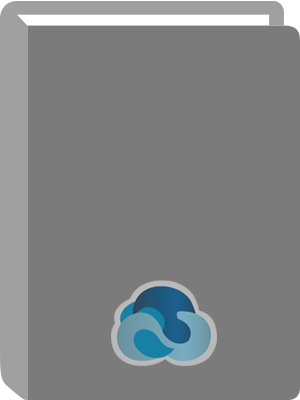
Chaos : From Simple Models to Complex Systems.
Title:
Chaos : From Simple Models to Complex Systems.
Author:
Cencini, Massimo.
ISBN:
9789814277662
Personal Author:
Physical Description:
1 online resource (482 pages)
Series:
Series on Advances in Statistical Mechanics ; v.17
Series on Advances in Statistical Mechanics
Contents:
Contents -- Preface -- Introduction -- Historical note -- Overview of the book -- Hints on how to use/read this book -- Introduction to Dynamical Systems and Chaos -- 1. First Encounter with Chaos -- 1.1 Prologue -- 1.2 The nonlinear pendulum -- 1.3 The damped nonlinear pendulum -- 1.4 The vertically driven and damped nonlinear pendulum -- 1.5 What about the predictability of pendulum evolution? -- 1.6 Epilogue -- 2. The Language of Dynamical Systems -- 2.1 Ordinary Differential Equations (ODE) -- 2.1.1 Conservative and dissipative dynamical systems -- BoxB.1 Hamiltonian dynamics -- A: Symplectic structure and Canonical Transformations -- B: Integrable systems and Action-Angle variables -- 2.1.2 PoincaréMap -- 2.2 Discrete time dynamical systems: maps -- 2.2.1 Two dimensional maps -- 2.2.1.1 The Hénon Map -- 2.2.1.2 Two-dimensional symplectic maps -- 2.3 The role of dimension -- 2.4 Stability theory -- 2.4.1 Classification of fixed points and linear stability analysis -- BoxB.2 A remark on the linear stability of symplectic maps -- 2.4.2 Nonlinear stability -- 2.4.2.1 Limit cycles -- 2.4.2.2 Lyapunov Theorem -- 2.5 Exercises -- 3. Examples of Chaotic Behaviors -- 3.1 The logisticmap -- BoxB.3 Topological conjugacy -- 3.2 The Lorenzmodel -- BoxB.4 Derivation of the Lorenz model -- 3.3 The Hénon-Heiles system -- 3.4 What did we learn and what will we learn? -- BoxB.5 Correlation functions -- 3.5 Closing remark -- 3.6 Exercises -- 4. Probabilistic Approach to Chaos -- 4.1 An informal probabilistic approach -- 4.2 Time evolution of the probability density -- BoxB.6 Markov Processes -- A: Finite states Markov Chains -- B: Continuous Markov processes -- C: Dynamical systems with additive noise -- 4.3 Ergodicity -- 4.3.1 An historical interlude on ergodic theory -- BoxB.7 Poincaré recurrence theorem.
4.3.2 Abstract formulation of the Ergodic theory -- 4.4 Mixing -- 4.5 Markov chains and chaoticmaps -- 4.6 Natural measure -- 4.7 Exercises -- 5. Characterization of Chaotic Dynamical Systems -- 5.1 Strange attractors -- 5.2 Fractals and multifractals -- 5.2.1 Box counting dimension -- 5.2.2 The stretching and folding mechanism -- 5.2.3 Multifractals -- BoxB.8 Brief excursion on Large Deviation Theory -- 5.2.4 Grassberger-Procaccia algorithm -- 5.3 Characteristic Lyapunov exponents -- BoxB.9 Algorithm for computing Lyapunov Spectrum -- 5.3.1 Oseledec theorem and the law of large numbers -- 5.3.2 Remarks on the Lyapunov exponents -- 5.3.2.1 Lyapunov exponents are topological invariant -- 5.3.2.2 Relationship between Lyapunov exponents of flows and Poincaré maps -- 5.3.3 Fluctuation statistics of finite time Lyapunov exponents -- 5.3.4 Lyapunov dimension -- BoxB.10 Mathematical chaos -- A: Hyperbolic sets and Anosov systems -- B: SRB measure -- C: The Arnold cat map -- 5.4 Exercises -- 6. From Order to Chaos in Dissipative Systems -- 6.1 The scenarios for the transition to turbulence -- 6.1.1 Landau-Hopf -- BoxB.11 Hopf bifurcation -- BoxB.12 The Van der Pol oscillator and the averaging technique -- 6.1.2 Ruelle-Takens -- 6.2 The period doubling transition -- 6.2.1 Feigenbaum renormalization group -- 6.3 Transition to chaos through intermittency: Pomeau-Manneville scenario -- 6.4 Amathematical remark -- 6.5 Transition to turbulence in real systems -- 6.5.1 A visit to laboratory -- 6.6 Exercises -- 7. Chaos in Hamiltonian Systems -- 7.1 The integrability problem -- 7.1.1 Poincaré and the non-existence of integrals of motion -- 7.2 Kolmogorov-Arnold-Moser theorem and the survival of tori -- BoxB.13 Arnold diffusion -- 7.3 Poincaré-Birkho. theorem and the fate of resonant tori -- 7.4 Chaos around separatrices -- BoxB.14 The resonance-overlap criterion.
