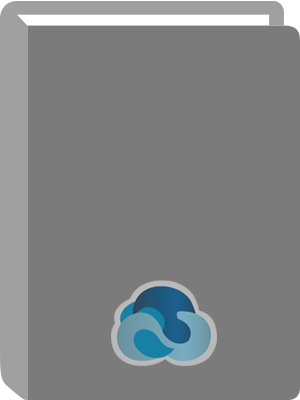
Template Matching Techniques in Computer Vision : Theory and Practice.
Title:
Template Matching Techniques in Computer Vision : Theory and Practice.
Author:
Brunelli, Roberto.
ISBN:
9780470744048
Personal Author:
Edition:
1st ed.
Physical Description:
1 online resource (350 pages)
Contents:
Template Matching Techniques in Computer Vision -- CONTENTS -- Preface -- 1 Introduction -- 1.1 Template Matching and Computer Vision -- 1.2 The Book -- 1.3 Bibliographical Remarks -- References -- 2 The Imaging Process -- 2.1 Image Creation -- 2.1.1 Light -- 2.1.2 Gathering Light -- 2.1.3 Diffraction-limited Systems -- 2.1.4 Quantum Noise -- 2.2 Biological Eyes -- 2.2.1 The Human Eye -- 2.2.2 Alternative Designs -- 2.3 Digital Eyes -- 2.4 Digital Image Representations -- 2.4.1 The Sampling Theorem -- 2.4.2 Image Resampling -- 2.4.3 Log-polar Mapping -- 2.5 Bibliographical Remarks -- References -- 3 Template Matching as Testing -- 3.1 Detection and Estimation -- 3.2 Hypothesis Testing -- 3.2.1 The Bayes Risk Criterion -- 3.2.2 The Neyman-Pearson Criterion -- 3.3 An Important Example -- 3.4 A Signal Processing Perspective: Matched Filters -- 3.5 Pattern Variability and the Normalized Correlation Coefficient -- 3.6 Estimation -- 3.6.1 Maximum Likelihood Estimation -- 3.6.2 Bayes Estimation -- 3.6.3 James-Stein Estimation -- 3.7 Bibliographical Remarks -- References -- 4 Robust Similarity Estimators -- 4.1 Robustness Measures -- 4.2 M-estimators -- 4.3 L1 Similarity Measures -- 4.4 Robust Estimation of Covariance Matrices -- 4.5 Bibliographical Remarks -- References -- 5 Ordinal Matching Measures -- 5.1 Ordinal Correlation Measures -- 5.1.1 Spearman Rank Correlation -- 5.1.2 Kendall Correlation -- 5.1.3 Bhat-Nayar Correlation -- 5.2 Non-parametric Local Transforms -- 5.2.1 The Census and Rank Transforms -- 5.2.2 Incremental Sign Correlation -- 5.3 Bibliographical Remarks -- References -- 6 Matching Variable Patterns -- 6.1 Multiclass Synthetic Discriminant Functions -- 6.2 Advanced Synthetic Discriminant Functions -- 6.3 Non-orthogonal Image Expansion -- 6.4 Bibliographical Remarks -- References -- 7 Matching Linear Structure: The Hough Transform.
