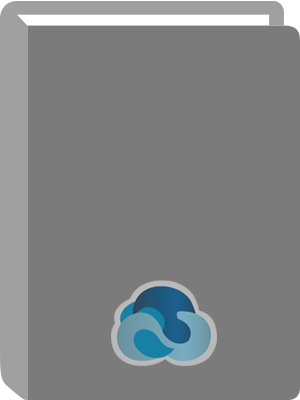
Nonlinear System Identification : NARMAX Methods in the Time, Frequency, and Spatio-Temporal Domains.
Title:
Nonlinear System Identification : NARMAX Methods in the Time, Frequency, and Spatio-Temporal Domains.
Author:
Billings, Stephen A.
ISBN:
9781118535547
Personal Author:
Edition:
1st ed.
Physical Description:
1 online resource (607 pages)
Contents:
Nonlinear System Identification: NARMAX Methods in the Time, Frequency, and Spatio-Tempora Domains -- Copyright -- Contents -- Preface -- 1 Introduction -- 1.1 Introduction to System Identification -- 1.1.1 System Models and Simulation -- 1.1.2 Systems and Signals -- 1.1.3 System Identification -- 1.2 Linear System Identification -- 1.3 Nonlinear System Identification -- 1.4 NARMAX Methods -- 1.5 The NARMAX Philosophy -- 1.6 What is System Identification For? -- 1.7 Frequency Response of Nonlinear Systems -- 1.8 Continuous-Time, Severely Nonlinear, and Time-Varying Models and Systems -- 1.9 Spatio-temporal Systems -- 1.10 Using Nonlinear System Identification in Practice and Case Study Examples -- References -- 2 Models for Linear and Nonlinear Systems -- 2.1 Introduction -- 2.2 Linear Models -- 2.2.1 Autoregressive Moving Average with Exogenous Input Model -- 2.2.1.1 FIR Model -- 2.2.1.2 AR Model -- 2.2.1.3 MA Model -- 2.2.1.4 ARMA Model -- 2.2.1.5 ARX Model -- 2.2.1.6 ARMAX Model -- 2.2.1.7 Box-Jenkins Model -- 2.2.2 Parameter Estimation for Linear Models -- 2.2.2.1 ARX Model Parameter Estimation - The Least Squares Algorithm -- 2.2.2.2 ARMAX Model Parameter Estimation - The Extended Least Squares Algorithm -- 2.3 Piecewise Linear Models -- 2.3.1 Spatial Piecewise Linear Models -- 2.3.1.1 Operating Regions -- 2.3.1.2 Parameter Estimation -- 2.3.1.3 Simulation Example -- 2.3.2 Models with Signal-Dependent Parameters -- 2.3.2.1 Decomposition of Signal-Dependent Models -- 2.3.2.2 Parameter Estimation of Signal-Dependent Models -- 2.3.2.3 Simulation Example -- 2.3.3 Remarks on Piecewise Linear Models -- 2.4 Volterra Series Models -- 2.5 Block-Structured Models -- 2.5.1 Parallel Cascade Models -- 2.5.2 Feedback Block-Structured Models -- 2.6 NARMAX Models -- 2.6.1 Polynomial NARMAX Model -- 2.6.2 Rational NARMAX Model -- 2.6.2.1 Integral Model.
2.6.2.2 Recursive Model -- 2.6.2.3 Output-affine Model -- 2.6.3 The Extended Model Set Representation -- 2.7 Generalised Additive Models -- 2.8 Neural Networks -- 2.8.1 Multi-layer Networks -- 2.8.2 Single-Layer Networks -- 2.8.2.1 Activation Functions -- 2.8.2.2 Radial Basis Function Networks -- 2.9 Wavelet Models -- 2.9.1 Dynamic Wavelet Models -- 2.9.1.1 Random Noise -- 2.9.1.2 Coloured Noise -- 2.10 State-Space Models -- 2.11 Extensions to the MIMO Case -- 2.12 Noise Modelling -- 2.12.1 Noise-Free -- 2.12.2 Additive Random Noise -- 2.12.3 Additive Coloured Noise -- 2.12.4 General Noise -- 2.13 Spatio-temporal Models -- References -- 3 Model Structure Detection and Parameter Estimation -- 3.1 Introduction -- 3.2 The Orthogonal Least Squares Estimator and the Error Reduction Ratio -- 3.2.1 Linear-in-the-Parameters Representation -- 3.2.2 The Matrix Form of the Linear-in-the-Parameters Representation -- 3.2.3 The Basic OLS Estimator -- 3.2.4 The Matrix Formulation of the OLS Estimator -- 3.2.5 The Error Reduction Ratio -- 3.2.6 An Illustrative Example of the Basic OLS Estimator -- 3.3 The Forward Regression OLS Algorithm -- 3.3.1 Forward Regression with OLS -- 3.3.1.1 The FROLS Algorithm -- 3.3.1.2 Variants of the FROLS Algorithm -- 3.3.2 An Illustrative Example of Forward Regression with OLS -- 3.3.3 The OLS Estimation Engine and Identification Procedure -- 3.4 Term and Variable Selection -- 3.5 OLS and Sum of Error Reduction Ratios -- 3.5.1 Sum of Error Reduction Ratios -- 3.5.2 The Variance of the s -Step-Ahead Prediction Error -- 3.5.3 The Final Prediction Error -- 3.5.4 The Variable Selection Algorithm -- 3.6 Noise Model Identification -- 3.6.1 The Noise Model -- 3.6.2 A Simulation Example with Noise Modelling -- 3.7 An Example of Variable and Term Selection for a Real Data Set -- 3.8 ERR is Not Affected by Noise.
