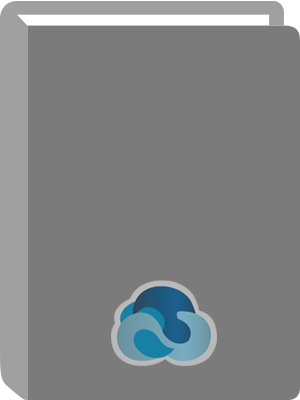
Parametric Statistical Theory.
Title:
Parametric Statistical Theory.
Author:
Pfanzagl, Johann.
ISBN:
9783110889765
Personal Author:
Physical Description:
1 online resource (388 pages)
Series:
De Gruyter Textbook
Contents:
Preface -- Introduction -- Chapter 1 Sufficiency and completeness -- 1.1 Introduction -- 1.2 Sufficiency and factorization of densities -- 1.3 Sufficiency and exhaustivity -- 1.4 Minimal sufficiency -- 1.5 Completeness -- 1.6 Exponential families -- 1.7 Auxiliary results on families with monotone likelihood ratios -- 1.8 Ancillary statistics -- 1.9 Equivariance and invariance -- 1.10 Appendix: Conditional expectations, conditional distributions -- Chapter 2 The evaluation of estimators -- 2.1 Introduction -- 2.2 Unbiasedness of estimators -- 2.3 The concentration of real valued estimators -- 2.4 Concentration of multivariate estimators -- 2.5 Evaluating estimators by loss functions -- 2.6 The relative efficiency of estimators -- 2.7 Examples on the evaluation of estimators -- Chapter 3 Mean unbiased estimators and convex loss functions -- 3.1 Introduction -- 3.2 The Rao-Blackwell-Lehmann-Scheffé-Theorem -- 3.3 Examples of mean unbiased estimators with minimal convex risk -- 3.4 Mean unbiased estimation of probabilities -- 3.5 A result on bounded mean unbiased estimators -- Chapter 4 Testing hypotheses -- 4.1 Basic concepts -- 4.2 Critical functions, critical regions -- 4.3 The Neyman-Pearson Lemma -- 4.4 Optimal tests for composite hypotheses -- 4.5 Optimal tests for families with monotone likelihood ratios -- 4.6 Tests of Neyman structure -- 4.7 Most powerful similar tests for a real parameter in the presence of a nuisance parameter -- Chapter 5 Confidence procedures -- 5.1 Basic concepts -- 5.2 The evaluation of confidence procedures -- 5.3 The construction of one-sided confidence bounds and median unbiased estimators -- 5.4 Optimal one-sided confidence bounds and median unbiased estimators -- 5.5 Optimal one-sided confidence bounds and median unbiased estimators in the presence of a nuisance parameter.
5.6 Examples of maximally concentrated confidence bounds -- Chapter 6 Consistent estimators -- 6.1 Introduction -- 6.2 A general consistency theorem -- 6.3 Consistency of M-estimators -- 6.4 Consistent solutions of estimating equations -- 6.5 Consistency of maximum likelihood estimators -- 6.6 Examples of ML estimators -- 6.7 Appendix: Uniform integrability, stochastic convergence and measurable selection -- Chapter 7 Asymptotic distributions of estimator sequences -- 7.1 Limit distributions -- 7.2 How to deal with limit distributions -- 7.3 Asymptotic confidence bounds -- 7.4 Solutions to estimating equations -- 7.5 The limit distribution of ML estimator sequences -- 7.6 Stochastic approximations to estimator sequences -- 7.7 Appendix: Weak convergence -- Chapter 8 Asymptotic bounds for the concentration of estimators and confidence bounds -- 8.1 Introduction -- 8.2 Regular sequences of confidence bounds and median unbiased estimators -- 8.3 Sequences of confidence bounds and median unbiased estimators with limit distributions -- 8.4 The convolution theorem -- 8.5 Maximally concentrated limit distributions -- 8.6 Superefficiency -- Chapter 9 Miscellaneous results on asymptotic distributions -- 9.1 Examples of ML estimators -- 9.2 Tolerance bounds -- 9.3 Probability measures with location- and scale parameters -- 9.4 Miscellaneous results on estimators -- Chapter 10 Asymptotic test theory -- 10.1 Introduction -- 10.2 Tests for a real valued functional -- 10.3 The asymptotic envelope power function for tests for a real valued functional -- References -- Author Index -- Subject Index -- Notation Index.
Abstract:
Parametric Statistical Theory.
Local Note:
Electronic reproduction. Ann Arbor, Michigan : ProQuest Ebook Central, 2017. Available via World Wide Web. Access may be limited to ProQuest Ebook Central affiliated libraries.
Genre:
Electronic Access:
Click to View