7.1 Getting Shapes: Edge Detection -- 7.2 The Radon Transform -- 7.3 The Hough Transform: Line and Circle Detection -- 7.4 The Generalized Hough Transform -- 7.5 Bibliographical Remarks -- References -- 8 Low-dimensionality Representations and Matching -- 8.1 Principal Components -- 8.1.1 Probabilistic PCA -- 8.1.2 How Many Components? -- 8.2 A Nonlinear Approach: Kernel PCA -- 8.3 Independent Components -- 8.4 Linear Discriminant Analysis -- 8.4.1 Bayesian Dual Spaces -- 8.5 A Sample Application: Photographic-quality Facial Composites -- 8.6 Bibliographical Remarks -- References -- 9 Deformable Templates -- 9.1 A Dynamic Perspective on the Hough Transform -- 9.2 Deformable Templates -- 9.3 Active Shape Models -- 9.4 Diffeomorphic Matching -- 9.5 Bibliographical Remarks -- References -- 10 Computational Aspects of Template Matching -- 10.1 Speed -- 10.1.1 Early Jump-out -- 10.1.2 The Use of Sum Tables -- 10.1.3 Hierarchical Template Matching -- 10.1.4 Metric Inequalities -- 10.1.5 The FFT Advantage -- 10.1.6 PCA-based Speed-up -- 10.1.7 A Combined Approach -- 10.2 Precision -- 10.2.1 A Perturbative Approach -- 10.2.2 Phase Correlation -- 10.3 Bibliographical Remarks -- References -- 11 Matching Point Sets: The Hausdorff Distance -- 11.1 Metric Pattern Spaces -- 11.2 Hausdorff Matching -- 11.3 Efficient Computation of the Hausdorff Distance -- 11.4 Partial Hausdorff Matching -- 11.5 Robustness Aspects -- 11.6 A Probabilistic Perspective -- 11.7 Invariant Moments -- 11.8 Bibliographical Remarks -- References -- 12 Support Vector Machines and Regularization Networks -- 12.1 Learning and Regularization -- 12.2 RBF Networks -- 12.2.1 RBF Networks for Gender Recognition -- 12.3 Support Vector Machines -- 12.3.1 Improving Efficiency -- 12.3.2 Multiclass SVMs -- 12.3.3 Best Practice -- 12.4 Bibliographical Remarks -- References -- 13 Feature Templates.
13.1 Detecting Templates by Features -- 13.2 Parametric Feature Manifolds -- 13.3 Multiclass Pattern Rejection -- 13.4 Template Features -- 13.5 Bibliographical Remarks -- References -- 14 Building a Multibiometric System -- 14.1 Systems -- 14.2 The Electronic Librarian -- 14.3 Score Integration -- 14.4 Rejection -- 14.5 Bibliographical Remarks -- References -- Appendices -- A AnImAl: A Software Environment for Fast Prototyping -- A.1 AnImAl: An Image Algebra -- A.2 Image Representation and Processing Abstractions -- A.3 The AnImAl Environment -- A.4 Bibliographical Remarks -- References -- B Synthetic Oracles for Algorithm Development -- B.1 Computer Graphics -- B.2 Describing Reality: Flexible Rendering Languages -- B.3 Bibliographical Remarks -- References -- C On Evaluation -- C.1 A Note on Performance Evaluation -- C.2 Training a Classifier -- C.3 Analyzing the Performance of a Classifier -- C.4 Evaluating a Technology -- C.5 Bibliographical Remarks -- References -- Index.
Abstract:
The detection and recognition of objects in images is a key research topic in the computer vision community. Within this area, face recognition and interpretation has attracted increasing attention owing to the possibility of unveiling human perception mechanisms, and for the development of practical biometric systems. This book and the accompanying website, focus on template matching, a subset of object recognition techniques of wide applicability, which has proved to be particularly effective for face recognition applications. Using examples from face processing tasks throughout the book to illustrate more general object recognition approaches, Roberto Brunelli: examines the basics of digital image formation, highlighting points critical to the task of template matching; presents basic and advanced template matching techniques, targeting grey-level images, shapes and point sets; discusses recent pattern classification paradigms from a template matching perspective; illustrates the development of a real face recognition system; explores the use of advanced computer graphics techniques in the development of computer vision algorithms. Template Matching Techniques in Computer Vision is primarily aimed at practitioners working on the development of systems for effective object recognition such as biometrics, robot navigation, multimedia retrieval and landmark detection. It is also of interest to graduate students undertaking studies in these areas.
Local Note:
Electronic reproduction. Ann Arbor, Michigan : ProQuest Ebook Central, 2017. Available via World Wide Web. Access may be limited to ProQuest Ebook Central affiliated libraries.
Genre:
Electronic Access:
Click to View