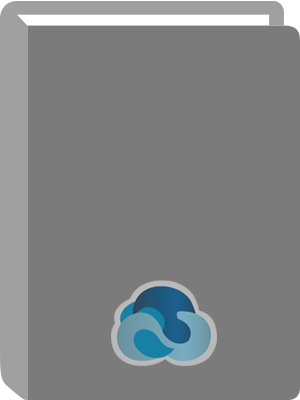
Data Mining Applications Using Ontologies in Biomedicine.
Title:
Data Mining Applications Using Ontologies in Biomedicine.
Author:
Popescu, Mihail.
ISBN:
9781596933712
Personal Author:
Edition:
1st ed.
Physical Description:
1 online resource (278 pages)
Contents:
Data Mining in Biomedicine Using Ontologies -- Contents -- Foreword -- Preface -- Chapter 1 Introduction to Ontologies -- 1.1 Introduction -- 1.2 History of Ontologies in Biomedicine -- 1.2.1 The Philosophical Connection -- 1.2.2 Recent Defi nition in Computer Science -- 1.2.3 Origins of Bio-Ontologies -- 1.2.4 Clinical and Medical Terminologies -- 1.2.5 Recent Advances in Computer Science -- 1.3 Form and Function of Ontologies -- 1.3.1 Basic Components of Ontologies -- 1.3.2 Components for Humans, Components for Computers -- 1.3.3 Ontology Engineering -- 1.4 Encoding Ontologies -- 1.4.1 The OBO Format and the OBO Consortium -- 1.4.2 OBO-Edit-The Open Biomedical Ontologies Editor -- 1.4.3 OWL and RDF/XML -- 1.4.4 Protégé-An OWL Ontology Editor -- 1.5 Spotlight on GO and UMLS -- 1.5.1 The Gene Ontology -- 1.5.2 The Unifi ed Medical Language System -- 1.6 Types and Examples of Ontologies -- 1.6.1 Upper Ontologies -- 1.6.2 Domain Ontologies -- 1.6.3 Formal Ontologies -- 1.6.4 Informal Ontologies -- 1.6.5 Reference Ontologies -- 1.6.6 Application Ontologies -- 1.6.7 Bio-Ontologies -- 1.7 Conclusion -- References -- Chapter 2 Ontological Similarity Measures -- 2.1 Introduction -- 2.1.1 History -- 2.1.2 Tversky's Parameterized Ratio Model of Similarity -- 2.1.3 Aggregation in Similarity Assessment -- 2.2 Traditional Approaches to Ontological Similarity -- 2.2.1 Path-Based Measures -- 2.2.2 Information Content Measures -- 2.2.3 A Relationship Between Path-Based and Information-Content Measures -- 2.3 New Approaches to Ontological Similarity -- 2.3.1 Entity Class Similarity in Ontologies -- 2.3.2 Cross-Ontological Similarity Measures -- 2.3.3 Exploiting Common Disjunctive Ancestors -- 2.4 Conclusion -- References -- Chapter 3 Clustering with Ontologies -- 3.1 Introduction -- 3.2 Relational Fuzzy C-Means (NERFCM) -- 3.3 Correlation Cluster Validity (CCV).
3.4 Ontological SOM (OSOM) -- 3.5 Examples of NERFCM, CCV, and OSOM Applications -- 3.5.1 Test Dataset -- 3.5.2 Clustering of the GPD194 Dataset Using NERFCM -- 3.5.3 Determining the Number of Clusters of GPD194 Dataset Using CCV -- 3.5.4 GPD194 Analysis Using OSOM -- 3.6 Conclusion -- References -- Chapter 4 Analyzing and Classifying Protein Family Data Using OWL Reasoning -- 4.1 Introduction -- 4.1.1 Analyzing Sequence Data -- 4.1.2 The Protein Phosphatase Family -- 4.2 Methods -- 4.2.1 The Phosphatase Classification Pipeline -- 4.2.2 The Datasets -- 4.2.3 The Phosphatase Ontology -- 4.3 Results -- 4.3.1 Protein Phosphatases in Humans -- 4.3.2 Results from the Analysis of A. Fumigatus -- 4.3.3 Ontology System Versus A. Fumigatus Automated Annotation Pipeline -- 4.4 Ontology Classification in the Comparative Analysis of Three Protozoan Parasites-A Case Study -- 4.4.1 TriTryps Diseases -- 4.4.2 TriTryps Protein Phosphatases -- 4.4.3 Methods for the Protozoan Parasites -- 4.4.4 Sequence Analysis Results from the TriTryps Phosphatome Study -- 4.4.5 Evaluation of the Ontology Classification Method -- 4.5 Conclusion -- References -- Chapter 5 GO-Based Gene Function and Network Characterization -- 5.1 Introduction -- 5.2 GO-Based Functional Similarity -- 5.2.1 GO Index-Based Functional Similarity -- 5.2.2 GO Semantic Similarity -- 5.3 Functional Relationship and High-Throughput Data -- 5.3.1 Gene-Gene Relationship Revealed in Microarray Data -- 5.3.2 The Relation Between Functional and Sequence Similarity -- 5.4 Theoretical Basis for Building Relationship Among Genes Through Data -- 5.4.1 Building the Relationship Among Genes Using One Dataset -- 5.4.2 Meta-Analysis of Microarray Data -- 5.4.3 Function Learning from Data -- 5.4.4 Functional-Linkage Network -- 5.5 Function-Prediction Algorithms -- 5.5.1 Local Prediction.
