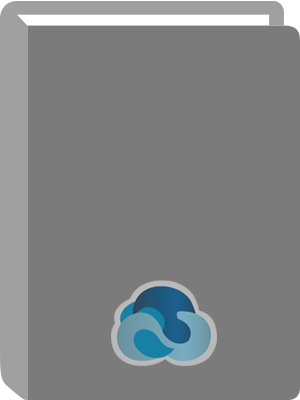
Hierarchical image classification with self-supervised vision transformer features
Başlık:
Hierarchical image classification with self-supervised vision transformer features
Yazar:
Karagüler, Caner, author.
Yazar Ek Girişi:
Fiziksel Tanımlama:
x, 53 leaves: charts;+ 1 computer laser optical disc.
Özet:
There are lots of works about image classification and most of them are based on convolutional neural networks (CNN). In image classification, some classes are more difficult to distinguish than others because of non-even visual separability. These difficult classes require domain-specific classifiers but traditional convolutional neural networks are trained as flat N-way classifiers. These flat classifiers can not leverage the hierarchical information of the classes well. To solve this issue, researchers proposed new techniques that embeds class-hierarchy into the convolutional neural networks and most of these techniques exceed existing convolutional neural networks' success rates on large-scale datasets like ImageNet. In this work, we questioned if a hierarchical image classification with self- supervised vision transformer features can exceed hierarchical convolutional neural networks. During this work, we used a hierarchical ETHEC dataset and extract attention features with the help of vision transformers. Using these attention features, we implemented 3 different hierarchical classification approaches and compared the results with CNN alternative of our approaches.
Yazar Ek Girişi:
Tüzel Kişi Ek Girişi:
Tek Biçim Eser Adı:
Thesis (Master)--İzmir Institute of Technology:Computer Engineering.
İzmir Institute of Technology: Energy Engineering --Thesis (Master).
Elektronik Erişim:
Access to Electronic Versiyon.