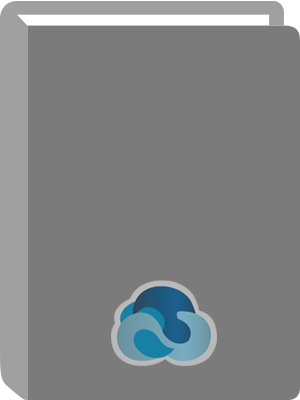
Measuring Operational and Reputational Risk : A Practitioner's Approach.
Title:
Measuring Operational and Reputational Risk : A Practitioner's Approach.
Author:
Soprano, Aldo.
ISBN:
9780470742112
Personal Author:
Edition:
1st ed.
Physical Description:
1 online resource (227 pages)
Series:
The Wiley Finance Ser. ; v.562
The Wiley Finance Ser.
Contents:
Measuring Operational and Reputational Risk -- Contents -- Foreword by Andrea Sironi -- Preface -- Acknowledgments -- 1 The Development of ORM in UniCredit Group -- 1.1 A brief history of a fast-growing group -- 1.2 Creating a new function -- 1.3 Developing the new control system -- 1.4 Challenges in the early stages -- 1.5 Methodology to measure operational risk -- 1.6 Training and internal communication focus -- 1.7 International regulatory challenges -- 1.8 Reputational risk management -- 2 The Calculation Dataset -- 2.1 Definitions -- 2.2 Rules of thumb -- 2.3 Internal loss data -- 2.3.1 Business line mapping -- 2.3.2 Event type classifications -- 2.3.3 Data quality analysis -- 2.3.4 Special cases -- 2.4 Minimum loss threshold -- 2.5 External data -- 2.5.1 Public or external data sources -- 2.5.2 Consortium data -- 2.5.3 Scenario data -- 2.6 Business environment and internal control factors -- 2.7 Scenarios -- 2.8 Insurance information -- 2.9 Scaling data -- 2.10 The Unicredit Group Operational Risk database evolution -- 2.11 Final considerations -- 3 Loss Distribution Approaches -- 3.1 Calculation dataset building -- 3.1.1 Internal calculation dataset -- 3.1.2 External calculation dataset -- 3.1.3 Scenario-generated calculation dataset -- 3.1.4 Risk indicators calculation dataset -- 3.2 General LDA framework -- 3.3 Operational risk classes -- 3.3.1 Identically distributed risk classes -- 3.3.2 Inflation adjustment -- 3.3.3 Data independence -- 3.4 Parametric estimation and goodness-of-fit techniques -- 3.4.1 Severity distributions -- 3.4.2 Graphical methods -- 3.4.3 Analytical methods -- 3.4.4 Frequency distributions -- 3.5 Applying extreme value theory -- 3.6 g-and-h distribution theory -- 3.7 Calculating operational capital at risk -- 3.7.1 Loss severity distribution -- 3.7.2 Loss frequency distribution -- 3.7.3 Annual loss distribution.
3.7.4 Single class capital at risk -- 3.8 Insurance modeling -- 3.8.1 Appropriate haircuts reflecting the policy's declining residual term -- 3.8.2 Payment uncertainty -- 3.8.3 Counterparty risk -- 3.8.4 Application of insurance -- 3.9 Adjustment for risk indicators -- 3.10 Operational risk classes aggregation -- 3.10.1 Copulae functions -- 3.10.2 Elliptical copulae -- 3.10.3 Archimedean copulae -- 3.10.4 Choice of copula -- 3.10.5 Correlation coefficients -- 3.11 The closed-form approximation for OpVaR -- 3.11.1 Effect of the minimum threshold on capital at risk -- 3.12 Confidence band for capital at risk -- 3.13 Stress testing -- 3.14 Loss data minimum threshold setting -- 3.15 Empirical application on Algo OpData -- 3.15.1 Descriptive statistics -- 3.15.2 Autocorrelation analysis -- 3.15.3 Capital at risk estimates using parametric models -- 3.15.4 Capital at risk estimates using EVT -- 3.15.5 Capital at risk estimates using the g-and-h distribution -- 3.15.6 Capital at risk estimates considering correlation -- 3.16 Regulatory capital requirement -- 3.16.1 The consolidated capital requirement -- 3.16.2 The individual capital requirement -- 3.17 Economic capital requirement -- 3.18 Integration of operational risk in the budgeting process -- 4 Analyzing Insurance Policies -- 4.1 Insurance management and risk transfer -- 4.2 Qualifying criteria in the Basel 2 capital framework -- 4.2.1 Rating of the insurance company -- 4.2.2 Duration and residual term of the insurance contract -- 4.2.3 Policy termination requisites -- 4.2.4 Claims reimbursement uncertainty and ineffective coverage -- 4.2.5 Conclusions -- 4.3 A practical application to traditional insurance -- 4.3.1 Insurance policies to cover financial institutions' operational risks -- 4.3.2 Operational event types and available insurance coverage -- 5 Managing Reputational Risk.
5.1 Introducing reputational risk -- 5.2 A financial institution's reputational risk exposure -- 5.3 Managing reputational risk: a matter of policy -- 5.4 Reputational risk measurement -- 5.4.1 Reputational risk as a function of share price volatility -- 5.4.2 Measuring reputational risk using scenarios -- 5.4.3 Scoring-card-based models for reputational risk assessment -- 5.5 A recent example of reputational event -- 5.5.1 A description of the event -- 5.5.2 Background -- 5.5.3 How the fake trading occurred -- 5.5.4 The discovery and first reactions -- 5.5.5 Measures planned and taken -- 5.5.6 Immediate consequences for SocGen -- 5.5.7 Reputational issues and comments -- 5.5.8 The lessons learned - what can we do to avoid being next? -- 5.5.9 Psychological, 'soft' factors -- 5.5.10 Control instruments -- 5.5.11 Managing data and signals -- 6 Conclusions -- References -- Further reading -- Index.
Abstract:
How to apply operational risk theory to real-life banking data Modelling Operational and Reputational Risks shows practitioners the best models to use in a given situation, according to the type of risk an organization is facing. Based on extensive applied research on operational risk models using real bank datasets, it offers a wide range of various testing models and fitting techniques for financial practitioners. With this book, professionals will have a foundation for measuring and predicting these important intangibles. Aldo Soprano (Madrid, Spain) is Group Head of operational risk management at UniCredit Group.
Local Note:
Electronic reproduction. Ann Arbor, Michigan : ProQuest Ebook Central, 2017. Available via World Wide Web. Access may be limited to ProQuest Ebook Central affiliated libraries.
Genre:
Electronic Access:
Click to View