity Analysis -- 3.4 Web Document Data Sets -- Chapter 4 Graph-Based Clustering -- 4.1 The Graph-Based k-Means Clustering Algorithm -- 4.2 Clustering Performance Measures -- 4.3 Comparison with Previously Published Results -- 4.4 Comparison of Different Graph-Theoretical Distance Measures and Graph Representations for Graph-Based Clustering -- 4.4.1 Comparison of distance measures -- 4.4.2 Comparison of graph representations -- 4.5 Comparison of Clustering Algorithms -- 4.6 Visualization of Graph Clustering -- 4.7 The Graph-Based Global k-Means Algorithm -- 4.7.1 Global k-means vs. random initialization -- 4.7.2 Optimum number of clusters -- Chapter 5 Graph-Based Classification -- 5.1 The k-Nearest Neighbors Algorithm -- 5.1.1 Traditional method -- 5.1.2 Graph-based approach -- 5.1.3 Experimental results -- 5.2 Graph-Based Multiple Classifier Ensembles -- 5.2.1 Basic algorithm -- 5.2.2 Experimental results -- Chapter 6 The Graph Hierarchy Construction Algorithm for Web Search Clustering -- 6.1 Cluster Hierarchy Construction Algorithm (CHCA) -- 6.1.1 A review of inheritance.
6.1.2 Brief overview of CHCA -- 6.1.3 CHCA in detail -- 6.1.4 CHCA: An example -- 6.1.5 Examination of CHCA as a clustering method -- 6.2 Application of CHCA to Search Results Processing -- 6.2.1 Asynchronous search -- 6.2.2 Implementation, input preparation and pre-processing -- 6.2.3 Selection of parameters for web search -- 6.3 Examples of Results -- 6.3.1 Comparison with Grouper -- 6.3.2 Comparison with Vivisimo -- 6.4 Graph Hierarchy Construction Algorithm (GHCA) -- 6.4.1 Parameters -- 6.4.2 Graph creation and pre-processing -- 6.4.3 Graph Hierarchy Construction Algorithm (GHCA) -- 6.4.4 GHCA examples -- 6.5 Comments -- Chapter 7 Conclusions and Future Work -- Appendix A Graph Examples -- College of Engineering -- Professional Engineering -- Professional Registration -- Preparation for Engineering -- Student Computer Policy -- Departments and Programs -- Chemical Engineering -- Civil and Environmental Engineering -- Computer Science and Engineering -- Electrical Engineering -- Industrial and Management Systems Engineering -- Mechanical Engineering -- Computer Service (SC) Courses -- College Computing Facilities -- Cooperative Education and Internship Programs -- Army, Air Force & Navy R.O.T.C. For Engineering Students -- Appendix B List of Stop Words -- Bibliography -- Index.
Abstract:
This book describes exciting new opportunities for utilizing robust graph representations of data with common machine learning algorithms. Graphs can model additional information which is often not present in commonly used data representations, such as vectors.
Local Note:
Electronic reproduction. Ann Arbor, Michigan : ProQuest Ebook Central, 2017. Available via World Wide Web. Access may be limited to ProQuest Ebook Central affiliated libraries.
Genre:
Electronic Access:
Click to View