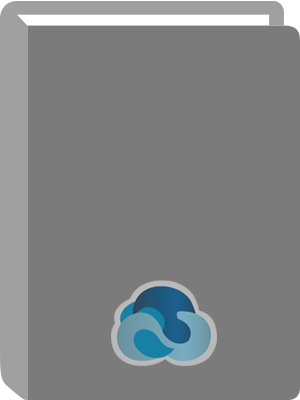
The Spectral Analysis of Time Series.
Başlık:
The Spectral Analysis of Time Series.
Yazar:
Koopmans, Lambert H.
ISBN:
9780080541563
Yazar Ek Girişi:
Fiziksel Tanımlama:
1 online resource (385 pages)
Seri:
Probability and Mathematical Statistics
İçerik:
Front Cover -- The Spectral Analysis of Time Series -- Copyright Page -- Contents -- Preface -- Acknowledgements -- Preface to the Second Edition -- Chapter 1. Preliminaries -- 1.1 Introduction -- 1.2 Time Series and Spectra -- 1.3 Summary of Vector Space Geometry -- 1.4 Some Probability Notations and Properties -- Chapter 2. Models for Spectral Analysis-The Univariate Case -- 2.1 Introduction -- 2.2 The Wiener Theory of Spectral Analysis -- 2.3 Stationary and Weakly Stationary Stochastic Processes -- 2.4 The Spectral Representation for Weakly Stationary Stochastic Processes-A Special Case -- 2.5 The General Spectral Representation for Weakly Stationary Processes -- 2.6 The Discrete and Continuous Components of the Process -- 2.7 Physical Realization of the Different Kinds of Spectra -- 2.8 The Real Spectral Representation -- 2.9 Ergodicity and the Connection between the Wiener and Stationary Process Theories -- 2.10 Statistical Estimation of the Autocovariance and the Mean Ergodic Theorem -- Appendix to Chapter 2 -- Chapter 3. Sampling, Aliasing, and Discrete-Time Models -- 3.1 Introduction -- 3.2 Sampling and the Aliasing Problem -- 3.3 The Spectral Model for Discrete-Time Series -- Chapter 4. Linear Filters-General Properties with Applications to Continuous-Time Processes -- 4.1 Introduction -- 4.2 Linear Filters -- 4.3 Combining Linear Filters -- 4.4 Inverting Linear Filters -- 4.5 Nonstationary Processes Generated by Time Varying Linear Filters -- Appendix to Chapter 4 -- Chapter 5. Multivariate Spectral Models and Their Applications -- 5.1 Introduction -- 5.2 The Spectrum of a Multivariate Time Series-Wiener Theory -- 5.3 Multivariate Weakly Stationary Stochastic Processes -- 5.4 Linear Filters for Multivariate Time Series -- 5.5 The Bivariate Spectral Parameters, Their Intepretations and Uses.
5.6 The Multivariate Spectral Parameters, Their Interpretations and Uses -- Appendix to Chapter 5 -- Chapter 6. Digital Filters -- 6.1 Introduction -- 6.2 General Properties of Digital Filters -- 6.3 The Effect of Finite Data Length -- 6.4 Digital Filters with Finitely Many Nonzero Weights -- 6.5 Digital Filters Obtained by Combining Simple Filters -- 6.6 Filters with Gapped Weights and Results Concerning the Filtering of Series with Polynomial Trends -- Appendix to Chapter 6 -- Chapter 7. Finite Parameter Models, Linear Prediction, and Real-Time Filtering -- 7.1 Introduction -- 7.2 Moving Averages -- 7.3 Autoregressive Processes -- 7.4 The Linear Prediction Problem -- 7.5 Mixed Autoregressive-Moving Average Processes and Recursive Prediction -- 7.6 Linear Filtering in Real Time -- Appendix to Chapter 7 -- Chapter 8. The Distribution Theory of Spectral Estimates with Applications to Statistical Inference -- 8.1 Introduction -- 8.2 Distribution of the Finite Fourier Transform and the Periodogram -- 8.3 Distribution Theory for Univariate Spectral Estimators -- 8.4 Distribution Theory for Multivariate Spectral Estimators with Applications to Statistical Inference -- Appendix to Chapter 8 -- Chapter 9. Sampling Properties of Spectral Estimates, Experimental Design, and Spectral Computations -- 9.1 Introduction -- 9.2 Properties of Spectral Estimators and the Selection of Spectral Windows -- 9.3 Experimental Design -- 9.4 Methods for Computing Spectral Estimators -- 9.5 Data Processing Problems and Techniques -- Appendix to Chapter 9 -- References -- Index -- Probability and Mathematical Statistics.
Özet:
To tailor time series models to a particular physical problem and to follow the working of various techniques for processing and analyzing data, one must understand the basic theory of spectral (frequency domain) analysis of time series. This classic book provides an introduction to the techniques and theories of spectral analysis of time series. In a discursive style, and with minimal dependence on mathematics, the book presents the geometric structure of spectral analysis. This approach makes possible useful, intuitive interpretations of important time series parameters and provides a unified framework for an otherwise scattered collection of seemingly isolated results. The books strength lies in its applicability to the needs of readers from many disciplines with varying backgrounds in mathematics. It provides a solid foundation in spectral analysis for fields that include statistics, signal process engineering, economics, geophysics, physics, and geology. Appendices provide details and proofs for those who are advanced in math. Theories are followed by examples and applications over a wide range of topics such as meteorology, seismology, and telecommunications. Topics covered include Hilbert spaces; univariate models for spectral analysis; multivariate spectral models; sampling, aliasing, and discrete-time models; real-time filtering; digital filters; linear filters; distribution theory; sampling properties ofspectral estimates; and linear prediction. Key Features * Hilbert spaces * univariate models for spectral analysis * multivariate spectral models * sampling, aliasing, and discrete-time models * real-time filtering * digital filters * linear filters * distribution theory * sampling properties of spectral estimates * linear prediction.
Notlar:
Electronic reproduction. Ann Arbor, Michigan : ProQuest Ebook Central, 2017. Available via World Wide Web. Access may be limited to ProQuest Ebook Central affiliated libraries.
Tür:
Elektronik Erişim:
Click to View