7.5 Melnikov's theory -- 7.5.1 An application to the Duffing's equation -- 7.6 Exercises -- Advanced Topics and Applications: From Information Theory to Turbulence -- 8. Chaos and Information Theory -- 8.1 Chaos, randomness and information -- 8.2 Information theory, coding and compression -- 8.2.1 Information sources -- 8.2.2 Properties and uniqueness of entropy -- 8.2.3 Shannon entropy rate and its meaning -- BoxB.15 Transient behavior of block-entropies -- 8.2.4 Coding and compression -- 8.3 Algorithmic complexity -- BoxB.16 Ziv-Lempel compression algorithm -- 8.4 Entropy and complexity in chaotic systems -- 8.4.1 Partitions and symbolic dynamics -- 8.4.2 Kolmogorov-Sinai entropy -- BoxB.17 Rényi entropies -- 8.4.3 Chaos, unpredictability and uncompressibility -- 8.5 Concluding remarks -- 8.6 Exercises -- 9. Coarse-Grained Information and Large Scale Predictability -- 9.1 Finite-resolution versus in.nite-resolution descriptions -- 9.2 ε-entropy in information theory: lossless versus lossy coding -- 9.2.1 Channel capacity -- 9.2.2 Rate distortion theory -- BoxB.18 ε-entropy for the Bernoulli and Gaussian source -- 9.3 ε-entropy in dynamical systems and stochastic processes -- 9.3.1 Systems classification according to e-entropy behavior -- BoxB.19 ε-entropy from exit-times statistics -- 9.4 The finite size lyapunov exponent (FSLE) -- 9.4.1 Linear vs nonlinear instabilities -- 9.4.2 Predictability in systems with different characteristic times -- 9.5 Exercises -- 10. Chaos in Numerical and Laboratory Experiments -- 10.1 Chaos in silico -- BoxB.20 Round-off errors and floating-point representation -- 10.1.1 Shadowing lemma -- 10.1.2 The effects of state discretization -- BoxB.21 Effect of discretization: a probabilistic argument -- 10.2 Chaos detection in experiments -- 10.2.1 Practical difficulties -- 10.2.1.1 Choice of delay time.
10.2.1.2 Choice of the embedding dimension -- 10.2.1.3 The necessary amount of data -- 10.2.1.4 Role of noise -- BoxB.22 Lyapunov exponents from experimental data -- 10.3 Can chaos be distinguished from noise? -- 10.3.1 The finite resolution analysis -- 10.3.2 Scale-dependent signal classiffication -- 10.3.3 Chaos or noise? A puzzling dilemma -- 10.3.3.1 Indeterminacy due to finite resolution -- 10.3.3.2 Indeterminacy due to fnite block length effects -- 10.4 Prediction andmodeling from data -- 10.4.1 Data prediction -- 10.4.2 Data modeling -- 11. Chaos in Low Dimensional Systems -- 11.1 Celestial mechanics -- 11.1.1 The restricted three-body problem -- 11.1.2 Chaos in the Solar system -- 11.1.2.1 The chaotic motion of Hyperion -- 11.1.2.2 Asteroids -- BoxB.23 A symplectic map for Halley comet -- 11.1.2.3 Long time behavior of the Solar system -- 11.2 Chaos and transport phenomena in fluids -- BoxB.24 Chaos and passive scalar transport -- 11.2.1 Lagrangian chaos -- 11.2.1.1 Eulerian vs Lagrangian chaos -- 11.2.1.2 Lagrangian chaos in point-vortex systems -- BoxB.25 Point vortices and the two-dimensional Euler equation -- 11.2.1.3 Lagrangian Chaos in the ABC flow -- 11.2.2 Chaos and diffusion in laminar flows -- 11.2.2.1 Transport in a model of the Gulf Stream -- 11.2.2.2 Standard and Anomalous diffusion in a chaotic model of transport -- BoxB.26 Relative dispersion in turbulence -- 11.2.3 Advection of inertial particles -- 11.3 Chaos in population biology and chemistry -- 11.3.1 Population biology: Lotka-Volterra systems -- 11.3.2 Chaos in generalized Lotka-Volterra systems -- 11.3.3 Kinetics of chemical reactions: Belousov-Zhabotinsky -- BoxB.27 Michaelis-Menten law of simple enzymatic reaction -- 11.3.4 Chemical clocks -- BoxB.28 A model for biochemical oscillations -- 11.4 Synchronization of chaotic systems.
