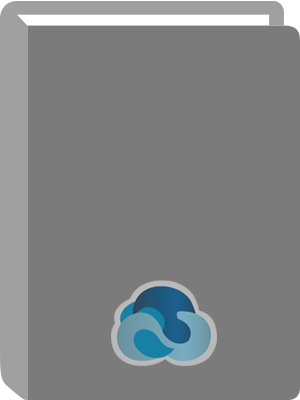
Crowdsourcing for Speech Processing : Applications to Data Collection, Transcription and Assessment.
Title:
Crowdsourcing for Speech Processing : Applications to Data Collection, Transcription and Assessment.
Author:
Eskenazi, Maxine.
ISBN:
9781118541272
Personal Author:
Edition:
1st ed.
Physical Description:
1 online resource (358 pages)
Contents:
CROWDSOURCING FOR SPEECH PROCESSING -- Contents -- List of Contributors -- Preface -- 1 An Overview -- 1.1 Origins of Crowdsourcing -- 1.2 Operational Definition of Crowdsourcing -- 1.3 Functional Definition of Crowdsourcing -- 1.4 Some Issues -- 1.5 Some Terminology -- 1.6 Acknowledgments -- References -- 2 The Basics -- 2.1 An Overview of the Literature on Crowdsourcing for Speech Processing -- 2.1.1 Evolution of the Use of Crowdsourcing for Speech -- 2.1.2 Geographic Locations of Crowdsourcing for Speech -- 2.1.3 Specific Areas of Research -- 2.2 Alternative Solutions -- 2.3 Some Ready-Made Platforms for Crowdsourcing -- 2.4 Making Task Creation Easier -- 2.5 Getting Down to Brass Tacks -- 2.5.1 Hearing and Being Heard over the Web -- 2.5.2 Prequalification -- 2.5.3 Native Language of the Workers -- 2.5.4 Payment -- 2.5.5 Choice of Platform in the Literature -- 2.5.6 The Complexity of the Task -- 2.6 Quality Control -- 2.6.1 Was That Worker a Bot? -- 2.6.2 Quality Control in the Literature -- 2.7 Judging the Quality of the Literature -- 2.8 Some Quick Tips -- 2.9 Acknowledgments -- References -- Further reading -- 3 Collecting Speech from Crowds -- 3.1 A Short History of Speech Collection -- 3.1.1 Speech Corpora -- 3.1.2 Spoken Language Systems -- 3.1.3 User-Configured Recording Environments -- 3.2 Technology for Web-Based Audio Collection -- 3.2.1 Silverlight -- 3.2.2 Java -- 3.2.3 Flash -- 3.2.4 HTML and JavaScript -- 3.3 Example: WAMI Recorder -- 3.3.1 The JavaScript API -- 3.3.2 Audio Formats -- 3.4 Example: The WAMI Server -- 3.4.1 PHP Script -- 3.4.2 Google App Engine -- 3.4.3 Server Configuration Details -- 3.5 Example: Speech Collection on Amazon Mechanical Turk -- 3.5.1 Server Setup -- 3.5.2 Deploying to Amazon Mechanical Turk -- 3.5.3 The Command-Line Interface -- 3.6 Using the Platform Purely for Payment.
3.7 Advanced Methods of Crowdsourced Audio Collection -- 3.7.1 Collecting Dialog Interactions -- 3.7.2 Human Computation -- 3.8 Summary -- 3.9 Acknowledgments -- References -- 4 Crowdsourcing for Speech Transcription -- 4.1 Introduction -- 4.1.1 Terminology -- 4.2 Transcribing Speech -- 4.2.1 The Need for Speech Transcription -- 4.2.2 Quantifying Speech Transcription -- 4.2.3 Brief History -- 4.2.4 Is Crowdsourcing Well Suited to My Needs? -- 4.3 Preparing the Data -- 4.3.1 Preparing the Audio Clips -- 4.3.2 Preprocessing the Data with a Speech Recognizer -- 4.3.3 Creating a Gold-Standard Dataset -- 4.4 Setting Up the Task -- 4.4.1 Creating Your Task with the Platform Template Editor -- 4.4.2 Creating Your Task on Your Own Server -- 4.4.3 Instruction Design -- 4.4.4 Know the Workers -- 4.4.5 Game Interface -- 4.5 Submitting the Open Call -- 4.5.1 Payment -- 4.5.2 Number of Distinct Judgments -- 4.6 Quality Control -- 4.6.1 Normalization -- 4.6.2 Unsupervised Filters -- 4.6.3 Supervised Filters -- 4.6.4 Aggregation Techniques -- 4.6.5 Quality Control Using Multiple Passes -- 4.7 Conclusion -- 4.8 Acknowledgments -- References -- 5 How to Control and Utilize Crowd-Collected Speech -- 5.1 Read Speech -- 5.1.1 Collection Procedure -- 5.1.2 Corpus Overview -- 5.2 Multimodal Dialog Interactions -- 5.2.1 System Design -- 5.2.2 Scenario Creation -- 5.2.3 Data Collection -- 5.2.4 Data Transcription -- 5.2.5 Data Analysis -- 5.3 Games for Speech Collection -- 5.4 Quizlet -- 5.5 Voice Race -- 5.5.1 Self-Transcribed Data -- 5.5.2 Simplified Crowdsourced Transcription -- 5.5.3 Data Analysis -- 5.5.4 Human Transcription -- 5.5.5 Automatic Transcription -- 5.5.6 Self-Supervised Acoustic Model Adaptation -- 5.6 Voice Scatter -- 5.6.1 Corpus Overview -- 5.6.2 Crowdsourced Transcription -- 5.6.3 Filtering for Accurate Hypotheses.
