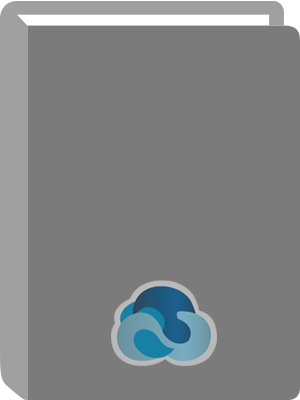
Integrative Cluster Analysis in Bioinformatics.
Title:
Integrative Cluster Analysis in Bioinformatics.
Author:
Abu-Jamous, Basel.
ISBN:
9781118906569
Personal Author:
Edition:
1st ed.
Physical Description:
1 online resource (454 pages)
Contents:
Title Page -- Copyright Page -- Brief Contents -- Contents -- Preface -- List of Symbols -- About the Authors -- Part One Introduction -- Chapter 1 Introduction to Bioinformatics -- 1.1 Introduction -- 1.2 The ``Omics ́́Era -- 1.3 The Scope of Bioinformatics -- 1.3.1 Areas of Molecular Biology Subject to Bioinformatics Analysis -- 1.3.2 Data Storage, Retrieval and Organisation -- 1.3.3 Data Analysis -- 1.3.4 Statistical Analysis -- 1.3.5 Presentation -- 1.4 What Do Information Engineers and Biologists Need to Know? -- 1.5 Discussion and Summary -- References -- Chapter 2 Computational Methods in Bioinformatics -- 2.1 Introduction -- 2.2 Machine Learning and Data Mining -- 2.2.1 Supervised Learning -- 2.2.2 Unsupervised Learning -- 2.3 Optimisation -- 2.4 Image Processing: Bioimage Informatics -- 2.5 Network Analysis -- 2.6 Statistical Analysis -- 2.7 Software Tools and Technologies -- 2.8 Discussion and Summary -- References -- Part Two Introduction to Molecular Biology -- Chapter 3 The Living Cell -- 3.1 Introduction -- 3.2 Prokaryotes and Eukaryotes -- 3.3 Multicellularity -- 3.3.1 Unicellular and Multicellular Organisms -- 3.3.2 Stem Cells and Cell Differentiation -- 3.4 Cell Components -- 3.4.1 Plasma Membrane and Transport Proteins -- 3.4.2 Cytoplasm -- 3.4.3 Extracellular Matrix -- 3.4.4 Centrosome and Microtubules -- 3.4.5 Actin Filaments and the Cytoskeleton -- 3.4.6 Nucleus -- 3.4.7 Vesicles -- 3.4.8 Ribosomes -- 3.4.9 Endoplasmic Reticulum -- 3.4.10 Golgi Apparatus -- 3.4.11 Mitochondrion and the Energy of the Cell -- 3.4.12 Lysosome -- 3.4.13 Peroxisome -- 3.5 Discussion and Summary -- References -- Chapter 4 Central Dogma of Molecular Biology -- 4.1 Introduction -- 4.2 Central Dogma of Molecular Biology Overview -- 4.3 Proteins -- 4.4 DNA -- 4.5 RNA -- 4.6 Genes -- 4.7 Transcription and Post-transcriptional Processes.
4.7.1 Post-transcriptional Processes -- 4.7.2 Gene-specific TFs -- 4.7.3 Post-transcriptional Regulation -- 4.8 Translation and Post-translational Processes -- 4.8.1 The Genetic Code -- 4.8.2 tRNAand Ribosomes -- 4.8.3 The Steps of Translation -- 4.8.4 Polyribosomes (Polysomes) -- 4.8.5 Post-translational Processes -- 4.9 Discussion and Summary -- References -- Part Three Data Acquisition and Pre-processing -- Chapter 5 High-throughput Technologies -- 5.1 Introduction -- 5.2 Microarrays -- 5.2.1 DNAMicroarrays -- 5.2.2 Protein Microarrays -- 5.2.3 Carbohydrate Microarrays (Glycoarrays) -- 5.2.4 Other Types of Microarrays -- 5.3 Next-generation Sequencing (NGS) -- 5.3.1 DNA Sequencing -- 5.3.2 RNA Sequencing (Transcripome Analysis) -- 5.3.3 Metagenomics -- 5.3.4 Other Applications of Sequencing -- 5.4 ChIPon Microarrays and Sequencing -- 5.5 Discussion and Summary -- References -- Chapter 6 Databases, Standards and Annotation -- 6.1 Introduction -- 6.2 NCBI Databases -- 6.2.1 Literature Databases (PubMed, PMC, the Bookshelf and MeSH) -- 6.2.2 GenBank (Nucleotide Database) -- 6.2.3 Reference Sequences (RefSeq) Database -- 6.2.4 Gene Database -- 6.2.5 Protein Database -- 6.2.6 Gene Expression Omnibus -- 6.2.7 Taxonomy and HomoloGene Databases -- 6.2.8 Sequence Read Archive -- 6.2.9 Genomic and Epigenomic Variations -- 6.2.10 Other NCBI Databases -- 6.3 The EBI Databases -- 6.4 Species-specific Databases -- 6.4.1 Animals -- 6.4.2 Plants -- 6.4.3 Fungi -- 6.4.4 Archaea and Bacteria -- 6.4.5 Viruses -- 6.5 Discussion and Summary -- References -- Chapter 7 Normalisation -- 7.1 Introduction -- 7.2 Issues Tackled by Normalisation -- 7.2.1 Within-slide and Between-slides Normalisation -- 7.2.2 Normalisation Based on Non-differentially Expressed Genes -- 7.2.3 Background Correction -- 7.2.4 Logarithmic Transformation.
