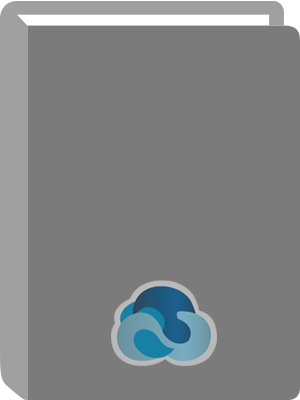
Comparable Corpora and Computer-assisted Translation.
Başlık:
Comparable Corpora and Computer-assisted Translation.
Yazar:
Delpech , Estelle Maryline.
ISBN:
9781119002529
Yazar Ek Girişi:
Basım Bilgisi:
1st ed.
Fiziksel Tanımlama:
1 online resource (305 pages)
Seri:
ISTE
İçerik:
Cover -- Title Page -- Copyright -- Contents -- Acknowledgments -- Introduction -- PART 1: Applicative and Scientific Context -- Chapter 1: Leveraging Comparable Corpora for Computer-assisted Translation -- 1.1. Introduction -- 1.2. From the beginnings of machine translation to comparable corpora processing -- 1.2.1. The dawn of machine translation -- 1.2.2. The development of computer-assisted translation -- 1.2.3. Drawbacks of parallel corpora and advantages of comparable corpora -- 1.2.4. Difficulties of technical translation -- 1.2.5. Industrial context -- 1.3. Term alignment from comparable corpora: a state-of-the-art -- 1.3.1. Distributional approach principle -- 1.3.2. Term alignment evaluation -- 1.3.2.1. Precision at rank N or TopN -- 1.3.2.2. MRR -- 1.3.2.3. MAP -- 1.3.3. Improvement and variants of the distributional approach -- 1.3.3.1. Favoring distributional symmetry -- 1.3.3.2. Using syntactic contexts -- 1.3.3.3. Relying on trusted elements -- 1.3.3.4. Improving semantic information representation -- 1.3.3.5. Using second-order semantic affinities -- 1.3.3.6. Improving the bilingual resource with semantic classes -- 1.3.3.7. Translating polylexical units -- 1.3.4. Influence of data and parameters on alignment quality -- 1.3.4.1. Data -- 1.3.4.2. Parameters -- 1.3.5. Limits of the distributional approach -- 1.4. CAT software prototype for comparable corpora processing -- 1.4.1. Implementation of a term alignment method -- 1.4.1.1. Implementation and data -- 1.4.1.2. Extraction of the terms to be aligned -- 1.4.1.3. Collecting context vectors -- 1.4.1.3.1. Monolexical term context vectors -- 1.4.1.4. Polylexical term context vectors -- 1.4.1.5. Translation of the source context vectors -- 1.4.1.6. Term alignment -- 1.4.2. Terminological records extraction -- 1.4.3. Lexicon consultation interface -- 1.5. Summary.
Chapter 2: User-Centered Evaluation of Lexicons Extracted from Comparable Corpora -- 2.1. Introduction -- 2.2. Translation quality evaluation methodologies -- 2.2.1. Machine translation evaluation -- 2.2.1.1. Automatic evaluation measures -- 2.2.1.2. Human MT evaluation -- 2.2.2. Human translation evaluation -- 2.2.2.1. Quantitative models -- 2.2.2.2. Non-quantitative models -- 2.2.3. Discussion -- 2.3. Design and experimentation of a user-centered evaluation -- 2.3.1. Methodological aspects -- 2.3.1.1. Evaluation criteria and purpose -- 2.3.1.2. Subject matter expertise -- 2.3.1.3. Basis for comparison -- 2.3.2. Experimentation protocol -- 2.3.2.1. Data -- 2.3.2.1.1. Comparable corpora and extracted lexica -- 2.3.2.1.2. Texts to be translated -- 2.3.2.1.3. Resources used in the translation situation -- 2.3.2.1.4. Translators and judges -- 2.3.2.2. Evaluation progress -- 2.3.2.2.1. Translation phase -- 2.3.2.2.2. Translation quality evaluation phase -- 2.3.3. Results -- 2.3.3.1. Lexicons usability -- 2.3.3.1.1. Translation speed -- 2.3.3.1.2. Use of resources -- 2.3.3.1.3. Translators' impressions on the lexicons extracted from comparable corpora -- 2.3.3.2. Quality of the generated translations -- 2.3.3.2.1. Inter-annotator agreement -- 2.3.3.2.2. Judgment task -- 2.3.3.2.3. Ranking task -- 2.3.3.3. Lexicon coverage -- 2.3.3.4. Reproducing the protocol on a wider scale -- 2.4. Discussion -- Chapter 3: Automatic Generation of Term Translations -- 3.1. Introduction -- 3.2. Compositional approaches -- 3.2.1. Compositional translation principle -- 3.2.2. Polylexical units compositional translation -- 3.2.2.1. Lexical variation and multiple decomposition -- 3.2.2.2. Morphological relations -- 3.2.2.3. Extracting translations from mixed documents -- 3.2.2.4. Bag of equivalents -- 3.2.2.5. Hybridization with distributional method.
