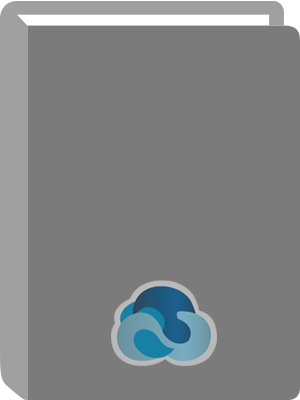
Bayesian Methods in Cosmology.
Title:
Bayesian Methods in Cosmology.
Author:
Hobson, Michael P.
ISBN:
9780511767081
Personal Author:
Physical Description:
1 online resource (317 pages)
Contents:
Cover -- Half-title -- Title -- Copyright -- Contents -- List of contributors -- Preface -- Part I Methods -- 1 Foundations and algorithms -- 1.1 Rational inference -- 1.2 Foundations -- 1.2.1 Lattices -- 1.2.2 Measure -- Addition -- Assignment -- Multiplication -- Commutativity -- 1.2.3 Information -- 1.2.4 Probability -- 1.3 Inference -- 1.3.1 Bayes' theorem -- 1.3.2 Prior probability -- Informal -- Symmetry -- Maximum entropy -- Continuous problems -- Geometry -- 1.4 Algorithms -- 1.4.1 Nested sampling -- Theory -- Kernel -- Uncertainty -- Exploring the prior -- Exploring the constraint -- Posterior -- 1.4.2 Simulated annealing -- Theory -- Uncertainty -- Schedule -- Exploration -- Posterior -- 1.4.3 Comparison -- 1.5 Concluding remarks -- References -- 2 Simple applications of Bayesian methods -- 2.1 Introduction -- 2.2 Essentials of modern cosmology -- 2.2.1 Standard rulers and candles -- 2.2.2 Motivation -- 2.3 Theorists and pre-processed data -- 2.4 Experimentalists and raw measurements -- 2.5 Concluding remarks -- References -- 3 Parameter estimation using Monte Carlo sampling -- 3.1 Why do sampling? -- 3.2 How do I get the samples? -- 3.2.1 Direct sampling methods -- 3.2.2 Problems with large dimensions -- 3.2.3 Markov chain sampling -- 3.2.4 Metropolis-Hastings algorithm -- 3.2.5 Other sampling methods -- 3.2.6 Thermodynamic and flat-histogram methods -- 3.2.7 Baby and toy -- 3.3 Have I taken enough samples yet? -- 3.4 What do I do with the samples? -- 3.4.1 Parameter constraints -- 3.4.2 Importance sampling -- 3.4.3 Inference from simulation -- 3.4.4 Model selection as parameter estimation -- 3.5 Conclusions -- References -- 4 Model selection and multi-model inference -- 4.1 Introduction -- 4.2 Levels of Bayesian inference -- 4.3 The Bayesian framework -- 4.3.1 Priors -- 4.3.2 Information and complexity.
4.4 Computing the Bayesian evidence -- 4.4.1 General Monte Carlo methods -- 4.4.2 Restricted Monte Carlo methods -- 4.4.3 Approximate methods -- 4.5 Interpretational scales -- 4.6 Applications -- 4.6.1 Applications to real data -- The spectral tilt -- Dark energy -- Other applications -- 4.6.2 Forecasting and survey design -- 4.7 Conclusions -- References -- 5 Bayesian experimental design and model selection forecasting -- 5.1 Introduction -- 5.2 Predicting the effectiveness of future experiments -- 5.2.1 Utility, expected utility and optimization -- 5.2.2 Choosing the best experiment - an example -- 5.3 Experiment optimization for error reduction -- 5.3.1 Fisher matrix error forecast -- 5.3.2 Utility functions for error minimization -- 5.3.3 Application to cosmology: optimization of the WFMOS survey -- 5.4 Experiment optimization for model selection -- 5.4.1 Quantifying experimental capabilities using Bayes factors -- 5.4.2 Application: dark energy vs. a cosmological constant -- 5.5 Predicting the outcome of model selection -- 5.5.1 Predictive distributions -- 5.5.2 Predictive posterior odds distribution -- 5.5.3 Application: spectral index from the Planck satellite -- 5.6 Summary -- References -- 6 Signal separation in cosmology -- 6.1 Model of the data -- 6.2 The hidden, visible and data spaces -- 6.3 Parameterization of the hidden space -- 6.3.1 Mixing matrix -- 6.3.2 Component fields -- 6.3.3 Linear and non-linear parameters -- 6.4 Choice of data space -- 6.4.1 Pixel-domain data space -- 6.4.2 Fourier-domain data space -- 6.5 Applying Bayes' theorem -- 6.5.1 Defining the posterior distribution -- 6.6 Non-blind signal separation -- 6.6.1 Wiener filtering -- WF posterior -- Optimal values and error estimates -- 6.6.2 Harmonic-space maximum-entropy method -- Harmonic-space MEM posterior -- Optimal values and error estimates.
