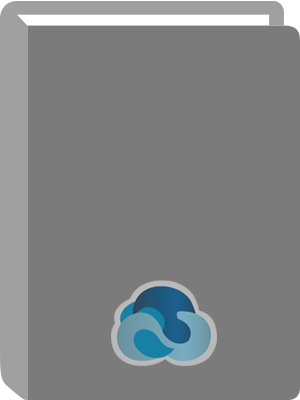
Automatic Treatment and Analysis of Learner Corpus Data.
Başlık:
Automatic Treatment and Analysis of Learner Corpus Data.
Yazar:
Díaz-Negrillo, Ana.
ISBN:
9789027270955
Yazar Ek Girişi:
Fiziksel Tanımlama:
1 online resource (320 pages)
Seri:
Studies in Corpus Linguistics ; v.59
Studies in Corpus Linguistics
İçerik:
Automatic Treatment and Analysis of Learner Corpus Data -- Editorial page -- Title page -- LCC data -- Table of contents -- Section 1. Introduction -- Introduction -- References -- Learner corpora -- 1. Introduction -- 2. Corpora types, processing and annotation -- 2.1 Types of learner corpora -- 2.2 Annotation -- 3. Uses and users of learner corpus data -- 3.1 Overview -- 3.2 Foreign language teaching -- 3.3 Second language acquisition research -- 3.4 Corpus and computational linguistics -- 4. Looking forwards -- References -- Section 2. Compilation, annotation and exchangeability of learner corpus data -- Developing corpus interoperability for phonetic investigation of learner corpora -- 1. Introduction -- 2. Processing and annotating spoken data -- 2.1 A tentative typology of spoken learner corpora -- 2.2 Existing annotation layers in phonetic corpora, corpus comparability and interoperability -- 2.3 Comparing with native corpora -- 3. Some of the limits of automatisation -- 3.1 The limits of phonetic annotation (forced alignments) -- 3.2 Some syllabification issues -- 3.3 Prosodic annotation -- 3.4 Speaker-dependent models? -- 3.5 The uses of automation (caveats) -- 4. Challenges and recommendations -- 4.1 Tokenisation and categorisation of realisations and learner phonetic errors -- 4.2 Modelling -- 4.3 Comparing with native data (corpus interoperability) -- 5. From spoken learner corpora to spoken learner databases -- 5.1 Textual datasets -- 5.2 XML and XML tools -- 5.3 Working with customized interface of Praat -- 5.4 An alternative stance: WinPitch -- 5.5 An incoming mixed model? -- 6. The advent of spoken databases vs. speech databases -- References -- Learner corpora and second language acquisition -- 1. Introduction -- 2. Learner corpora in SLA research -- 2.1 A bias in second language research.
2.2 Corpora in language acquisition research -- 2.3 An overview of learner corpora and learner corpus research -- 2.4 L2 Spanish learner corpora: Introducing CEDEL2 -- 3. Design principles in learner corpora for SLA purposes: CEDEL2, a case study -- 3.1 Principle 1. Content selection -- 3.2 Principle 2. Representativeness -- 3.3 Principle 3. Contrast -- 3.4 Principle 4. Structural criteria -- 3.5 Principle 5. Annotation -- 3.6 Principle 6. Sample size -- 3.7 Principle 7. Documentation -- 3.8 Principle 8. Balance -- 3.9 Principle 9. Topic -- 3.10 Principle 10. Homogeneity -- 3.11 Conclusion -- 4. Current status of CEDEL2 -- 4.1 Data collection -- 4.2 Data distribution -- 4.3 Source of data -- 4.4 Preliminary segmentation and annotation -- 4.5 CEDEL2: Next steps -- 5. Learner corpora: The way forward -- 6. Conclusion -- References -- Appendices -- Competing target hypotheses in the Falko corpus -- 1. Introduction: Why corpus architecture matters -- 2. What kind of information should a learner corpus provide and what kind of data is needed? -- 2.1 POS & lemmas -- 2.2 Target hypotheses -- Error exponent -- Conflicting spans -- 2.3 Stand-off models -- 3. Case study: Falko -- 3.1 Target hypotheses in the Falko essay corpus -- 3.2 Automatic error tagging -- 3.3 Manual error tagging -- 3.4 Parsing learner data -- 4. Summary -- References -- Section 3. Automatic approaches to the identification of learner language features in learner corpus data -- Using learner corpora for automatic error detection and correction -- 1. Introduction -- 2. System development guided by annotated learner corpora -- 2.1 Cambridge Learner Corpus -- 2.2 Distribution of errors in CLC -- 2.3 Morphological confusions and errors -- 3. Training data for machine-learned systems -- 3.1 Statistical methods for learner error detection -- 3.2 Using well-formed data for training.