3.2.3. Monolexical units compositional translation -- 3.2.3.1. Translation of words formed by means of prefixation -- 3.2.3.2. Translation of words formed by means of neoclassical compounding -- 3.2.3.3. Translation of word compounds -- 3.2.4. Candidate translation filtering -- 3.2.4.1. Looking for an attestation -- 3.2.4.2. Filtering based on context similarities -- 3.2.4.3. Supervised learning filtering -- 3.3. Data-driven approaches -- 3.3.1. Analogy-based translation -- 3.3.2. Rewriting rules learning -- 3.3.3. Dealing with morphological variation -- 3.4. Evaluation of term translator generation methods -- 3.5. Research perspectives -- PART 2: Contributions to Compositional Translation -- Chapter 4: Morph-Compositional Translation: Methodological Framework -- 4.1. Introduction -- 4.2. Morpho-compositional translation method -- 4.2.1. Scientific positioning -- 4.2.2. Definitions and terminology -- 4.2.2.1. Polylexical units -- 4.2.2.2. Monolexical unit -- 4.2.2.3. Word -- 4.2.2.4. Complex word -- 4.2.2.5. Morpheme -- 4.2.2.6. Simple word or free morpheme -- 4.2.2.7. Bound morpheme -- 4.2.2.8. Prefix -- 4.2.2.9. Combining forms -- 4.2.2.10. Suffix -- 4.2.2.11. Notations -- 4.2.3. Underlying assumptions -- 4.2.3.1. Compositional meaning -- 4.2.3.2. Compositional translation -- 4.2.3.3. Fertility -- 4.2.3.4. Distortion -- 4.2.3.5. Lexical divergence -- 4.2.3.6. Morphological variation -- 4.2.4. Advantages of the proposed approach for processing comparable corpora -- 4.3. Issues addressed and contributions -- 4.3.1. Generating fertile translations -- 4.3.1.1. Semantic fertility -- 4.3.1.2. Surface fertility -- 4.3.2. Dealing with diverse morphological structures -- 4.3.2.1. Word compounding composition -- 4.3.2.2. Neoclassical compounding -- 4.3.2.3. Prefixation -- 4.3.2.4. Suffixation -- 4.3.3. Candidate translations ranking.
4.4. Evaluation methodology -- 4.4.1. A priori reference -- 4.4.2. A posteriori reference -- 4.5. Conclusion -- Chapter 5: Experimental Data -- 5.1. Introduction -- 5.2. Comparable corpora -- 5.3. Source terms -- 5.4. Reference data for translation generation evaluation -- 5.4.1. A priori reference -- 5.4.2. A posteriori reference -- 5.4.2.1. Exact -- 5.4.2.2. Acceptable -- 5.4.2.3. Close -- 5.4.2.4. False -- 5.5. Translation ranking training and evaluation data -- 5.6. Linguistic resources -- 5.6.1. General language bilingual dictionary -- 5.6.2. Thesaurus -- 5.6.3. Bound morphemes translation table -- 5.6.4. Lexicon for word decomposition -- 5.6.5. Morphological families -- 5.6.6. Dictionary of cognates -- 5.7. Summary -- Chapter 6: Formalization and Evaluation of Candidate Translation Generation -- 6.1. Introduction -- 6.2. Translation generation algorithm -- 6.2.1. Decomposition -- 6.2.1.1. Morphological decomposition (SPLIT) -- 6.2.1.2. Morpheme concatenation (CONCATENATE) -- 6.2.2. Translation -- 6.2.3. Recomposition -- 6.2.3.1. Permutation of translated elements (PERMUTATE) -- 6.2.3.2. Concatenation into words (CONCATENATE) -- 6.2.3.3. Filtering (FILTER) -- 6.2.4. Selection -- 6.2.4.1. Definition of a candidate translation -- 6.3. Morphological splitting evaluation -- 6.4. Translation generation evaluation -- 6.4.1. Reference data and evaluation measures -- 6.4.1.1. A posteriori reference -- 6.4.1.1.1. Coverage (C) -- 6.4.1.1.2. Precision (P) -- 6.4.1.1.3. Usability (U) -- 6.4.1.1.4. Results obtained -- 6.4.1.2. A priori reference -- 6.4.1.2.1. Precision (P) -- 6.4.1.2.2. Recall (R) -- 6.4.1.2.3. F1-measure (F1) -- 6.4.1.2.4. Results obtained -- 6.4.2. Model genericity influence -- 6.4.2.1. A posteriori evaluation -- 6.4.2.2. A priori evaluation -- 6.4.2.3. Synthesis -- 6.4.3. Linguistic resources influence -- 6.4.3.1. A posteriori evaluation.