7.2.5 Intensity-dependent Bias - (MA) Plots -- 7.2.6 Replicates and Summarisation -- 7.3 Normalisation Methods -- 7.3.1 Microarray Suite 5 (MAS5.0) -- 7.3.2 Robust Multi-array Average (RMA) -- 7.3.3 Quantile Normalisation -- 7.3.4 Locally Weighted Scatter-plot Smoothing (Lowess) Normalisation -- 7.3.5 Scaling Methods -- 7.3.6 Model-based Expression Index (MBEI) -- 7.3.7 Other Normalisation Methods -- 7.4 Discussion and Summary -- References -- Chapter 8 Feature Selection -- 8.1 Introduction -- 8.2 FS and FG - Problem Definition -- 8.3 Consecutive Ranking -- 8.3.1 Forward Search (Most Informative First Admitted) -- 8.3.2 Backward Elimination (Least Useful First Eliminated) -- 8.4 Individual Ranking -- 8.4.1 Information Content -- 8.4.2 SNR Criteria -- 8.5 Principal Component Analysis -- 8.6 Genetic Algorithms and Genetic Programming -- 8.7 Discussion and Summary -- References -- Chapter 9 Differential Expression -- 9.1 Introduction -- 9.2 Fold Change -- 9.3 Statistical Hypothesis Testing - Overview -- 9.3.1 p-Values and Volcano Plots -- 9.3.2 The Multiple-hypothesis Testing Problem -- 9.4 Statistical Hypothesis Testing - Methods -- 9.4.1 t-Statistic, Modified t-Statistics and the Analysis of Variance (ANOVA) -- 9.4.2 B-Statistic -- 9.4.3 Fisherś Exact Test -- 9.4.4 Likelihood Ratio Test -- 9.4.5 Methods for Over-dispersed Poisson Distribution -- 9.5 Discussion and Summary -- References -- Part Four Clustering Methods -- Chapter 10 Clustering Forms -- 10.1 Introduction -- 10.2 Proximity Measures -- 10.2.1 Distance Metrics for Discrete Feature Objects -- 10.2.1.1 Hamming Distance -- 10.2.1.2 Matching Coefficient -- 10.2.2 Distance Metrics for Continuous Feature Objects -- 10.3 Clustering Families -- 10.3.1 Partitional Clustering -- 10.3.2 Hierarchical Clustering -- 10.3.3 Fuzzy Clustering -- 10.3.4 Neural Network-based Clustering.
10.3.5 Mixture Model Clustering -- 10.3.6 Graph-based Clustering -- 10.3.7 Consensus Clustering -- 10.3.8 Biclustering -- 10.4 Clusters and Partitions -- 10.5 Discussion and Summary -- References -- Chapter 11 Partitional Clustering -- 11.1 Introduction -- 11.2 k-Means and its Applications -- 11.2.1 Principles -- 11.2.2 Variations -- 11.2.3 Applications -- 11.3 k-Medoids and its Applications -- 11.3.1 Principles -- 11.3.2 Variations -- 11.3.3 Applications -- 11.4 Discussion and Summary -- References -- Chapter 12 Hierarchical Clustering -- 12.1 Introduction -- 12.2 Principles -- 12.2.1 Agglomerative Methods -- 12.2.2 Divisive Methods -- 12.3 Discussion and Summary -- References -- Chapter 13 Fuzzy Clustering -- 13.1 Introduction -- 13.2 Principles -- 13.2.1 Fuzzy c-Means -- 13.2.2 Probabilistic c-Means -- 13.2.3 Hybrid c-Means -- 13.2.4 Gustafson-Kessel Algorithm -- 13.2.5 Gath-Geva Algorithm -- 13.2.6 Fuzzy c-Shell -- 13.2.7 FANNY -- 13.2.8 Other Fuzzy Clustering Algorithms -- 13.3 Discussion -- References -- Chapter 14 Neural Network-based Clustering -- 14.1 Introduction -- 14.2 Algorithms -- 14.2.1 SOM -- 14.2.2 GLVQ -- 14.2.3 Neural-gas -- 14.2.4 ART -- 14.2.5 OPTOC -- 14.2.6 SOON -- 14.3 Discussion -- References -- Chapter 15 Mixture Model Clustering -- 15.1 Introduction -- 15.2 Finite Mixture Models -- 15.2.1 Various Mixture Models -- 15.2.2 Non-Bayesian Methods -- 15.2.3 Bayesian Methods -- 15.3 Infinite Mixture Models -- 15.3.1 DPM Model -- 15.3.2 CRP Mixture Model -- 15.3.3 SBP Mixture Model -- 15.4 Discussion -- References -- Chapter 16 Graph Clustering -- 16.1 Introduction -- 16.2 Basic Definitions -- 16.2.1 Graph and Adjacency Matrix -- 16.2.2 Measures and Metrics -- 16.2.3 Similarity Matrices -- 16.3 Graph Clustering -- 16.3.1 Graph Cut Clustering -- 16.3.2 Spectral Clustering -- 16.3.3 AP Clustering -- 16.3.4 Modularity-based Clustering.