3.9 Common Structured Models to Accommodate Different Parameters -- 3.10 Model Parameters as a Function of Another Variable -- 3.10.1 System Internal and External Parameters -- 3.10.2 Parameter-Dependent Model Structure -- 3.10.3 Modelling Auxetic Foams - An Example of External Parameter-Dependent Model Identification -- 3.11 OLS and Model Reduction -- 3.12 Recursive Versions of OLS -- References -- 4 Feature Selection and Ranking -- 4.1 Introduction -- 4.2 Feature Selection and Feature Extraction -- 4.3 Principal Components Analysis -- 4.4 A Forward Orthogonal Search Algorithm -- 4.4.1 The Basic Idea of the FOS-MOD Algorithm -- 4.4.2 Feature Detection and Ranking -- 4.4.3 Monitoring the Search Procedure -- 4.4.4 Illustrative Examples -- 4.5 A Basis Ranking Algorithm Based on PCA -- 4.5.1 Principal Component-Derived Multiple Regression -- 4.5.2 PCA-Based MFROLS Algorithms -- 4.5.3 An Illustrative Example -- References -- 5 Model Validation -- 5.1 Introduction -- 5.2 Detection of Nonlinearity -- 5.3 Estimation and Test Data Sets -- 5.4 Model Predictions -- 5.4.1 One-Step-Ahead Prediction -- 5.4.2 Model Predicted Output -- 5.5 Statistical Validation -- 5.5.1 Correlation Tests for Input-Output Models -- 5.5.2 Correlation Tests for Time Series Models -- 5.5.3 Correlation Tests for MIMO Models -- 5.5.4 Output-Based Tests -- 5.6 Term Clustering -- 5.7 Qualitative Validation of Nonlinear Dynamic Models -- 5.7.1 Poincaré Sections -- 5.7.2 Bifurcation Diagrams -- 5.7.3 Cell Maps -- 5.7.4 Qualitative Validation in Nonlinear System Identification -- 5.7.4.1 Poincaré Maps for Model Validation -- 5.7.4.2 Bifurcation Diagrams for Model Validation -- 5.7.4.3 Poincaré Maps and Bifurcation Diagrams for Model Validation of Chaotic Systems -- References -- 6 The Identification and Analysis of Nonlinear Systems in the Frequency Domain -- 6.1 Introduction.
6.2 Generalised Frequency Response Functions -- 6.2.1 The Volterra Series Representation of Nonlinear Systems -- 6.2.1.1 The Volterra Series -- 6.2.1.2 Volterra Series Models of Continuous- and Discrete-Time Nonlinear Systems -- 6.2.2 Generalised Frequency Response Functions -- 6.2.3 The Relationship Between GFRFs and Output Response of Nonlinear Systems -- 6.2.3.1 The System Time Domain Output Response Representation Using GFRFs -- 6.2.3.2 The Relationship Between GFRFs and the System Frequency Domain Output Response -- 6.2.4 Interpretation of the Composition of the Output Frequency Response of Nonlinear Systems -- 6.2.5 Estimation and Computation of GFRFs -- 6.2.5.1 Multi-dimensional Spectral Estimation Approaches -- 6.2.5.2 Frequency-Domain Volterra System Identification Approaches -- 6.2.5.3 Parametric Model-Based Approach -- 6.2.6 The Analysis of Nonlinear Systems Using GFRFs -- 6.2.6.1 Summary of the Parametric Method of Estimating GFRFs -- 6.2.6.2 Case Study of a Real System -- 6.3 Output Frequencies of Nonlinear Systems -- 6.3.1 Output Frequencies of Nonlinear Systems under Multi-tone Inputs -- 6.3.2 Output Frequencies of Nonlinear Systems for General Inputs -- 6.4 Nonlinear Output Frequency Response Functions -- 6.4.1 Definition and Properties of NOFRFs -- 6.4.2 Evaluation of NOFRFs -- 6.4.3 Damage Detection Using NARMAX Modelling and NOFRF-Based Analysis -- 6.4.3.1 Basic Idea -- 6.4.3.2 Damage Detection Procedure -- 6.4.3.3 An Experimental Case Study -- 6.5 Output Frequency Response Function of Nonlinear Systems -- 6.5.1 Definition of the OFRF -- 6.5.2 Determination of the OFRF -- 6.5.2.1 Determining the OFRF Structure -- 6.5.2.2 Determining the OFRF `Coefficients' -- 6.5.3 Application of the OFRF to Analysis of Nonlinear Damping for Vibration Control -- References.
