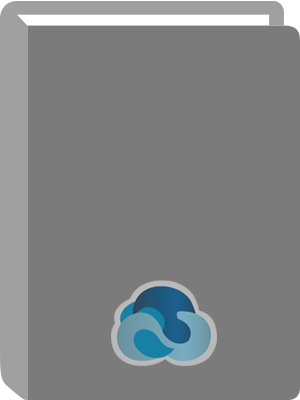
A mutation-based approach to alleviate the class imbalance problem in software defect prediction
Başlık:
A mutation-based approach to alleviate the class imbalance problem in software defect prediction
Yazar:
Güner, Dinçer, author.
Yazar Ek Girişi:
Fiziksel Tanımlama:
x, 119 leaves: charts;+ 1 computer laser optical disc.
Özet:
Highly imbalanced training datasets considerably degrade the performance of software defect predictors. Software Defect Prediction (SDP) datasets have a general problem, which is class imbalance. Therefore, a variety of methods have been developed to alleviate Class Imbalance Problem (CIP). However, these classical methods, like datasampling, balance datasets without connecting any relation with SDP. Over-sampling techniques generate synthetic minor class instances, which generalize a small number of minor class instances and result in less diverse instances, whereas under-sampling techniques eliminate major class instances, resulting in significant information loss. In this study, we present an approach that uses software mutations to balance software repositories. Mutation-based Approach (MBA) injects mutants into defect-free instances, causing them to transform into defective instances. In this way, MBA balances datasets with diverse data produced by mutation operators, and there is no loss on instances as in under-sampling. For recall scores, almost all rebalancing methods outperformed Baseline in Interrelease Defect Prediction (IRDP) scenario but only MBA significantly outperformed Baseline in Cross-project Defect Prediction (CPDP) scenario. The performance increase in recall resulted in the production of more false alarms. We can not generalize that MBA outperforms Baseline and the five over-sampling strategies in terms of AUC scores. In terms of recall values, the MBA performed better in CPDP than IRDP. For both IRDP and CPDP scenarios, there were significant and positive correlations between SMC (the change percentage of software measures) and recall, and SMC and false alarm but there was no significant correlation between SMC and AUC.
Tüzel Kişi Ek Girişi:
Tek Biçim Eser Adı:
Thesis (Master)--İzmir Institute of Technology:Computer Engineering.
İzmir Institute of Technology: Computer Engineering--Thesis (Master).
Elektronik Erişim:
Access to Electronic Versiyon.