5.6.4 Self-Supervised Acoustic Model Adaptation -- 5.7 Summary -- 5.8 Acknowledgments -- References -- 6 Crowdsourcing in Speech Perception -- 6.1 Introduction -- 6.2 Previous Use of Crowdsourcing in Speech and Hearing -- 6.3 Challenges -- 6.3.1 Control of the Environment -- 6.3.2 Participants -- 6.3.3 Stimuli -- 6.4 Tasks -- 6.4.1 Speech Intelligibility, Quality and Naturalness -- 6.4.2 Accent Evaluation -- 6.4.3 Perceptual Salience and Listener Acuity -- 6.4.4 Phonological Systems -- 6.5 BigListen: A Case Study in the Use of Crowdsourcing to Identify Words in Noise -- 6.5.1 The Problem -- 6.5.2 Speech and Noise Tokens -- 6.5.3 The Client-Side Experience -- 6.5.4 Technical Architecture -- 6.5.5 Respondents -- 6.5.6 Analysis of Responses -- 6.5.7 Lessons from the BigListen Crowdsourcing Test -- 6.6 Issues for Further Exploration -- 6.7 Conclusions -- References -- 7 Crowdsourced Assessment of Speech Synthesis -- 7.1 Introduction -- 7.2 Human Assessment of TTS -- 7.3 Crowdsourcing for TTS: What Worked and What Did Not -- 7.3.1 Related Work: Crowdsourced Listening Tests -- 7.3.2 Problem and Solutions: Audio on the Web -- 7.3.3 Problem and Solution: Test of Significance -- 7.3.4 What Assessment Types Worked -- 7.3.5 What Did Not Work -- 7.3.6 Problem and Solutions: Recruiting Native Speakers of Various Languages -- 7.3.7 Conclusion -- 7.4 Related Work: Detecting and Preventing Spamming -- 7.5 Our Experiences: Detecting and Preventing Spamming -- 7.5.1 Optional Playback Interface -- 7.5.2 Investigating the Metrics Further: Mandatory Playback Interface -- 7.5.3 The Prosecutor's Fallacy -- 7.6 Conclusions and Discussion -- References -- 8 Crowdsourcing for Spoken Dialog System Evaluation -- 8.1 Introduction -- 8.2 Prior Work on Crowdsourcing: Dialog and Speech Assessment -- 8.2.1 Prior Work on Crowdsourcing for Dialog Systems.