7 Design of Nonlinear Systems in the Frequency Domain - Energy Transfer Filters and Nonlinear Damping -- 7.1 Introduction -- 7.2 Energy Transfer Filters -- 7.2.1 The Time and Frequency Domain Representation of the NARX Model with Input Nonlinearity -- 7.2.2 Energy Transfer Filter Designs -- 7.2.2.1 The Problem Description -- 7.2.2.2 ETF Design for a Specified Input -- 7.2.2.3 ETF Designs Using Orthogonal Least Squares -- 7.2.2.4 ETF Design for Several Specified Inputs -- 7.3 Energy Focus Filters -- 7.3.1 Output Frequencies of Nonlinear Systems with Input Signal Energy Located in Two Separate Frequency Intervals -- 7.3.2 The Energy Focus Filter Design Procedure and an Example -- 7.4 OFRF -Based Approach for the Design of Nonlinear Systems in the Frequency Domain -- 7.4.1 OFRF -Based Design of Nonlinear Systems in the Frequency Domain -- 7.4.1.1 General Procedure for the OFRF -Based Design of Nonlinear Systems in the Frequency Domain -- 7.4.2 Design of Nonlinear Damping in the Frequency Domain for Vibration Isolation: An Experimental Study -- 7.4.2.1 Experimental Setup -- 7.4.2.2 Modelling the Experimental Vibration Isolation System -- 7.4.2.3 The OFRF -Based Design for Nonlinear Damping -- References -- 8 Neural Networks for Nonlinear System Identification -- 8.1 Introduction -- 8.2 The Multi-layered Perceptron -- 8.3 Radial Basis Function Networks -- 8.3.1 Training Schemes for RBF Networks -- 8.3.2 Fixed Kernel Centres with a Single Width -- 8.3.3 Limitation of RBF Networks with a Single Kernel Width -- 8.3.4 Fixed Kernel Centres and Multiple Kernel Widths -- 8.4 Wavelet Networks -- 8.4.1 Wavelet Decompositions -- 8.4.2 Wavelet Networks -- 8.4.3 Limitations of Fixed Grid Wavelet Networks -- 8.4.4 A New Class of Wavelet Networks -- 8.4.4.1 The Structure of the New Wavelet Networks -- 8.4.4.2 Determining the Number of Candidate Wavelet Terms.
8.4.4.3 Determining Significant Wavelet Terms.
Abstract:
Nonlinear System Identification: NARMAX Methods in the Time, Frequency, and Spatio-Temporal Domains describes a comprehensive framework for the identification and analysis of nonlinear dynamic systems in the time, frequency, and spatio-temporal domains. This book is written with an emphasis on making the algorithms accessible so that they can be applied and used in practice. Includes coverage of: The NARMAX (nonlinear autoregressive moving average with exogenous inputs) model The orthogonal least squares algorithm that allows models to be built term by term where the error reduction ratio reveals the percentage contribution of each model term Statistical and qualitative model validation methods that can be applied to any model class Generalised frequency response functions which provide significant insight into nonlinear behaviours A completely new class of filters that can move, split, spread, and focus energy The response spectrum map and the study of sub harmonic and severely nonlinear systems Algorithms that can track rapid time variation in both linear and nonlinear systems The important class of spatio-temporal systems that evolve over both space and time Many case study examples from modelling space weather, through identification of a model of the visual processing system of fruit flies, to tracking causality in EEG data are all included to demonstrate how easily the methods can be applied in practice and to show the insight that the algorithms reveal even for complex systems NARMAX algorithms provide a fundamentally different approach to nonlinear system identification and signal processing for nonlinear systems. NARMAX methods provide models that are transparent, which can easily be analysed, and which can be used to solve real problems. This book is intended for graduates, postgraduates and researchers in the sciences and engineering,
and also for users from other fields who have collected data and who wish to identify models to help to understand the dynamics of their systems.
Local Note:
Electronic reproduction. Ann Arbor, Michigan : ProQuest Ebook Central, 2017. Available via World Wide Web. Access may be limited to ProQuest Ebook Central affiliated libraries.
Genre:
Added Author:
Electronic Access:
Click to View