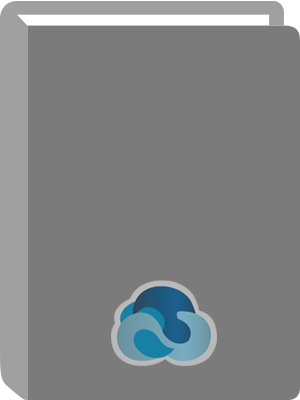
Multiscale Modeling A Bayesian Perspective
Title:
Multiscale Modeling A Bayesian Perspective
Author:
Ferreira, Marco A. R. author.
ISBN:
9780387708980
Personal Author:
Physical Description:
XII, 245 p. online resource.
Series:
Springer Series in Statistics,
Contents:
Models for Spatial Data -- Illustrative Example -- Convolutions and Wavelets -- Convolution Methods -- Wavelet Methods -- Explicit Multiscale Models -- Overview of Explicit Multiscale Models -- Gaussian Multiscale Models on Trees -- Hidden Markov Models on Trees -- Mass-Balanced Multiscale Models on Trees -- Multiscale Random Fields -- Multiscale Time Series -- Change of Support Models -- Implicit Multiscale Models -- Implicit Computationally Linked Model Overview -- Metropolis-Coupled Methods -- Genetic Algorithms -- Case Studies -- Soil Permeability Estimation -- Single Photon Emission Computed Tomography Example -- Conclusions.
Abstract:
A wide variety of processes occur on multiple scales, either naturally or as a consequence of measurement. This book contains methodology for the analysis of data that arise from such multiscale processes. The book brings together a number of recent developments and makes them accessible to a wider audience. Taking a Bayesian approach allows for full accounting of uncertainty, and also addresses the delicate issue of uncertainty at multiple scales. The Bayesian approach also facilitates the use of knowledge from prior experience or data, and these methods can handle different amounts of prior knowledge at different scales, as often occurs in practice. The book is aimed at statisticians, applied mathematicians, and engineers working on problems dealing with multiscale processes in time and/or space, such as in engineering, finance, and environmetrics. The book will also be of interest to those working on multiscale computation research. The main prerequisites are knowledge of Bayesian statistics and basic Markov chain Monte Carlo methods. A number of real-world examples are thoroughly analyzed in order to demonstrate the methods and to assist the readers in applying these methods to their own work. To further assist readers, the authors are making source code (for R) available for many of the basic methods discussed herein. Marco A. R. Ferreira is an Assistant Professor of Statistics at the University of Missouri, Columbia. Herbert K. H. Lee is an Associate Professor of Applied Mathematics and Statistics at the University of California, Santa Cruz, and authored the book Bayesian Nonparametrics via Neural Networks.
Added Author:
Added Corporate Author:
Electronic Access:
http://dx.doi.org/10.1007/978-0-387-70898-0