5.5.2 Global Prediction Using a Boltzmann Machine -- 5.6 Gene Function-Prediction Experiments -- 5.6.1 Data Processing -- 5.6.2 Sequence-Based Prediction -- 5.6.3 Meta-Analysis of Yeast Microarray Data -- 5.6.4 Case Study: Sin1 and PCBP2 Interactions -- 5.7 Transcription Network Feature Analysis -- 5.7.1 Time Delay in Transcriptional Regulation -- 5.7.2 Kinetic Model for Time Series Microarray -- 5.7.3 Regulatory Network Reconstruction -- 5.7.4 GO-Enrichment Analysis -- 5.8 Software Implementation -- 5.8.1 GENEFAS -- 5.8.2 Tools for Meta-Analysis -- 5.9 Conclusion -- Acknowledgements -- References -- Chapter 6 Mapping Genes to Biological Pathways Using Ontological Fuzzy Rule Systems -- 6.1 Rule-Based Representation in Biomedical Applications -- 6.2 Ontological Similarity as a Fuzzy Membership -- 6.3 Ontological Fuzzy Rule System (OFRS) -- 6.4 Application of OFRSs: Mapping Genes to Biological Pathways -- 6.4.1 Mapping Gene to Pathways Using a Disjunctive OFRS -- 6.4.2 Mapping Genes to Pathways Using an OFRS in an Evolutionary Framework -- 6.5 Conclusion -- Acknowledgments -- References -- Chapter 7 Extracting Biological Knowledge by Association Rule Mining -- 7.1 Association Rule Mining and Fuzzy Association Rule Mining Overview -- 7.1.1 Association Rules: Formal Defi nition -- 7.1.2 Association Rule Mining Algorithms -- 7.1.3 Apriori Algorithm -- 7.1.4 Fuzzy Association Rules -- 7.2 Using GO in Association Rule Mining -- 7.2.1 Unveiling Biological Associations by Extracting Rules Involving GO Terms -- 7.2.2 Giving Biological Signifi cance to Rule Sets by Using GO -- 7.2.3 Other Joint Applications of Association Rules and GO -- 7.3 Applications for Extracting Knowledge from Microarray Data -- 7.3.1 Association Rules That Relate Gene Expression Patterns with Other Features.
7.3.2 Association Rules to Obtain Relations Between Genes and TheirExpression Values -- Acknowledgements -- References -- Chapter 8 Text Summarization Using Ontologies -- 8.1 Introduction -- 8.2 Representing Background Knowledge-Ontology -- 8.2.1 An Algebraic Approach to Ontologies -- 8.2.2 Modeling Ontologies -- 8.2.3 Deriving Similarity -- 8.3 Referencing the Background Knowledge-Providing Descriptions -- 8.3.1 Instantiated Ontology -- 8.4 Data Summarization Through Background Knowledge -- 8.4.1 Connectivity Clustering -- 8.4.2 Similarity Clustering -- 8.5 Conclusion -- References -- Chapter 9 Reasoning over Anatomical Ontologies -- 9.1 Why Reasoning Matters -- 9.2 Data, Reasoning, and a New Frontier -- 9.2.1 A Taxonomy of Data and Reasoning -- 9.2.2 Contemporary Reasoners -- 9.2.3 Anatomy as a New Frontier for Biological Reasoners -- 9.3 Biological Ontologies Today -- 9.3.1 Current Practices -- 9.3.2 Structural Issues That Limit Reasoning -- 9.3.3 A Biological Example: The Maize Tassel -- 9.3.4 Representational Issues -- 9.4 Facilitating Reasoning About Anatomy -- 9.4.1 Link Different Kinds of Knowledge -- 9.4.2 Layer on Top of the Ontology -- 9.4.3 Change the Representation -- 9.5 Some Visions for the Future -- Acknowledgments -- References -- Chapter 10 Ontology Applications in Text Mining -- 10.1 Introduction -- 10.1.1 What Is Text Mining? -- 10.1.2 Ontologies -- 10.2 The Importance of Ontology to Text Mining -- 10.3 Semantic Document Clustering and Summarization: Ontology Applications in Text Mining -- 10.3.1 Introduction to Document Clustering -- 10.3.2 The Graphical Representation Model -- 10.3.3 Graph Clustering for Graphical Representations -- 10.3.4 Text Summarization -- 10.3.5 Document Clustering and Summarization with Graphical Representation -- 10.4 Swanson's Undiscovered Public Knowledge (UDPK) -- 10.4.1 How Does UDPK Work?.
10.4.2 A Semantic Version of Swanson's UDPK Model -- 10.4.3 The Bio-SbKDS Algorithm -- 10.5 Conclusion -- References -- About the Editors -- List of Contributors -- Index.
Abstract:
Presently, a growing number of ontologies are being built and used for annotating data in biomedical research. Thanks to the tremendous amount of data being generated, ontologies are now being used in numerous ways, including connecting different databases, refining search capabilities, interpreting experimental/clinical data, and inferring knowledge. This cutting-edge resource introduces you to latest developments in bio-ontologies. The book provides you with the theoretical foundations and examples of ontologies, as well as applications of ontologies in biomedicine, from molecular levels to clinical levels. You also find details on technological infrastructure for bio-ontologies. This comprehensive, one-stop volume presents a wide range of practical bio-ontology information, offering you detailed guidance in the clustering of biological data, protein classification, gene and pathway prediction, and text mining. More than 160 illustrations support key topics throughout the book.
Local Note:
Electronic reproduction. Ann Arbor, Michigan : ProQuest Ebook Central, 2017. Available via World Wide Web. Access may be limited to ProQuest Ebook Central affiliated libraries.
Genre:
Added Author:
Electronic Access:
Click to View