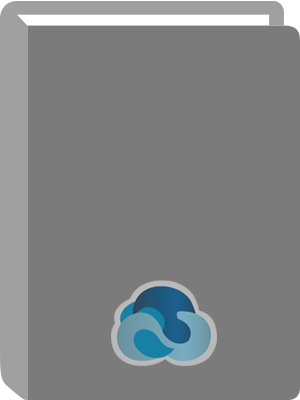
Improved image based localization using semantic descriptors
Başlık:
Improved image based localization using semantic descriptors
Yazar:
Çınaroğlu, İbrahim, author.
Yazar Ek Girişi:
Fiziksel Tanımlama:
xiv, 98 leaves: color illustrations, charts;+ 1 computer laser optical disc.
Özet:
Place recognition and Visual Localization (VL) for autonomous driving are the topics that keep their popularity in the field of Computer Vision. In this study, semantically improved Hybrid-VL approaches, that use localization aware semantic information in street-level driving images are proposed. Initially, Semantic Descriptor (SD) is extracted from semantically segmented images with a Convolutional Neural Network (CNN) trained for localization task. Then, image retrieval based VL task is performed using the approximate nearest neighbor search (ANNS) in 2D-2D matching context. This proposed method is named as SD-VL and its success is compared with the success of the state-of-the-art Local Descriptor (LD) based VL method (LD-VL) which is frequently used in the literature. Furthermore, with the aim of alleviating the shortcomings of both two methods, a novel decision-level Hybrid-VL (Hybrid-VLDL) method is proposed by combining SD-VL and LD-VL in post-processing stage. Also feature-level Hybrid-VL (Hybrid-VLFL) method is proposed in order to produce automatically tuned hybrid result. These proposed VL methods are examined on two challenging benchmarks; RobotCar Seasons and Malaga Downtown Data Sets. Moreover, a new VL data set Malaga Streetview Challenge is generated by collecting Google Streetview images on the same path of Malaga Downtown in order to observe impact of environmental and wide-baseline changes. This newly generated test set will be useful for researchers studying in this field. After all, the proposed semantically boosted Hybrid-VLDL method is able to increase localization performance on both RobotCar Seasons and Malaga Streetview Challenge data sets by 11.6% and 4.5% Top-1 recall@5, and 4% and 5.4% recall@1 scores respectively. Additionally, reliability of our hyper-parameter (W) based Hybrid-VLDL approach is supported by very close performance of the Hybrid-VLFL method.
Yazar Ek Girişi:
Tüzel Kişi Ek Girişi:
Tek Biçim Eser Adı:
Thesis (Doctoral)--İzmir Institute of Technology:Computer Engineering.
İzmir Institute of Technology:Computer Engineering. --Thesis (Doctoral).
Elektronik Erişim:
Access to Electronic Versiyon.