Relationship between the MEM and WF -- Determination of the regularization constant -- Iterative updating of the signal covariance matrix -- Accommodation of spatially varying noise and spectral parameters -- 6.6.3 Mixed-space maximum-entropy method -- 6.7 (Semi-)blind signal separation -- 6.7.1 Pixel-domain parameter estimation -- Uncorrelated signals and noise -- Correlated signals and noise -- 6.7.2 Independent component analysis (ICA) -- SMICA data model -- SMICA likelihood -- SMICA priors -- SMICA posterior -- 6.7.3 Correlated component analysis (CCA) -- 6.7.4 Determining the optimal number of components -- References -- Part II Applications -- 7 Bayesian source extraction -- 7.1 Traditional approaches -- 7.2 The Bayesian approach -- 7.2.1 Discrete sources in a background -- 7.2.2 Bayesian inference -- 7.2.3 Defining the posterior distribution -- 7.3 Variable-source-number models -- 7.4 Fixed-source-number models -- 7.5 Single-source models -- 7.5.1 Analytic source extraction and the matched filter -- 7.5.2 Iterative source extraction: local maximization -- 7.5.3 Iterative source extraction: global maximization -- 7.5.4 Simultaneous source extraction -- 7.5.5 Pixel-by-pixel source extraction -- 7.6 Conclusions -- Acknowledgments -- References -- 8 Flux measurement -- 8.1 Introduction -- 8.2 Photometric measurements -- 8.3 Classical flux estimation -- 8.4 The source population -- 8.5 Bayesian flux inference -- 8.6 The faintest sources -- 8.7 Practical flux measurement -- References -- 9 Gravitational wave astronomy -- 9.1 A new spectrum -- 9.2 Gravitational wave data analysis -- 9.2.1 The traditional approach -- 9.3 The Bayesian approach -- 9.3.1 Parameter estimation -- 9.3.2 Search strategies -- 9.3.3 Model selection -- References -- 10 Bayesian analysis of cosmic microwave background data -- 10.1 Introduction.
10.2 The CMB as a hierarchical model -- 10.2.1 CMB data -- 10.2.2 From detectors to maps -- Destripers -- Deconvolution mapmaking -- Noise marginalization -- Wiener filters -- 10.2.3 From maps to power spectra -- 10.2.4 From spectra to cosmological parameters -- 10.3 Polarization -- Power spectra and E/B separation -- 10.4 Complications -- 10.4.1 Foregrounds and systematics -- 10.4.2 Non-Gaussianity -- 10.5 Conclusions -- References -- 11 Bayesian multilevel modelling of cosmological populations -- 11.1 Introduction -- 11.2 Galaxy distance indicators -- 11.2.1 The calibration problem -- 11.2.2 The estimation problem -- 11.2.3 Applications of galaxy distance indicators -- 11.3 Multilevel models -- 11.3.1 Adjusting source estimates: shrinkage -- 11.3.2 Poisson point process multilevel models -- 11.4 Future directions -- Acknowledgments -- References -- 12 A Bayesian approach to galaxy evolution studies -- 12.1 Discovery space -- 12.2 Average versus maximum likelihood -- 12.3 Priors and Malmquist/Eddington bias -- 12.4 Small samples -- 12.5 Measuring a width in the presence of a contaminating population -- 12.6 Fitting a trend in the presence of outliers -- 12.7 What is the number returned by tests such as… -- 12.8 Summary -- References -- 13 Photometric redshift estimation: methods and applications -- 13.1 Introduction -- 13.2 Template methods -- 13.3 Bayesian methods and non-colour priors -- 13.4 Training methods and neural networks -- 13.5 Errors on photo-z -- 13.6 Optimal filters -- 13.7 Comparison of photo-z codes -- 13.8 The role of spectroscopic datasets -- 13.9 Synergy with cosmological probes -- 13.10 Discussion -- References -- Index.
Abstract:
Comprehensive introduction to Bayesian methods in cosmological studies, for graduate students and researchers in cosmology, astrophysics and applied statistics.
Local Note:
Electronic reproduction. Ann Arbor, Michigan : ProQuest Ebook Central, 2017. Available via World Wide Web. Access may be limited to ProQuest Ebook Central affiliated libraries.
Subject Term:
Genre:
Electronic Access:
Click to View