8.2.2 Prior Work on Crowdsourcing for Speech Assessment -- 8.3 Prior Work in SDS Evaluation -- 8.3.1 Subjective User Judgments -- 8.3.2 Interaction Metrics -- 8.3.3 PARADISE Framework -- 8.3.4 Alternative Approach to Crowdsourcing for SDS Evaluation -- 8.4 Experimental Corpus and Automatic Dialog Classification -- 8.5 Collecting User Judgments on Spoken Dialogs with Crowdsourcing -- 8.5.1 Tasks for Dialog Evaluation -- 8.5.2 Tasks for Interannotator Agreement -- 8.5.3 Approval of Ratings -- 8.6 Collected Data and Analysis -- 8.6.1 Approval Rates and Comments from Workers -- 8.6.2 Consistency between Automatic Dialog Classification and Manual Ratings -- 8.6.3 Interannotator Agreement among Workers -- 8.6.4 Interannotator Agreement on the Let's Go! System -- 8.6.5 Consistency between Expert and Nonexpert Annotations -- 8.7 Conclusions and Future Work -- 8.8 Acknowledgments -- References -- 9 Interfaces for Crowdsourcing Platforms -- 9.1 Introduction -- 9.2 Technology -- 9.2.1 TinyTask Web Page -- 9.2.2 World Wide Web -- 9.2.3 Hypertext Transfer Protocol -- 9.2.4 Hypertext Markup Language -- 9.2.5 Cascading Style Sheets -- 9.2.6 JavaScript -- 9.2.7 JavaScript Object Notation -- 9.2.8 Extensible Markup Language -- 9.2.9 Asynchronous JavaScript and XML -- 9.2.10 Flash -- 9.2.11 SOAP and REST -- 9.2.12 Section Summary -- 9.3 Crowdsourcing Platforms -- 9.3.1 Crowdsourcing Platform Workflow -- 9.3.2 Amazon Mechanical Turk -- 9.3.3 CrowdFlower -- 9.3.4 Clickworker -- 9.3.5 WikiSpeech -- 9.4 Interfaces to Crowdsourcing Platforms -- 9.4.1 Implementing Tasks Using a GUI on the CrowdFlower Platform -- 9.4.2 Implementing Tasks Using the Command-Line Interface in MTurk -- 9.4.3 Implementing a Task Using a RESTful Web Service in Clickworker -- 9.4.4 Defining Tasks via Configuration Files in WikiSpeech -- 9.5 Summary -- References.
10 Crowdsourcing for Industrial Spoken Dialog Systems -- 10.1 Introduction -- 10.1.1 Industry's Willful Ignorance -- 10.1.2 Crowdsourcing in Industrial Speech Applications -- 10.1.3 Public versus Private Crowd -- 10.2 Architecture -- 10.3 Transcription -- 10.4 Semantic Annotation -- 10.5 Subjective Evaluation of Spoken Dialog Systems -- 10.6 Conclusion -- References -- 11 Economic and Ethical Background of Crowdsourcing for Speech -- 11.1 Introduction -- 11.2 The Crowdsourcing Fauna -- 11.2.1 The Crowdsourcing Services Landscape -- 11.2.2 Who Are the Workers? -- 11.2.3 Ethics and Economics in Crowdsourcing: How to Proceed? -- 11.3 Economic and Ethical Issues -- 11.3.1 What Are the Problems for the Workers? -- 11.3.2 Crowdsourcing and Labor Laws -- 11.3.3 Which Economic Model Is Sustainable for Crowdsourcing? -- 11.4 Under-Resourced Languages: A Case Study -- 11.4.1 Under-Resourced Languages Definition and Issues -- 11.4.2 Collecting Annotated Speech for African Languages Using Crowdsourcing -- 11.4.3 Experiment Description -- 11.4.4 Results -- 11.4.5 Discussion and Lessons Learned -- 11.5 Toward Ethically Produced Language Resources -- 11.5.1 Defining a Fair Compensation for Work Done -- 11.5.2 Impact of Crowdsourcing on the Ecology of Linguistic Resources -- 11.5.3 Defining an Ethical Framework: Some Solutions -- 11.6 Conclusion -- Disclaimer -- References -- Index.
Abstract:
Provides an insightful and practical introduction to crowdsourcing as a means of rapidly processing speech data Intended for those who want to get started in the domain and learn how to set up a task, what interfaces are available, how to assess the work, etc. as well as for those who already have used crowdsourcing and want to create better tasks and obtain better assessments of the work of the crowd. It will include screenshots to show examples of good and poor interfaces; examples of case studies in speech processing tasks, going through the task creation process, reviewing options in the interface, in the choice of medium (MTurk or other) and explaining choices, etc. Provides an insightful and practical introduction to crowdsourcing as a means of rapidly processing speech data. Addresses important aspects of this new technique that should be mastered before attempting a crowdsourcing application. Offers speech researchers the hope that they can spend much less time dealing with the data gathering/annotation bottleneck, leaving them to focus on the scientific issues. Readers will directly benefit from the book's successful examples of how crowd- sourcing was implemented for speech processing, discussions of interface and processing choices that worked and choices that didn't, and guidelines on how to play and record speech over the internet, how to design tasks, and how to assess workers. Essential reading for researchers and practitioners in speech research groups involved in speech processing.
Local Note:
Electronic reproduction. Ann Arbor, Michigan : ProQuest Ebook Central, 2017. Available via World Wide Web. Access may be limited to ProQuest Ebook Central affiliated libraries.
Genre:
Electronic Access:
Click to View