6.4.3.2. A priori evaluation -- 6.4.3.3. Synthesis -- 6.4.4. Fallback strategy influence -- 6.4.4.1. A posteriori evaluation -- 6.4.4.2. A priori evaluation -- 6.4.4.3. Synthesis -- 6.4.5. Fertile translations influence -- 6.4.5.1. A posteriori evaluation -- 6.4.5.2. A priori evaluation -- 6.4.5.3. Synthesis -- 6.4.6. Popular science corpus influence -- 6.4.6.1. Fertility and discourse type -- 6.4.7. Qualitative analysis -- 6.4.7.1. Silence analysis -- 6.4.7.2. Noise analysis -- 6.5. Discussion -- 6.5.1. Findings -- 6.5.1.1. A priori versus a posteriori reference -- 6.5.1.1.1. Comparing language pairs -- 6.5.1.1.2. Fertile translations -- 6.5.2. Research perspectives -- 6.5.2.1. Improving linguistic resources -- 6.5.2.2. Fertile translations -- Chapter 7: Formalization and Evaluation of Candidate Translation Ranking -- 7.1. Introduction -- 7.2. Ranking criteria -- 7.2.1. Context similarity -- 7.2.2. Candidate translation frequency -- 7.2.3. Parts-of-speech translation probability -- 7.2.4. Components translation mode -- 7.3. Criteria combination -- 7.3.1. Value standardization -- 7.3.2. Linear combination -- 7.3.3. Learning-to-rank model -- 7.4. Evaluation -- 7.4.1. Reference data and evaluation measures -- 7.4.2. Bases of comparison -- 7.4.3. Results -- 7.4.3.1. Ranking English ---> French translations -- 7.4.3.2. Ranking English ---> German translations -- 7.5. Discussion -- 7.5.1. Findings -- 7.5.1.1. Usefulness of criteria combination -- 7.5.1.2. Inconclusive results of the methods based on training -- 7.5.2. Research perspectives -- 7.5.2.1. Language and domain independent models -- 7.5.2.2. Better suited evaluation methods -- 7.5.2.3. Identification of correct translations -- 7.5.2.4. Combining different methods -- 7.5.2.5. Looking for new ranking features -- Conclusion and Perspectives -- PART 3: Appendices -- Appendix 1: Measures.
Appendix 2: Data.
Özet:
Computer-assisted translation (CAT) has always used translation memories, which require the translator to have a corpus of previous translations that the CAT software can use to generate bilingual lexicons. This can be problematic when the translator does not have such a corpus, for instance, when the text belongs to an emerging field. To solve this issue, CAT research has looked into the leveraging of comparable corpora, i.e. a set of texts, in two or more languages, which deal with the same topic but are not translations of one another. This work had two primary objectives. The first is to assess the input of lexicons extracted from comparable corpora in the context of a specialized human translation task. The second objective is to identify bilingual-lexicon-extraction methods which best match the translators' needs, determining the current limits of these techniques and suggesting improvements. The author focuses, in particular, on the identification of fertile translations, the management of multiple morphological structures, and the ranking of candidate translations. The experiments are carried out on two language pairs (English-French and English-German) and on specialized texts dealing with breast cancer. This research puts significant emphasis on applicability - methodological choices are guided by the needs of the final users. This book is organized in two parts: the first part presents the applicative and scientific context of the research, and the second part is given over to efforts to improve compositional translation. The research work presented in this book received the PhD Thesis award 2014 from the French association for natural language processing (ATALA).
Notlar:
Electronic reproduction. Ann Arbor, Michigan : ProQuest Ebook Central, 2017. Available via World Wide Web. Access may be limited to ProQuest Ebook Central affiliated libraries.
Tür:
Elektronik Erişim:
Click to View