16.3.5 Multilevel Graph Partitioning and Hypergraph Partitioning -- 16.3.6 Markov Cluster Algorithm -- 16.4 Resources -- 16.5 Discussion -- References -- Chapter 17 Consensus Clustering -- 17.1 Introduction -- 17.2 Overview -- 17.3 Consensus Functions -- 17.3.1 P-P Comparison -- 17.3.2 C-C Comparison -- 17.3.3 MIC Voting -- 17.3.4 M-M Co-occurrence -- 17.4 Discussion -- References -- Chapter 18 Biclustering -- 18.1 Introduction -- 18.2 Overview -- 18.2.1 Statement of the Biclustering Problem -- 18.2.2 Types of Biclusters -- 18.2.3 Classification of Biclustering -- 18.3 Biclustering Methods -- 18.3.1 Variance-minimisation Biclustering Methods -- 18.3.2 Correlation-maximisation Biclustering Methods -- 18.3.3 Two-way Clustering Methods -- 18.3.4 Probabilistic and Generative Methods -- 18.4 Discussion -- References -- Chapter 19 Clustering Methods Discussion -- 19.1 Introduction -- 19.2 Hierarchical Clustering -- 19.2.1 Yeast Cell Cycle Data -- 19.2.2 Breast Cancer -- 19.2.3 Diffuse Large B-Cell Lymphoma -- 19.3 Fuzzy Clustering -- 19.3.1 DNA Motifs Clustering -- 19.3.2 Microarray Gene Expression -- 19.4 Neural Network-based Clustering -- 19.5 Mixture Model-based Clustering -- 19.5.1 Examples of Finite Mixture Models -- 19.5.2 Examples of Infinite Mixture Models -- 19.6 Graph-based Clustering -- 19.7 Consensus Clustering -- 19.8 Biclustering -- 19.9 Summary -- References -- Part Five Validation and Visualisation -- Chapter 20 Numerical Validation -- 20.1 Introduction -- 20.2 External Criteria -- 20.2.1 Rand Index -- 20.2.2 Adjusted Rand Index -- 20.2.3 Jaccard Index -- 20.2.4 Normalised Mutual Information -- 20.3 Internal Criteria -- 20.3.1 Adjusted Figure of Merit -- 20.3.2 CLEST -- 20.4 Relative Criteria -- 20.4.1 Minimum Description Length -- 20.4.2 Minimum Message Length -- 20.4.3 Bayesian Information Criterion.
20.4.4 Akaikeś Information Criterion.
Abstract:
Clustering techniques are increasingly being put to use in the analysis of high-throughput biological datasets. Novel computational techniques to analyse high throughput data in the form of sequences, gene and protein expressions, pathways, and images are becoming vital for understanding diseases and future drug discovery. This book details the complete pathway of cluster analysis, from the basics of molecular biology to the generation of biological knowledge. The book also presents the latest clustering methods and clustering validation, thereby offering the reader a comprehensive review of clustering analysis in bioinformatics from the fundamentals through to state-of-the-art techniques and applications. Key Features: Offers a contemporary review of clustering methods and applications in the field of bioinformatics, with particular emphasis on gene expression analysis Provides an excellent introduction to molecular biology with computer scientists and information engineering researchers in mind, laying out the basic biological knowledge behind the application of clustering analysis techniques in bioinformatics Explains the structure and properties of many types of high-throughput datasets commonly found in biological studies Discusses how clustering methods and their possible successors would be used to enhance the pace of biological discoveries in the future Includes a companion website hosting a selected collection of codes and links to publicly available datasets.
Local Note:
Electronic reproduction. Ann Arbor, Michigan : ProQuest Ebook Central, 2017. Available via World Wide Web. Access may be limited to ProQuest Ebook Central affiliated libraries.
Genre:
Electronic Access:
Click to View