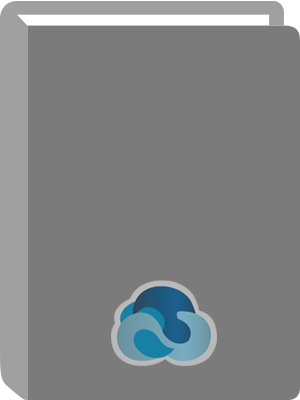
Sales history-based demand prediction by using generalized linear models
Başlık:
Sales history-based demand prediction by using generalized linear models
Yazar:
Özenboy, Başar, author.
Yazar Ek Girişi:
Fiziksel Tanımlama:
xii, 101 leaves:+ 1 computer laser optical disc.
Özet:
Improved data collection and storage capabilities make vast amounts of data available in appropriate formats. Commercial enterprises store their sales data. It’s vital for companies to accurately predict demand by utilizing the existing sales data. Such predictive analytics is a crucial part of their decision support systems to increase the profitability of the company. In predictive data analytics, the branch of regression modeling commonly is used to predict a numerical response variable like sales amount. In recent years, generalized linear models provide a generalization to better address the specificities of the problem at hand. To begin with, they relax the assumption of normally distributed error terms. Moreover, the relationship of the set of predictor variables and the response variable could be represented by a set of link functions rather than the sole choice of the identity function. This thesis models the sales amount prediction problem through the use of generalized linear models. Unique company sales data are explored and fitted accordingly with the right distribution function of the response variable along with an appropriate link function. The experimental results are compared with the other regression models, classification algorithms, and time series models. The model selection is performed via the use of MSE and AIC metrics respectively.
Yazar Ek Girişi:
Tüzel Kişi Ek Girişi:
Tek Biçim Eser Adı:
Thesis (Master)--İzmir Institute of Technology: Computer Engineering.
İzmir Institute of Technology: Computer Engineering--Thesis (Master).
Elektronik Erişim:
Access to Electronic Versiyon.