3.3 Using annotated learner data for training -- 4. Evaluation of ESL error detection systems -- 4.1 Evaluation metrics -- 4.2 Annotation issues -- 4.3 Future directions for evaluation and annotation -- 5. Summary -- References -- Automatic suprasegmental parameter extraction in learner corpora -- 1. Introduction -- 1.1 Corpus -- 1.2 Automatic parameter extraction -- 2. Benchmarking automatic against manual segmentation -- 2.1 Duration of read passages -- 2.2 Speech rate -- 2.3 Vowel duration -- 3. Alternative suprasegmental parameters and automatic classification -- 3.1 Voiced (VO) vs. voiceless (UV) intervals -- 3.2 Pitch extraction -- 3.3 Intensity -- 4. Automatic classification -- 5. Discussion and conclusion -- References -- Criterial feature extraction using parallel learner corpora and machine learning -- 1. Introduction -- 2. Method -- 2.1 The corpora -- 2.2 Problems of manual error-tagging -- 2.3 Parallel corpora and edit distance -- 2.4 Extractions of errors and data analysis -- 3. Results 1: Correspondence Analysis and Variability-based Neighbour Clustering -- 3.1 Distributions of surface taxonomy errors -- 3.2 Correspondence analysis over the different error types across school years -- 3.3 Refining the analysis by using Variability-based Neighbour Clustering -- 4. Results 2: Random forest -- 4.1 Extraction of addition and omission errors -- 4.2 Results of random forest -- 5. Discussion -- References -- Section 4. Analysis of learner corpus data -- Phonological acquisition in the French-English interlanguage -- 1. Background -- 2. Subsets and predictions -- 3. Method and results -- 3.1 Subset 1 (S1) -- 3.2 Subset 2 (S2) -- 3.3 Subset S3 (S3) -- 4. Conclusion -- References -- Prosody in a contrastive learner corpus -- 1. Introduction -- 2. ANGLISH: A learner corpus -- 2.1 Constitution and description: Objectives.
2.2 Conception and design -- 3. Experiment -- 3.1 Data -- 3.2 Method -- 3.3 Results -- 4. Discussion -- 5. Conclusion -- References -- A corpus-based comparison of syntactic complexity in NNS and NS university students' writing -- 1. Introduction -- 2. Syntactic complexity in second language writing -- 3. Measuring L2 syntactic complexity -- 4. Method -- 4.1 Data -- 4.2 Research questions -- 4.3 Analysis -- 5. Results and discussion -- 5.1 Research question 1 -- 5.2 Research question 2 -- 6. Conclusions and implications -- References -- Analysing coherence in upper-intermediate learner writing -- 1. Introduction -- 2. Conceptualizations of coherence in learner writing -- 3. Coherence and cohesion - A close match? -- 3.1 Rhetorical Structure Theory (RST) as a model for the analysis of coherence -- 3.2 RST and learner writing -- 4. The study - Using RST to analyse coherence in learner writing -- 4.1 Data -- 4.2 Method -- 4.3 Initial results -- 5. Potential and limitations of using RST for the analysis of coherence in learner writing -- 6. Conclusion and outlook -- References -- Statistical tests for the analysis of learner corpus data -- 1. Introduction -- 1.1 General introduction -- 1.2 A very brief view on caveats regarding learner corpus research -- 1.3 The corpus data: The genitive alternation (of- vs. s-genitives) -- 2. Elementary statistical tests -- 2.1 Two-dimensional frequency tables: Chi-squared tests -- 2.2 Measures of central tendency -- 3. A primer on multifactorial methods: Logistic regression -- 3.1 Logistic regressions with two categorical independent variables -- 3.2 Logistic regressions with one categorical and one numeric independent variable -- 4. Concluding remarks -- 4.1 Conditional inference trees: An alternative to regressions -- 4.2 Pointers to additional references -- References -- Index.
Özet:
This paper is an overview of several basic statistical tools in corpus-based SLA research. I first discuss a few issues relevant to the analysis of learner corpus data. Then, I illustrate a few widespread quantitative techniques and statistical visualizations and exemplify them on the basis of corpus data on the genitive alternation - the of-genitive vs. the s-genitive from German learners and native speakers of English. The statistical methods discussed include a test for differences between frequencies (the chi-squared test), tests for differences between means/medians (the U-test), and a more advanced multifactorial extension, binary logistic regression.
Notlar:
Electronic reproduction. Ann Arbor, Michigan : ProQuest Ebook Central, 2017. Available via World Wide Web. Access may be limited to ProQuest Ebook Central affiliated libraries.
Tür:
Elektronik Erişim:
Click to View