11.4.1 Synchronization of regular oscillators -- 11.4.2 Phase synchronization of chaotic oscillators -- 11.4.3 Complete synchronization of chaotic systems -- 12. Spatiotemporal Chaos -- 12.1 Systems and models for spatiotemporal chaos -- 12.1.1 Overview of spatiotemporal chaotic systems -- 12.1.1.1 Reaction diffusion systems -- 12.1.1.2 Rayleigh Bénard convection -- 12.1.1.3 Complex Ginzburg-Landau and Kuramoto-Sivanshisky equations -- 12.1.1.4 Coupled map lattices -- 12.1.1.5 Nonlinear lattices: the Fermi-Pasta-Ulam model -- 12.1.1.6 Fully developed turbulence -- 12.1.1.7 Delayed ordinary differential equations -- 12.1.2 Networks of chaotic systems -- 12.2 The thermodynamic limit -- 12.3 Growth and propagation of space-time perturbations -- 12.3.1 An overview -- 12.3.2 "Spatial" and "Temporal" Lyapunov exponents -- 12.3.3 The comoving Lyapunov exponent -- 12.3.4 Propagation of perturbations -- BoxB.29 Stable chaos and supertransients -- 12.3.5 Convective chaos and sensitivity to boundary conditions -- 12.4 Non-equilibrium phenomena and spatiotemporal chaos -- BoxB.30 Non-equilibrium phase transitions -- Directed Percolation -- Multiplicative Noise -- Kardar-Parisi-Zhang equation and surface roughening -- 12.4.1 Spatiotemporal perturbations and interfaces roughening -- 12.4.2 Synchronization of extended chaotic systems -- 12.4.3 Spatiotemporal intermittency -- 12.5 Coarse-grained description of high dimensional chaos -- 12.5.1 Scale-dependent description of high-dimensional systems -- 12.5.2 Macroscopic chaos: low dimensional dynamics embedded in high dimensional chaos -- 13. Turbulence as a Dynamical System Problem -- 13.1 Fluids as dynamical systems -- 13.2 Statistical mechanics of ideal fluids and turbulence phenomenology -- 13.2.1 Three dimensional ideal fluids -- 13.2.2 Two dimensional ideal fluids.
13.2.3 Phenomenology of three dimensional turbulence.
Abstract:
Chaos: from simple models to complex systems aims to guide science and engineering students through chaos and nonlinear dynamics from classical examples to the most recent fields of research. The first part, intended for undergraduate and graduate students, is a gentle and self-contained introduction to the concepts and main tools for the characterization of deterministic chaotic systems, with emphasis to statistical approaches. The second part can be used as a reference by researchers as it focuses on more advanced topics including the characterization of chaos with tools of information theory and applications encompassing fluid and celestial mechanics, chemistry and biology. The book is novel in devoting attention to a few topics often overlooked in introductory textbooks and which are usually found only in advanced surveys such as: information and algorithmic complexity theory applied to chaos and generalization of Lyapunov exponents to account for spatiotemporal and non-infinitesimal perturbations. The selection of topics, numerous illustrations, exercises and proposals for computer experiments make the book ideal for both introductory and advanced courses. Sample Chapter(s). Introduction (164 KB). Chapter 1: First Encounter with Chaos (1,323 KB). Contents: First Encounter with Chaos; The Language of Dynamical Systems; Examples of Chaotic Behaviors; Probabilistic Approach to Chaos; Characterization of Chaotic Dynamical Systems; From Order to Chaos in Dissipative Systems; Chaos in Hamiltonian Systems; Chaos and Information Theory; Coarse-Grained Information and Large Scale Predictability; Chaos in Numerical and Laboratory Experiments; Chaos in Low Dimensional Systems; Spatiotemporal Chaos; Turbulence as a Dynamical System Problem; Chaos and Statistical Mechanics: Fermi-Pasta-Ulam a Case Study. Readership: Students and researchers in science
(physics, chemistry, mathematics, biology) and engineering.
Local Note:
Electronic reproduction. Ann Arbor, Michigan : ProQuest Ebook Central, 2017. Available via World Wide Web. Access may be limited to ProQuest Ebook Central affiliated libraries.
Genre:
Electronic Access:
Click to View